REDFormer: Radar Enlightens the Darkness of Camera Perception With Transformers.
IEEE Trans. Intell. Veh.(2024)
摘要
Enhancing the accuracy and reliability of perception systems in automated vehicles is critical, especially under varying driving conditions. Unfortunately, the challenges of adverse weather and low-visibility conditions can seriously degrade camera performance, introducing significant risks to vehicle safety. To address these concerns, in this study, we introduce a novel transformer-based 3D object detection model named ‘REDFormer’. By exploiting bird's-eye-view camera-radar fusion, the REDFormer offers a more practical and financially viable solution for tackling low-visibility conditions. We validate our model using the comprehensive nuScenes dataset, incorporating camera images, multi-radar point clouds, weather information, and time-of-day data. In comparative analyses, our model surpasses state-of-the-art benchmarks in both classification and detection accuracy. An in-depth ablation study further elucidates the individual contributions of each model component in overcoming the challenges posed by weather and lighting conditions. Experimental results specifically highlight the model's significant performance improvements, demonstrating a 31.31% increase in accuracy under rainy conditions and a 46.99% enhancement during nighttime scenarios, affirming REDFormer's potential as a robust and cost-effective solution for automated vehicles.
更多查看译文
关键词
Sensor fusion,automated vehicle,computer vision,perception,machine learning
AI 理解论文
溯源树
样例
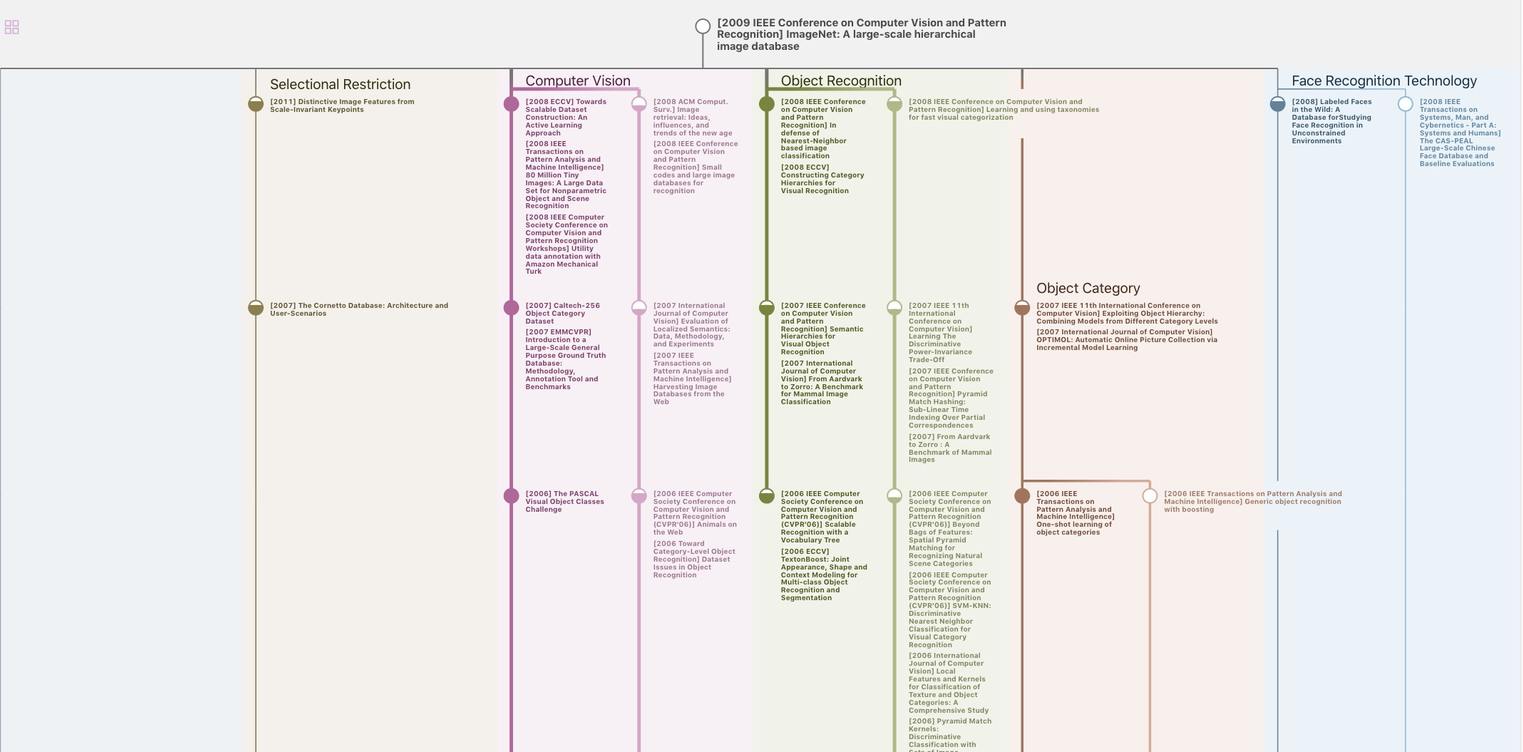
生成溯源树,研究论文发展脉络
Chat Paper
正在生成论文摘要