Feature Entropy Adaptive Network for Weak Magnetic Signal Classification
IEEE SENSORS JOURNAL(2024)
摘要
Magnetic anomaly signals are composed of anomaly signal and the geomagnetic field. Due to the similarity in magnitude between these two types of signals and the difficulty in acquiring magnetic field data, distinguishing between them is challenging, and the available dataset is small. This article aims to address the classification of weak magnetic signals with limited samples obtained from actual measurements, and a novel neural network-based approach for magnetic anomaly classification is proposed. First, the feature selection is performed on the fused magnetic field signal features. The measured magnetic signals are decomposed using the standard orthogonal basis functions (OBFs), and the coefficients of the basis functions are utilized as magnetic moment features. The wavelet transform is employed to calculate the coefficients as the time-frequency features of the magnetic field data. Statistical features are extracted based on the characteristics of the magnetic anomaly data. Using the statistical feature mean as a benchmark, selection is conducted considering the characteristics of the feature dataset, resulting in improved classification results. Afterward, a lightweight magnetic anomaly classification model, MAD_FA, was designed, resulting in an average reduction of 41.67% in training time. Focal loss was employed as the loss function during training, leading to an improvement of 3.86% in classification accuracy. A multifeature adaptive entropy weighting (MFAEW) method is proposed to extract magnetic signal features, which adaptively determines feature weights and effectively utilizes the mutual information and complementarity between features. This approach accelerates network convergence and improves the classification accuracy by 2.53%. Finally, a comparison was made between the MAD_FA model and classical signal classification models, and a series of ablation experiments were conducted to evaluate the model. The suggested technique performs well in the task of classifying weak magnetic signals, with a classification accuracy of 99.96%, an F1 score of 96.38%, and an AUC score of 99.12%. The higher classification accuracy and stronger robustness compared to the traditional methods demonstrate the potential application of the magnetic anomaly classification model with feature adaptation (MAC_FA) model in weak magnetic signal classification tasks.
更多查看译文
关键词
Magnetic anomaly classification model with feature adaptation (MAC_FA) network,multifeature adaptive entropy weighting (MFAEW),orthogonal basis function (OBF),weak magnetic classification
AI 理解论文
溯源树
样例
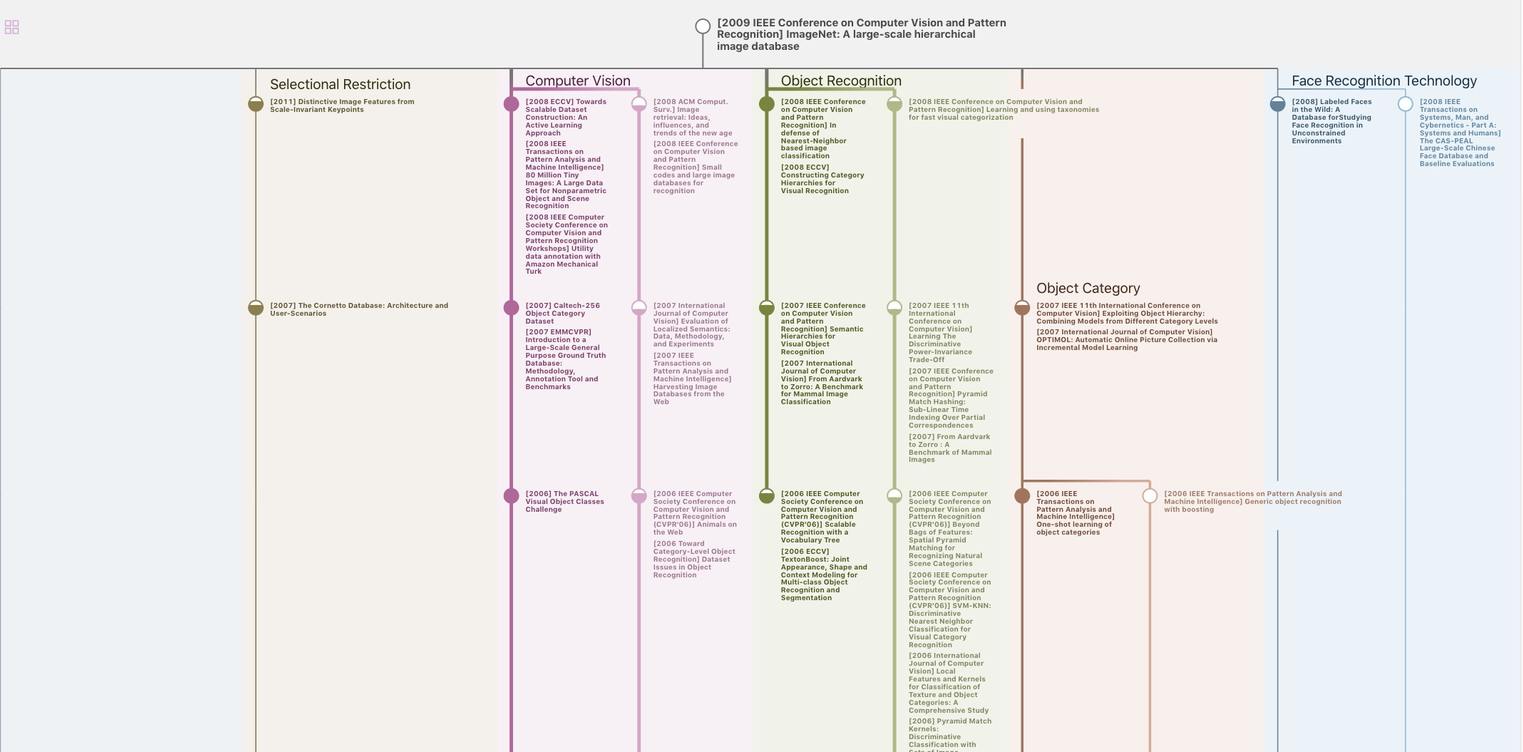
生成溯源树,研究论文发展脉络
Chat Paper
正在生成论文摘要