Global-and-Local Attention-Based Reinforcement Learning for Cooperative Behaviour Control of Multiple UAVs
IEEE TRANSACTIONS ON VEHICULAR TECHNOLOGY(2024)
摘要
Due to the strong adaptability and high flexibility, unmanned aerial vehicles (UAVs) have been extensively studied and widely applied in both civil and military applications. Although UAVs can achieve significant cost reduction and performance enhancement in large-scale systems by taking full advantage of their cooperation and coordination, they result in a serious cooperative behaviour control problem. Especially in dynamic environments, the cooperative behaviour control problem which has to quickly produce a safe and effective behaviour decision for each UAV to achieve group missions, is NP-hard and difficult to settle. In this work, we design a global-and-local attention-based reinforcement learning algorithm for the cooperative behaviour control problem of UAVs. First, with the motion and coordination models, we analyze the collision avoidance, motion state update, and task execution constraints of multiple UAVs, and abstract the cooperative behaviour control problem as a multi-constraint decision-making one. Then, inspired from the human-learning process where more attention is devoted to the important parts of data, we design a multi-agent reinforcement learning algorithm with a global-and-local attention mechanism to cooperatively control the behaviours of UAVs and achieve the coordination. Simulation experiments in a multi-agent particle environment provided by OpenAI are conducted to verify the effectiveness and efficiency of the proposed approach. Compared with baselines, our approach shows significant advantages in mean reward, training time, and coordination effect.
更多查看译文
关键词
Global-and-local attention mechanism,reinforcement learning,cooperative behaviour control,multiple UAVs,multi-constraint decision-making
AI 理解论文
溯源树
样例
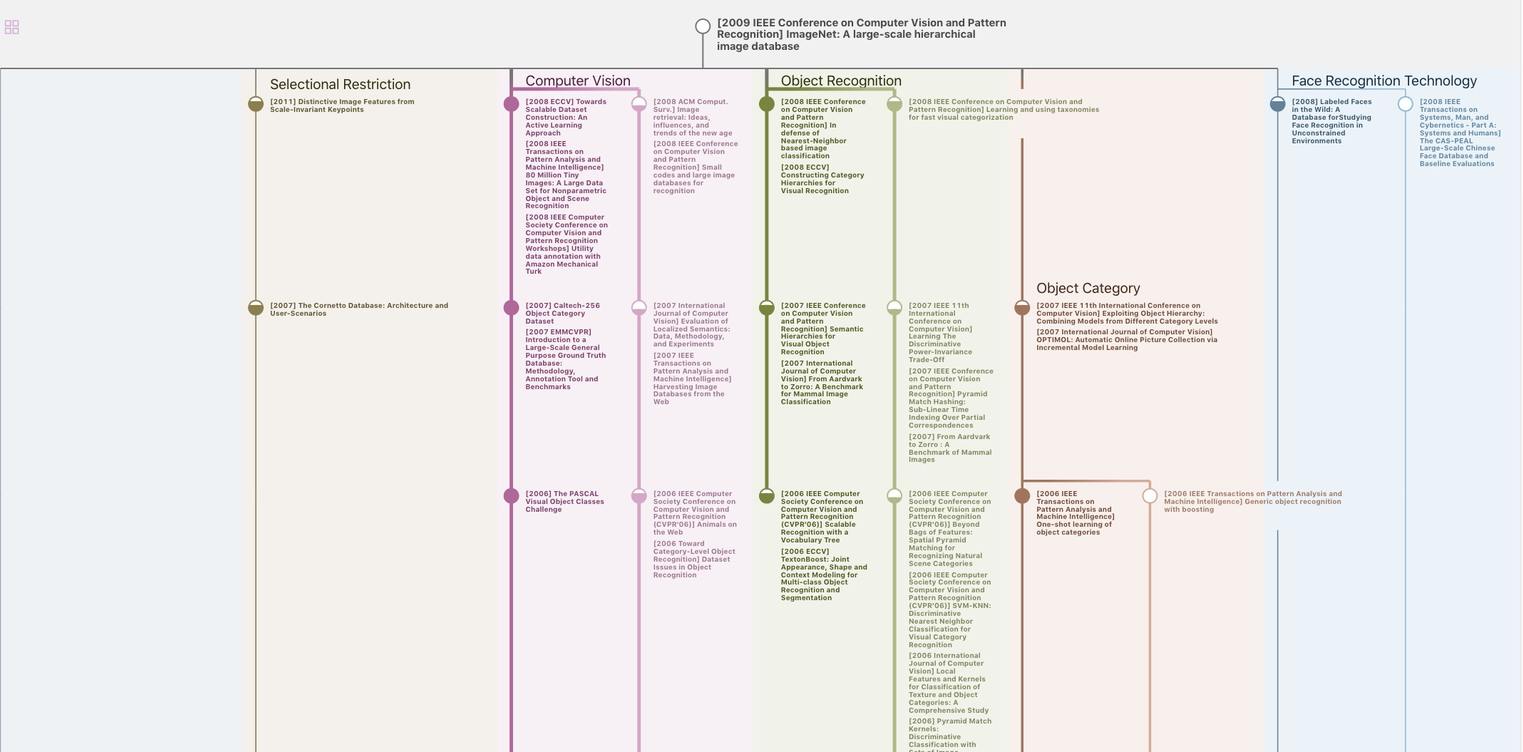
生成溯源树,研究论文发展脉络
Chat Paper
正在生成论文摘要