i-Razor: A Differentiable Neural Input Razor for Feature Selection and Dimension Search in DNN-Based Recommender Systems
IEEE Transactions on Knowledge and Data Engineering(2023)
摘要
Input features play a crucial role in DNN-based recommender systems with thousands of categorical and continuous fields from users, items, contexts, and interactions. Noisy features and inappropriate embedding dimension assignments can deteriorate the performance of recommender systems and introduce unnecessary complexity in model training and online serving. Optimizing the input configuration of DNN models, including feature selection and embedding dimension assignment, has become one of the essential topics in feature engineering. However, in existing industrial practices, feature selection and dimension search are optimized sequentially, i.e., feature selection is performed first, followed by dimension search to determine the optimal dimension size for each selected feature. Such a sequential optimization mechanism increases training costs and risks generating suboptimal input configurations. To address this problem, we propose a differentiable neural
i
nput
razor
(
i-Razor
) that enables joint optimization of feature selection and dimension search. Concretely, we introduce an end-to-end differentiable model to learn the relative importance of different embedding regions of each feature. Furthermore, a flexible pruning algorithm is proposed to achieve feature filtering and dimension derivation simultaneously. Extensive experiments on two large-scale public datasets in the Click-Through-Rate (CTR) prediction task demonstrate the efficacy and superiority of i-Razor in balancing model complexity and performance.
更多查看译文
关键词
AutoML,recommender systems,deep learning,feature selection,dimension search
AI 理解论文
溯源树
样例
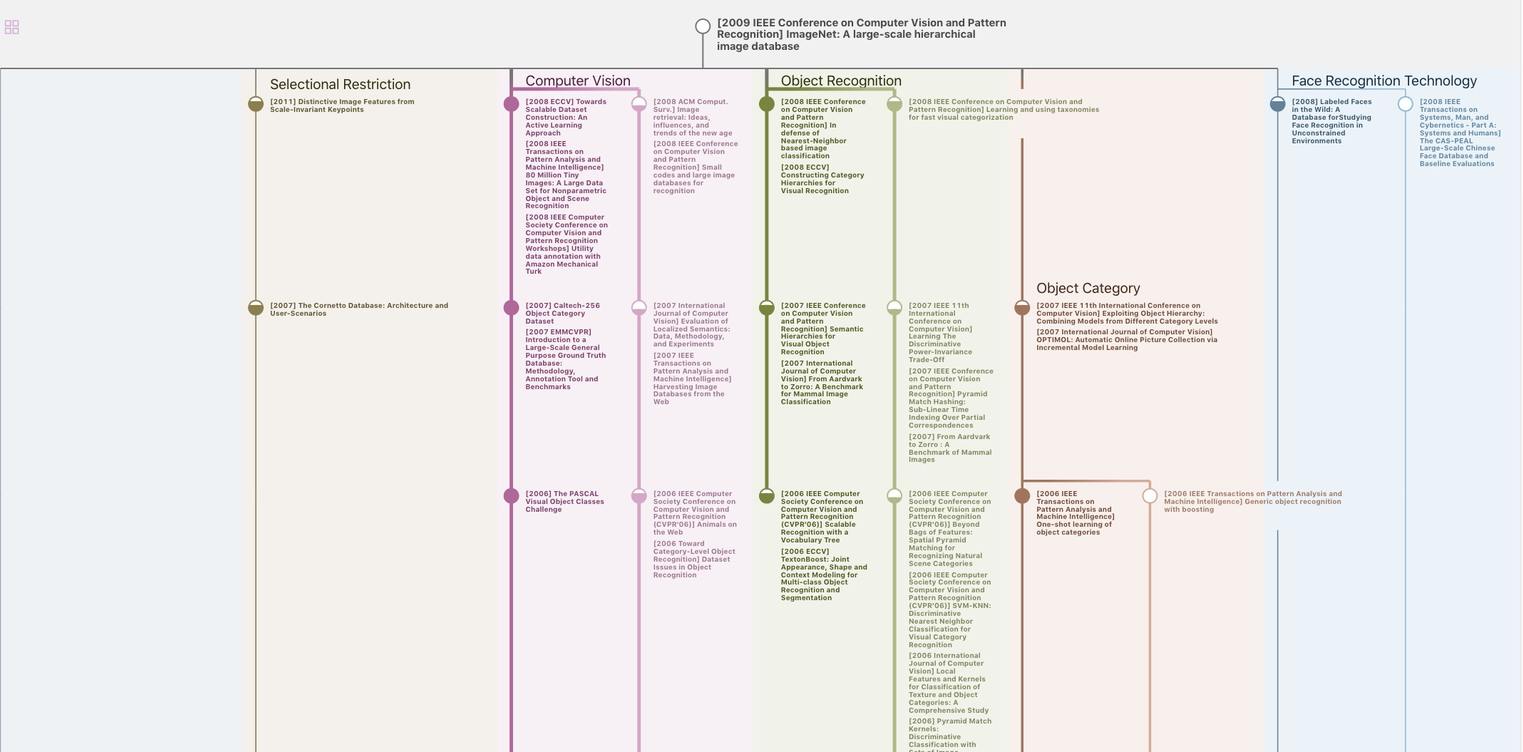
生成溯源树,研究论文发展脉络
Chat Paper
正在生成论文摘要