MUD-PQFed: Towards Malicious User Detection on model corruption in Privacy-preserving Quantized Federated learning
Computers & Security(2023)
摘要
The use of cryptographic privacy-preserving techniques in Federated Learning (FL) inadvertently induces a security dilemma because tampered local model parameters are encrypted and thus prevented from auditing. This work firstly demonstrates the triviality of performing model corruption attacks against privacy-preserving FL. We consider the scenario where the model updates are quantized to reduce the communication overhead, whilst the adversary can simply provide local parameters out of a legitimate range to corrupt the model. We then propose MUD-PQFed, a protocol that can precisely detect malicious attacks and enforce fair penalties on malicious clients. By deleting the contributions from the detected malicious clients, the global model utility is preserved as compared to the baseline global model in the absence of the corruption attack. Extensive experiments on MNIST, CIFAR-10, and CelebA benchmark datasets validate the efficacy in terms of retaining the baseline accuracy and effectiveness in terms of detecting malicious clients in a fine-grained manner.
更多查看译文
关键词
Federated learning,Privacy-preserving,Quantization,Model poisoning attack,Model corruption
AI 理解论文
溯源树
样例
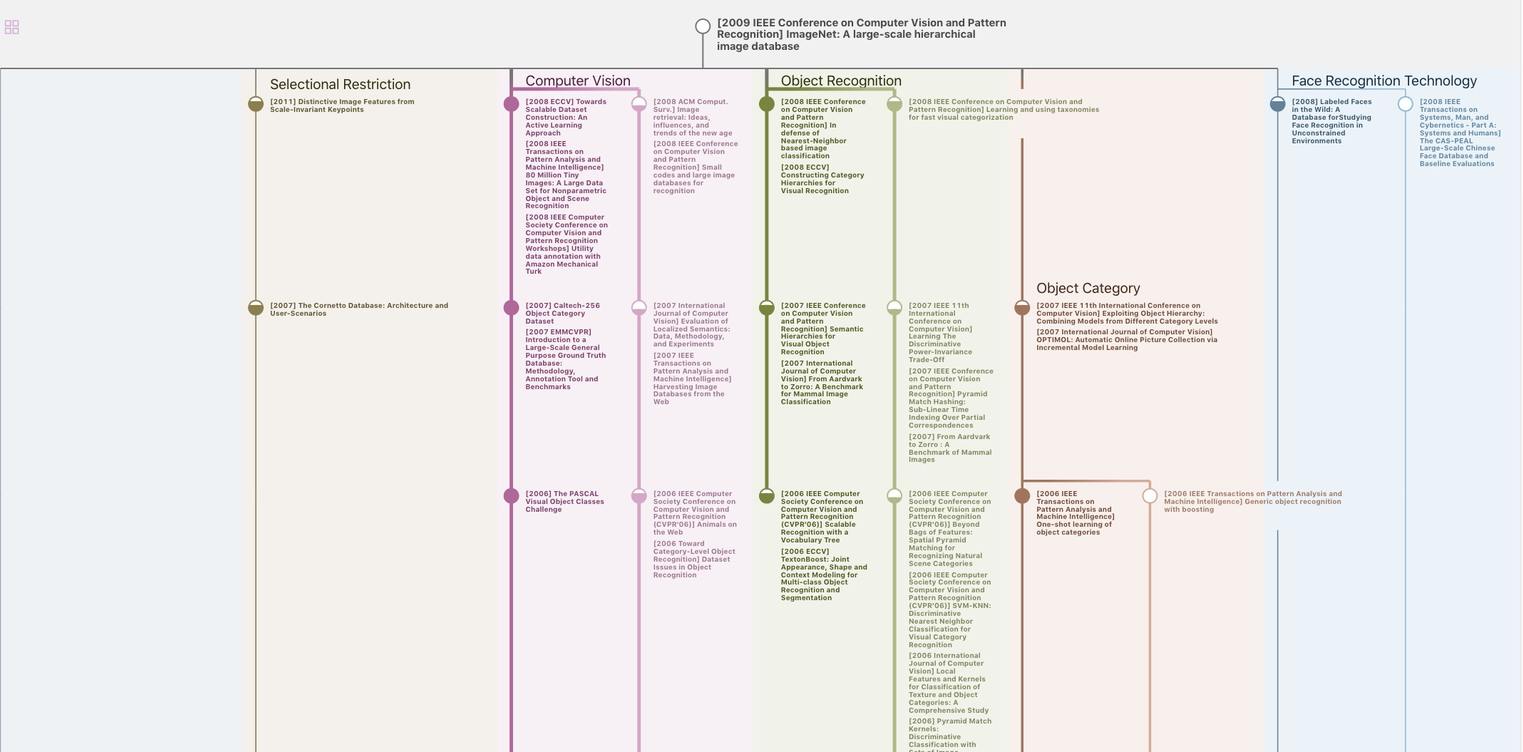
生成溯源树,研究论文发展脉络
Chat Paper
正在生成论文摘要