Dynamic Spatial Focus for Efficient Compressed Video Action Recognition
IEEE TRANSACTIONS ON CIRCUITS AND SYSTEMS FOR VIDEO TECHNOLOGY(2024)
摘要
Recent years have witnessed a growing interest in compressed video action recognition due to the rapid growth of online videos. It remarkably reduces the storage by replacing raw videos with sparsely sampled RGB frames and other compressed motion cues (motion vectors and residuals). However, existing compressed video action recognition methods face two main issues: First, the inefficiency caused by the usage of coarse-level information under full resolution, and second, the disturbing due to the noisy dynamics in motion vectors. To address the two issues, this paper proposes a dynamic spatial focus method for efficient compressed video action recognition (CoViFocus). Specifically, we first use a light-weighted two-stream architecture to localize the task-relevant patches for both the RGB frames and motion vectors. Then the selected patch pair will be processed by a high-capacity two-stream deep model for the final prediction. Such a patch selection strategy crops out the irrelevant motion noise in motion vectors, as well as reduces the spatial redundancy of the inputs, leading to the high efficiency of our method in the compressed domain. Moreover, we found that the motion vectors can help our method to address the possibly happened static-issue, which means that the focus patches get stuck at some regions related to static objects rather than target actions, which further improves our method. Extensive results on both the HMDB-51 and UCF-101 datasets demonstrate the effectiveness and efficiency of our method in compressed video action recognition tasks.
更多查看译文
关键词
Video action recognition,compressed video,efficient video analysis,dynamic neural networks
AI 理解论文
溯源树
样例
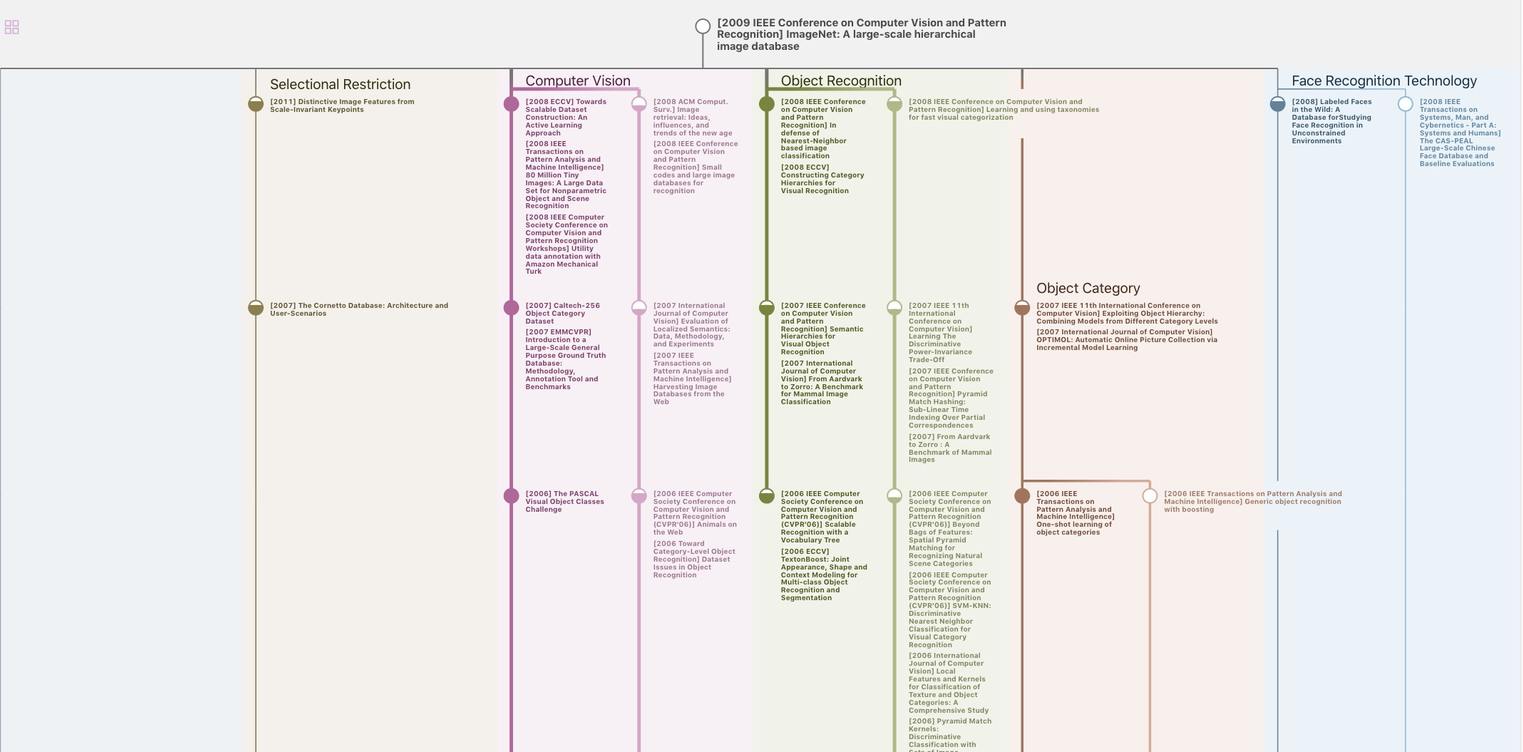
生成溯源树,研究论文发展脉络
Chat Paper
正在生成论文摘要