Relational Fisher Analysis: Dimensionality Reduction in Relational Data with Global Convergence
ALGORITHMS(2023)
摘要
Most of the dimensionality reduction algorithms assume that data are independent and identically distributed (i.i.d.). In real-world applications, however, sometimes there exist relationships between data. Some relational learning methods have been proposed, but those with discriminative relationship analysis are lacking yet, as important supervisory information is usually ignored. In this paper, we propose a novel and general framework, called relational Fisher analysis (RFA), which successfully integrates relational information into the dimensionality reduction model. For nonlinear data representation learning, we adopt the kernel trick to RFA and propose the kernelized RFA (KRFA). In addition, the convergence of the RFA optimization algorithm is proved theoretically. By leveraging suitable strategies to construct the relational matrix, we conduct extensive experiments to demonstrate the superiority of our RFA and KRFA methods over related approaches.
更多查看译文
关键词
relational learning,dimensionality reduction,graph embedding,trace ratio,document understanding and recognition,face recognition
AI 理解论文
溯源树
样例
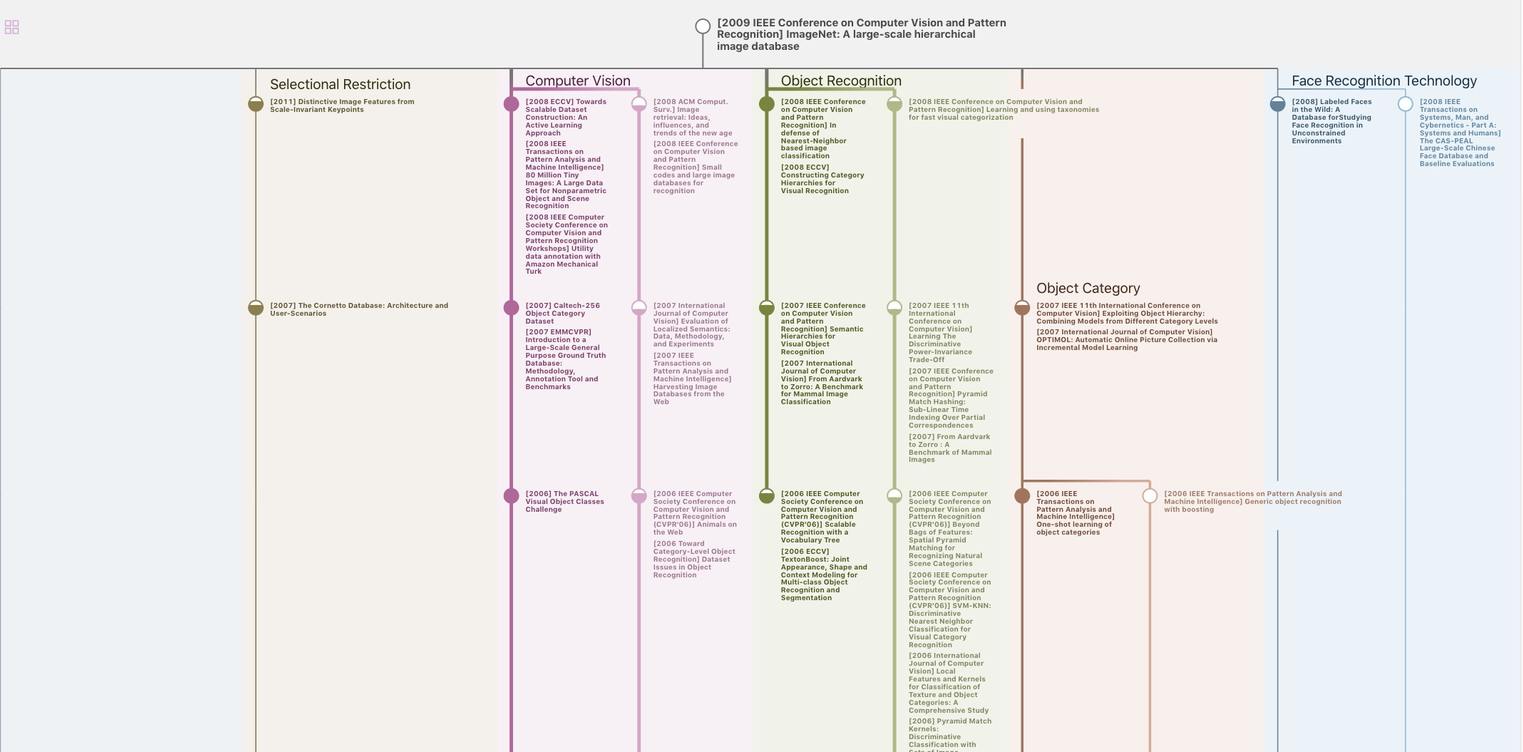
生成溯源树,研究论文发展脉络
Chat Paper
正在生成论文摘要