On-Device Indoor Positioning: A Federated Reinforcement Learning Approach With Heterogeneous Devices.
IEEE Internet Things J.(2024)
摘要
The widespread deployment of machine learning techniques in ubiquitous computing environments has sparked interests in exploiting the vast amount of data stored on mobile devices. To preserve data privacy, federated learning has been proposed to learn a shared model by performing distributed training locally on participating devices and aggregating the local models into a global one. Reinforcement learning (RL) can improve indoor localization by accounting for environmental dynamics, but has been trained on centralized data. A federated learning version of RL can help train a global localization model using data from different user clients whereas keeping data on device without centralization. We propose a personalized federated RL for indoor localization that addresses two major challenges. Due to the limited network connectivity of mobile devices, under the federated computing setting, it is impractical to aggregate updates from all clients in any learning iteration. Data gathered on different devices are heterogeneous, imposing difficulty in training high accuracy models. In our approach, each client performs RL to learn an action policy that can quickly search for a target based on its own data (e.g., personalized) and then a central server communicates with clients only for their model updates and learns a global model that is in the proximity of all client models (e.g., federated). Empirical evaluations demonstrate superior performance of the proposed approach in terms of localization accuracy and steadiness over existing methods. We further extend our approach to few-shot learning that can quickly position a new user with sparse annotated location data.
更多查看译文
关键词
Indoor Localization,Privacy Preserving,Device Heterogeneity,Federated Learning,Deep Reinforcement Learning,Few-Shot Learning
AI 理解论文
溯源树
样例
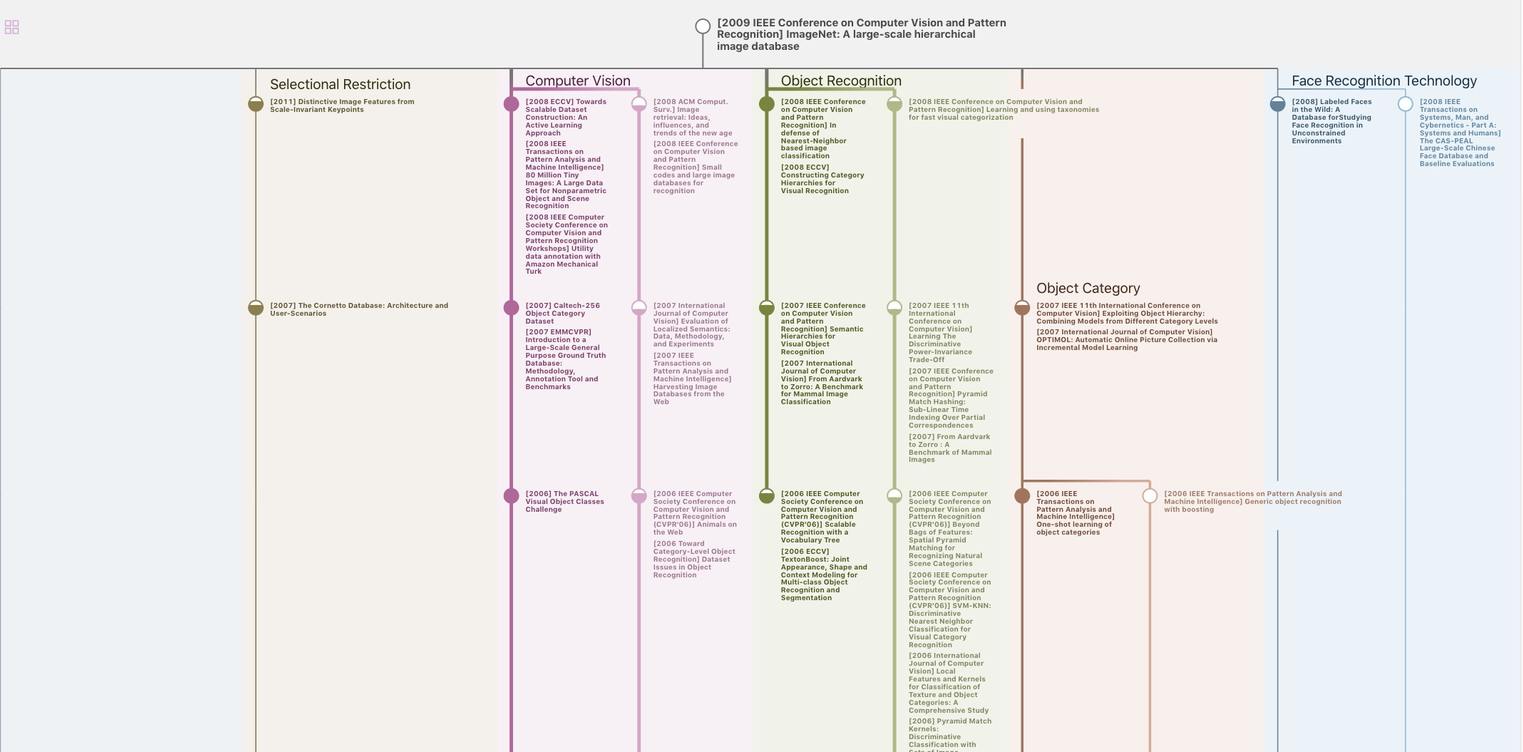
生成溯源树,研究论文发展脉络
Chat Paper
正在生成论文摘要