Explaining Anomalous Events in Flight Data of UAV With Deep Attention-Based Multi-Instance Learning
IEEE TRANSACTIONS ON VEHICULAR TECHNOLOGY(2024)
摘要
Ensuring the safety and reliability of unmanned aerial vehicles (UAVs) has become a critical issue as they continue to advance. Analyzing anomalous events using event-based explanations is an effective approach to identifying key anomalous behaviors and mitigating potential risks. However, this task is challenging because anomalous events in flight data lack time-step labels in real-world UAV flight scenarios. To address this challenge, we propose a dual attention-based multi-instance learning (DA-DI-MIL) for pinpointing anomaly instances and automatically explaining anomalous events. Our MIL-based framework, from a weakly supervised learning perspective, treats a segment of flight data as a 'bag' with an available label, and its time steps as 'instances' without labels. The dual attention mechanism in DA-DI-MIL combines a temporal pseudo-label, predicted by temporal attention, and sensor variable importance obtained from delta feature attention to better pinpoint anomalies from instance-level labels. We use these labels in our proposed MIL-based framework to establish the relationships between anomalous events and anomalous behaviors. We conducted extensive experiments on real UAV flight data with engine failures to demonstrate the effectiveness and robustness of our proposed method compared to existing state-of-the-art methods. DA-DI-MIL achieves near 90% in evaluation metrics with less than 5 time steps' delay of anomalous event detection and around 85% in temporal anomaly detection. Additionally, we present an illustration of both global and local interpretations in the time domain and feature space, providing comprehensive insights into anomalous events. Our proposed method has efficiently and automatically explained anomalous events in UAV flight data, contributing to high-level aviation safety and reliability.
更多查看译文
关键词
Sensors,Anomaly detection,Behavioral sciences,Autonomous aerial vehicles,Time series analysis,Data models,Training,anomalous events,attention mechanism,aviation safety,deep learning,multiple instance learning,unmanned aerial vehicle
AI 理解论文
溯源树
样例
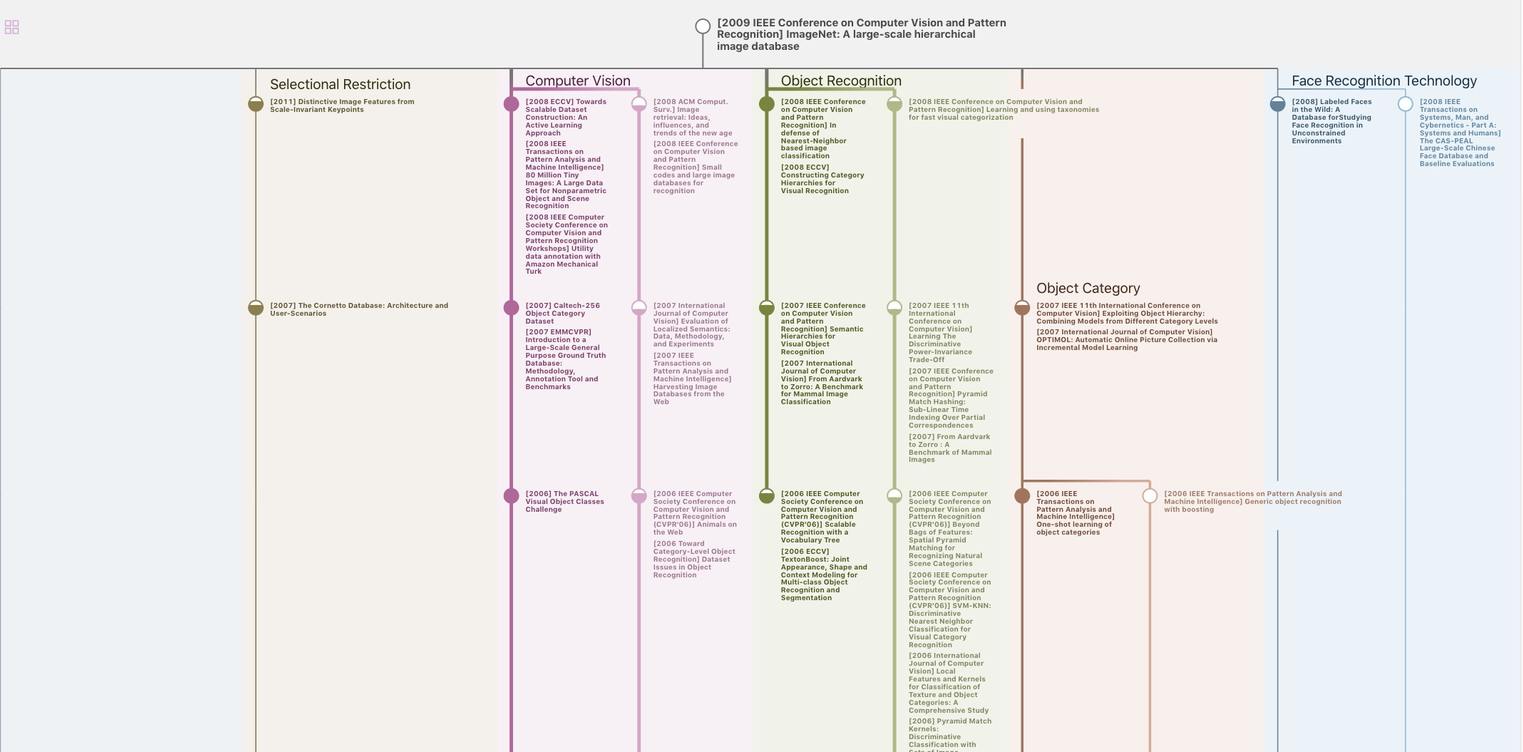
生成溯源树,研究论文发展脉络
Chat Paper
正在生成论文摘要