Exploring Sparse Visual Prompt for Domain Adaptive Dense Prediction
AAAI 2024(2024)
摘要
The visual prompts have provided an efficient manner in addressing visual cross-domain problems. Previous works introduce domain prompts to tackle the classification Test-Time Adaptation (TTA) problem by placing image-level prompts on the input and fine-tuning prompts for each target domain. However, since the image-level prompts mask out continuous spatial details in the prompt-allocated region, it will suffer from inaccurate contextual information and limited domain knowledge extraction, particularly when dealing with dense prediction TTA problems. To overcome these challenges, we propose a novel Sparse Visual Domain Prompts (SVDP) approach, which applies minimal trainable parameters (e.g., 0.1%) to pixels across the entire image and reserves more spatial information of the input. To better apply SVDP in extracting domain-specific knowledge, we introduce the Domain Prompt Placement (DPP) method to adaptively allocates trainable parameters of SVDP on the pixels with large distribution shifts. Furthermore, recognizing that each target domain sample exhibits a unique domain shift, we design Domain Prompt Updating (DPU) strategy to optimize prompt parameters differently for each sample, facilitating efficient adaptation to the target domain. Extensive experiments were conducted on widely-used TTA and continual TTA benchmarks, and our proposed method achieves state-of-the-art performance in both semantic segmentation and depth estimation tasks.
更多查看译文
关键词
ML: Transfer, Domain Adaptation, Multi-Task Learning,CV: Applications
AI 理解论文
溯源树
样例
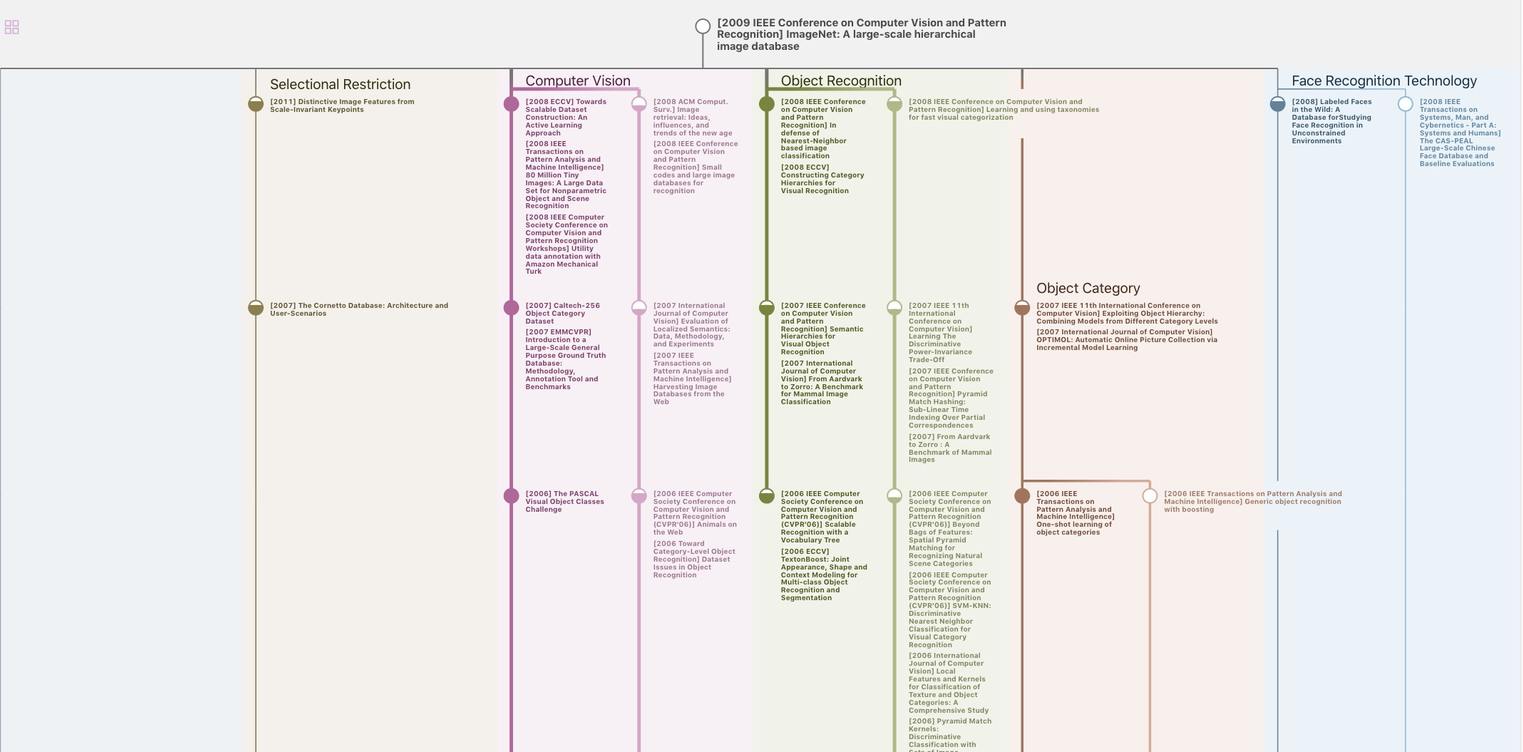
生成溯源树,研究论文发展脉络
Chat Paper
正在生成论文摘要