DomainAdaptor: A Novel Approach to Test-time Adaptation
arXiv (Cornell University)(2023)
摘要
To deal with the domain shift between training and test samples, current methods have primarily focused on learning generalizable features during training and ignore the specificity of unseen samples that are also critical during the test. In this paper, we investigate a more challenging task that aims to adapt a trained CNN model to unseen domains during the test. To maximumly mine the information in the test data, we propose a unified method called DomainAdaptor for the test-time adaptation, which consists of an AdaMixBN module and a Generalized Entropy Minimization (GEM) loss. Specifically, AdaMixBN addresses the domain shift by adaptively fusing training and test statistics in the normalization layer via a dynamic mixture coefficient and a statistic transformation operation. To further enhance the adaptation ability of AdaMixBN, we design a GEM loss that extends the Entropy Minimization loss to better exploit the information in the test data. Extensive experiments show that DomainAdaptor consistently outperforms the state-of-the-art methods on four benchmarks. Furthermore, our method brings more remarkable improvement against existing methods on the few-data unseen domain. The code is available at https://github.com/koncle/DomainAdaptor.
更多查看译文
关键词
adaptation,test-time
AI 理解论文
溯源树
样例
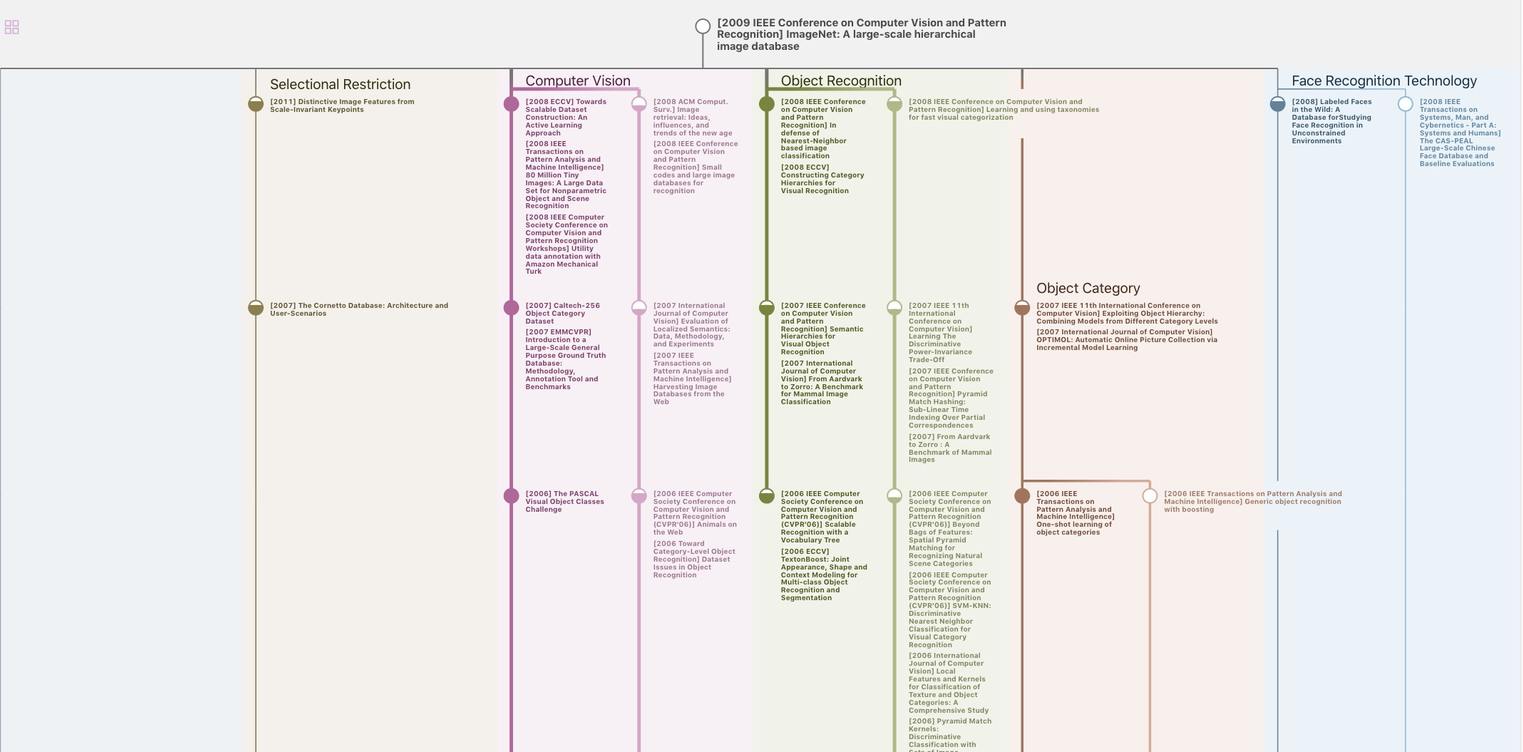
生成溯源树,研究论文发展脉络
Chat Paper
正在生成论文摘要