Massive Data-Centric Parallelism in the Chiplet Era
arXiv (Cornell University)(2023)
摘要
Recent works have introduced task-based parallelization schemes to accelerate graph search and sparse data-structure traversal, where some solutions scale up to thousands of processing units (PUs) on a single chip. However parallelizing these memory-intensive workloads across millions of cores requires a scalable communication scheme as well as designing a cost-efficient computing node that makes multi-node systems practical, which have not been addressed in previous research. To address these challenges, we propose a task-oriented scalable chiplet architecture for distributed execution (Tascade), a multi-node system design that we evaluate with up to 256 distributed chips -- over a million PUs. We introduce an execution model that scales to this level via proxy regions and selective cascading, which reduce overall communication and improve load balancing. In addition, package-time reconfiguration of our chiplet-based design enables creating chip products that optimized post-silicon for different target metrics, such as time-to-solution, energy, or cost. We evaluate six applications and four datasets, with several configurations and memory technologies to provide a detailed analysis of the performance, power, and cost of data-centric execution at a massive scale. Our parallelization of Breadth-First-Search with RMAT-26 across a million PUs -- the largest of the literature -- reaches 3021 GTEPS.
更多查看译文
关键词
data-centric
AI 理解论文
溯源树
样例
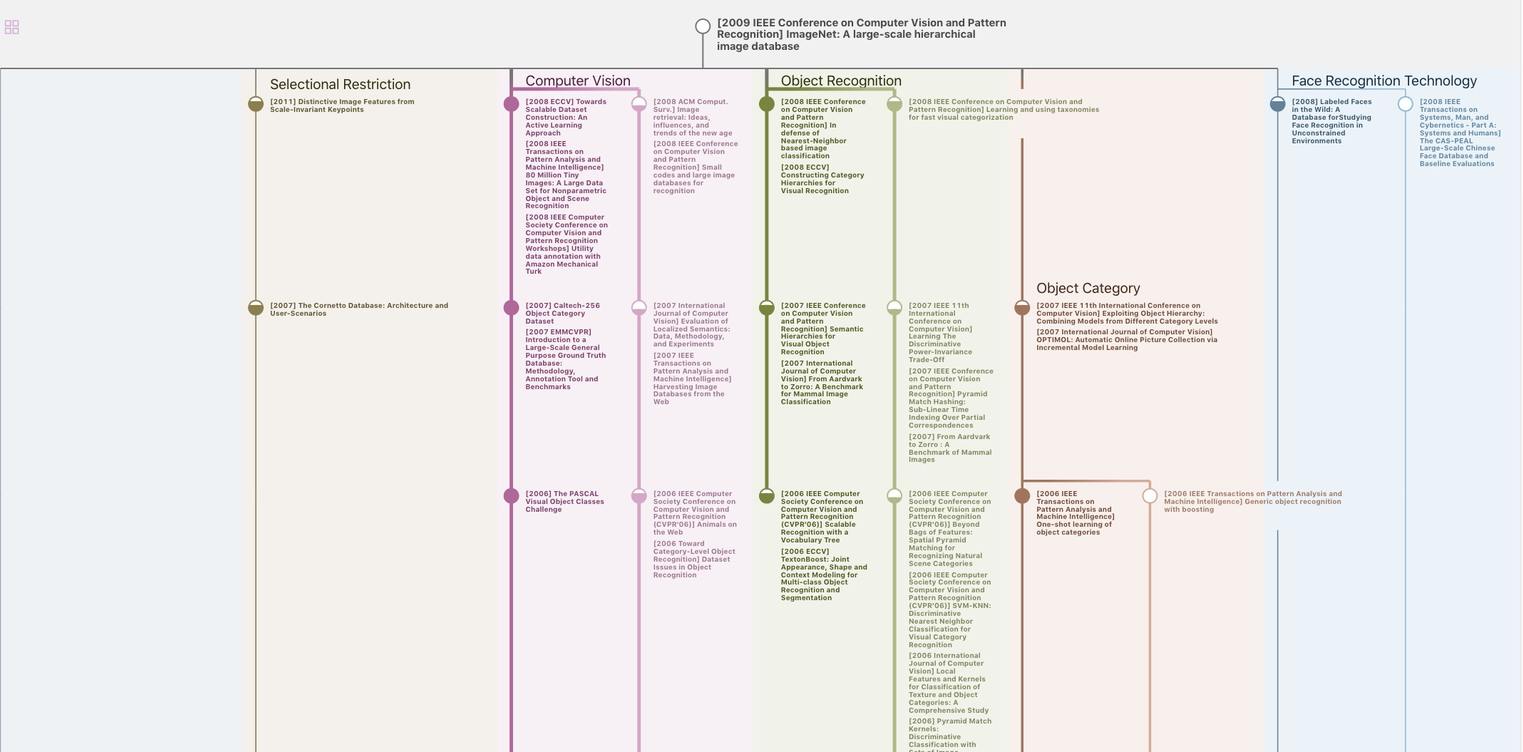
生成溯源树,研究论文发展脉络
Chat Paper
正在生成论文摘要