Dynamic Confidence Sampling and Label Semantic Guidance Learning for Domain Adaptive Retrieval
IEEE TRANSACTIONS ON MULTIMEDIA(2024)
摘要
To accurately retrieve similar objects from different domains, domain adaptive retrieval method is applied to cope with the domain shift problem in information retrieval. However, existing methods still have two problems: a) they fail to filter out low-confidence samples, leading to error accumulation; and b) they ignore the negative effect of domain discrepancy. To address these two issues, we propose an efficient method called Dynamic Confidence Sampling and Label Semantic Guidance Learning (DCS-LSG). First, Dynamic Confidence Sampling (DCS) is employed to dynamically select high-confidence samples from the target domain so as to improve the effectiveness of learning. Second, Label Semantic Guidance (LSG) learning is presented to enhance the label semantics of features during domain adaptive retrieval. In addition, we introduce a Dual-Projection Relaxation (DPR) strategy to learn more effective features on two specific projection spaces. At last, a two-step hashing strategy is used to generate high-quality hash codes. Experiments on multiple cross-domain retrieval datasets demonstrate that the proposed DCS-LSG can achieve a significant performance improvement.
更多查看译文
关键词
Semantics,Codes,Training,Navigation,Adaptive systems,Reliability,Headphones,Domain adaptive retrieval,dynamic confidence sampling,label semantic guidance learning,relaxation variables
AI 理解论文
溯源树
样例
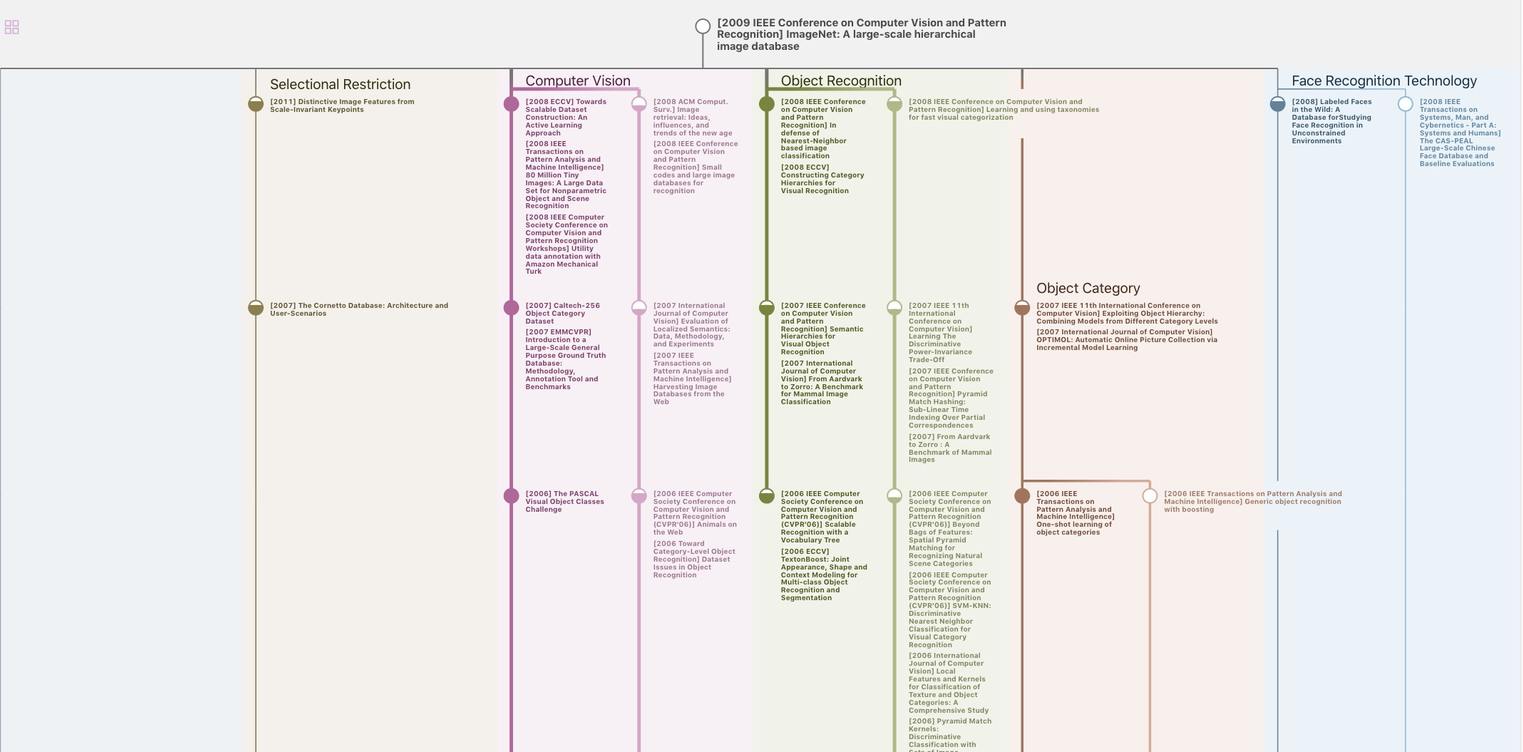
生成溯源树,研究论文发展脉络
Chat Paper
正在生成论文摘要