Multi-task neural network blind deconvolution and its application to bearing fault feature extraction
Measurement Science and Technology(2023)
摘要
Abstract Blind deconvolution (BD) is one of the effective methods that extract fault-related characteristics in vibration signals. Currently, most BD methods specify an optimization criterion and use frequency or time domain signal independently to optimize a deconvolution filter. However, they prone to overfitting due to the various noises. The time-domain-based BD methods tend to extract fault-unrelated single peak impulse, and the frequency-domain-based BD methods tend to retain the maximum energy frequency component but lose harmonics frequency components. To solve the above issue, we propose a hybrid criterion that combines the kurtosis in time domain and the G-l1/l2 norm in the frequency domain. Two criteria have opposite monotonicity that mutually constrain to avoid overfitting. Moreover, we construct a multi-task 1D convolutional neural network with two branches to achieve an optimal solution. This network realizes the simultaneous optimization of two domains. Experimental results show that our proposed method outperforms other state-of-the-art BD methods.
更多查看译文
关键词
blind deconvolution,fault,neural network,multi-task
AI 理解论文
溯源树
样例
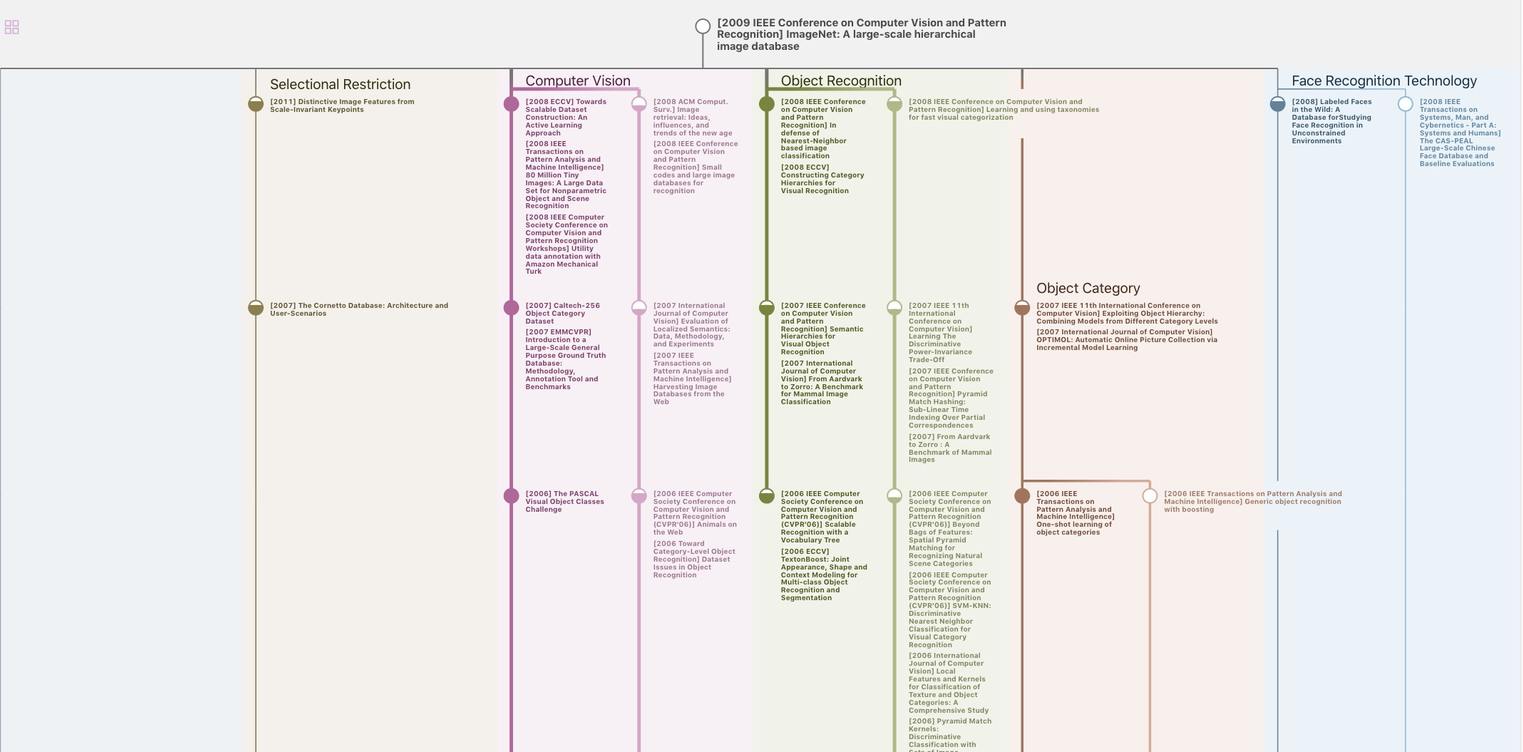
生成溯源树,研究论文发展脉络
Chat Paper
正在生成论文摘要