DAR-ILL: Double-attention Refining and Iterative Labeling Learning for Weakly Supervised Object Detection
IEEE Transactions on Cognitive and Developmental Systems(2023)
摘要
Weakly Supervised Object Detection (WSOD), which utilizes image-level annotated datasets to reduce labeling costs, has been gaining significant attention. Among the WSOD methods, multi-instance learning (MIL) has emerged as a popular solution. However, MIL suffers from reduced accuracy in both localization and classification, as it focuses solely on the most discriminative local regions. To address this limitation, we propose a novel end-to-end MIL-based WSOD method named DAR-ILL. Our approach leverages double-attention refining and iterative learning labeling to capture the region covering the entire object. The double-attention refining consists of two modules: the attention aggregation module (AAM) and the attention erasure module (AEM). AAM effectively combines spatial attention and channel attention to extract features with higher accuracy, while AEM selectively erases the most discriminative local region and subsequently expands the region to encompass the entire object. The iterative labeling learning procedure allows us to identify additional positive instances by introducing a threshold that identifies regions capable of covering the object. To further enhance supervisory information and optimize confidence maps, we introduce the dense optimization module, which facilitates more effective training of all branches. Extensive experimental evaluations demonstrate the superiority of DAR-ILL, as evidenced by its competitive performance in terms of mAP and CorLoc, with particular emphasis on CorLoc.
更多查看译文
关键词
Weakly supervised object detection,Attention erasure,Iterative label learning,Dense optimization
AI 理解论文
溯源树
样例
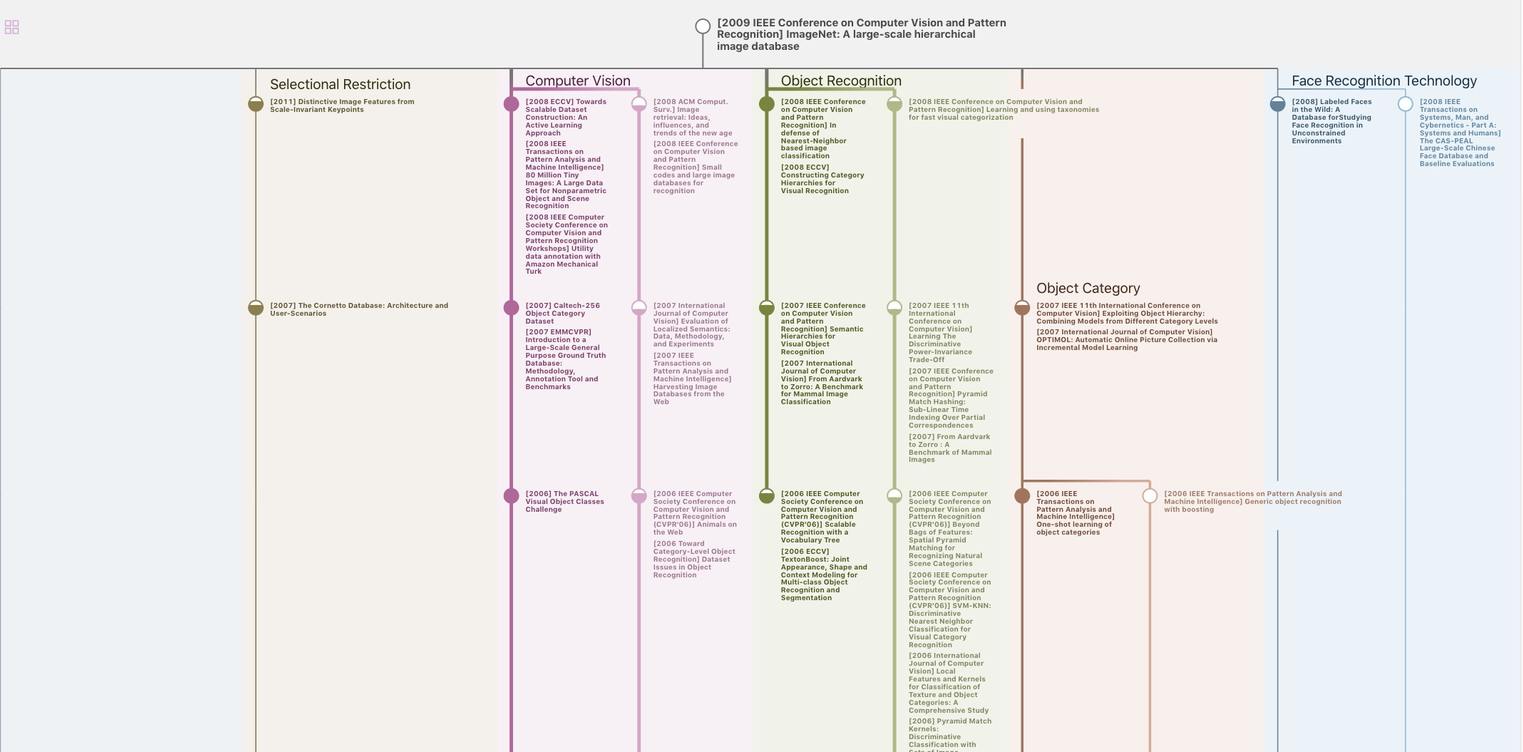
生成溯源树,研究论文发展脉络
Chat Paper
正在生成论文摘要