A Local-Global Feature Fusion Model For Aspect-Based Sentiment Classification
Research Square (Research Square)(2023)
摘要
Abstract The goal of aspect-based sentiment analysis is to predict the emotional polarity of a specific aspect within a sentence. However, while most current research in this area focuses on modeling context and extracting features, it often fails to consider the contextual information of aspect words, which can negatively impact the overall classification performance of the model. To address this issue, we propose a new aspect sentiment analysis model called LGFF-BERT, which is based on the fusion of local and global features using BERT. Our model includes a local feature extraction module and a global feature extraction module. We use two masking matrices to extract local contextual information between aspect words and context based on the semantic relevance distance (SRD), and extract global feature information through multi-head attention and point-wise convolution. Additionally, we incorporate an RFF residual module to reduce information loss during feature fusion. We evaluated our model using three common ABSA datasets: the SemEval-2014 laptop and restaurant datasets, as well as the ACL Twitter dataset. Our experimental results show that LGFF-BERT outperforms the baseline model significantly. Furthermore, through ablation experiments and parameter selection, we demonstrate the significance and effectiveness of our model design.
更多查看译文
关键词
classification,fusion,local-global,aspect-based
AI 理解论文
溯源树
样例
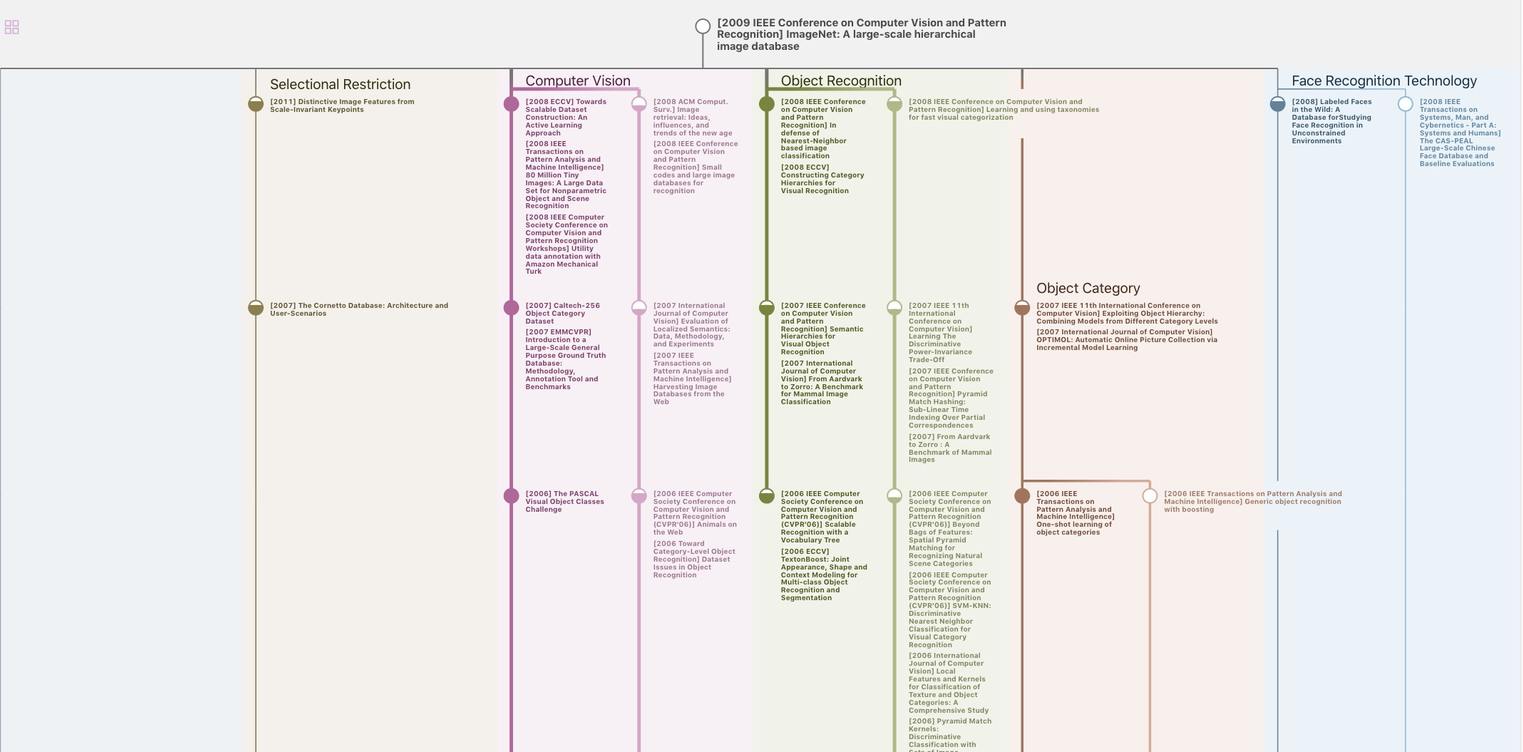
生成溯源树,研究论文发展脉络
Chat Paper
正在生成论文摘要