JLCSR: Joint Learning of Compactness and Separability Representations for Few-Shot Classification
IEEE TRANSACTIONS ON COGNITIVE AND DEVELOPMENTAL SYSTEMS(2024)
摘要
Few-shot classification (FSC) has aroused increasing attentions over years, which attempts to perform classification given a few labeled samples. In the context of transfer-learning for settling FSC, learning a general feature representation is very vital. For this purpose, our work focus on mining more information from the supervised data jointly provided by a certain amount of annotated samples and its corresponding self-supervised learning (SSL) task. To this end, we prove that the supervised losses of cross-entropy (CE) and supervised contrastive (SC) are, respectively, good at compactness and separability representations (SRs). On the basis of the above theory analysis, we further propose the joint learning of compactness and SRs (JLCSRs) for FSC. Specifically, for both original supervised data and its augmentation ones in the SSL task, it first, respectively, constructs CE loss and SC loss in the feature space. Then, joint learning is performed on the backbone network with the linear combination of above losses. The parameters of the backbone network are finally fixed to do the FSC evaluation. Extensive experiments on FSC benchmarks have demonstrated that the compactness and SRs learning can complement with each other and our method can reach comparable results with other state-of-the-art methods
更多查看译文
关键词
Few-shot classification (FSC),self-supervised learning (SSL),supervised learning (SL)
AI 理解论文
溯源树
样例
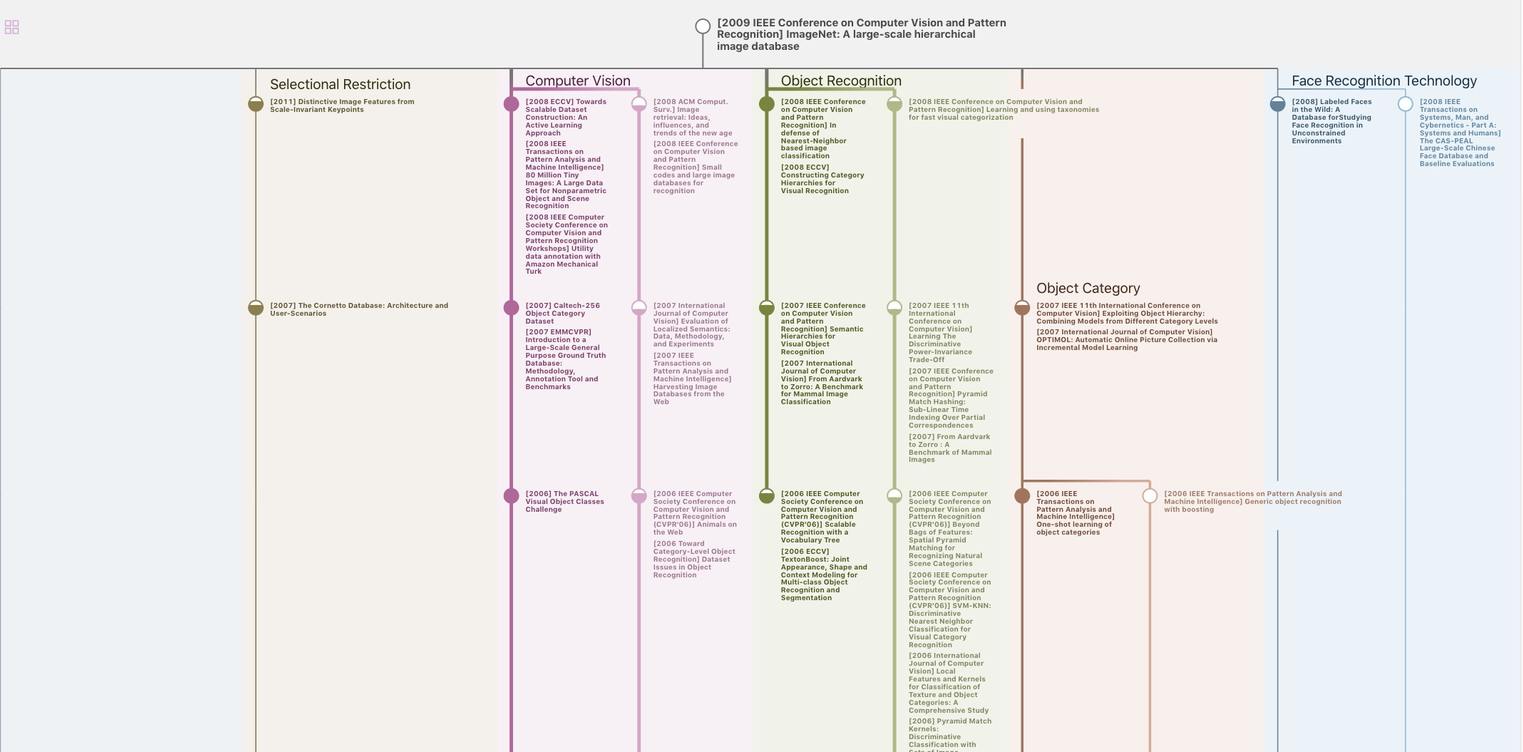
生成溯源树,研究论文发展脉络
Chat Paper
正在生成论文摘要