Mixture-of-Linear-Experts for Long-term Time Series Forecasting
CoRR(2023)
摘要
Long-term time series forecasting (LTSF) aims to predict future values of a
time series given the past values. The current state-of-the-art (SOTA) on this
problem is attained in some cases by linear-centric models, which primarily
feature a linear mapping layer. However, due to their inherent simplicity, they
are not able to adapt their prediction rules to periodic changes in time series
patterns. To address this challenge, we propose a Mixture-of-Experts-style
augmentation for linear-centric models and propose Mixture-of-Linear-Experts
(MoLE). Instead of training a single model, MoLE trains multiple linear-centric
models (i.e., experts) and a router model that weighs and mixes their outputs.
While the entire framework is trained end-to-end, each expert learns to
specialize in a specific temporal pattern, and the router model learns to
compose the experts adaptively. Experiments show that MoLE reduces forecasting
error of linear-centric models, including DLinear, RLinear, and RMLP, in over
78% of the datasets and settings we evaluated. By using MoLE existing
linear-centric models can achieve SOTA LTSF results in 68% of the experiments
that PatchTST reports and we compare to, whereas existing single-head
linear-centric models achieve SOTA results in only 25% of cases. Additionally,
MoLE models achieve SOTA in all settings for the newly released Weather2K
datasets.
更多查看译文
AI 理解论文
溯源树
样例
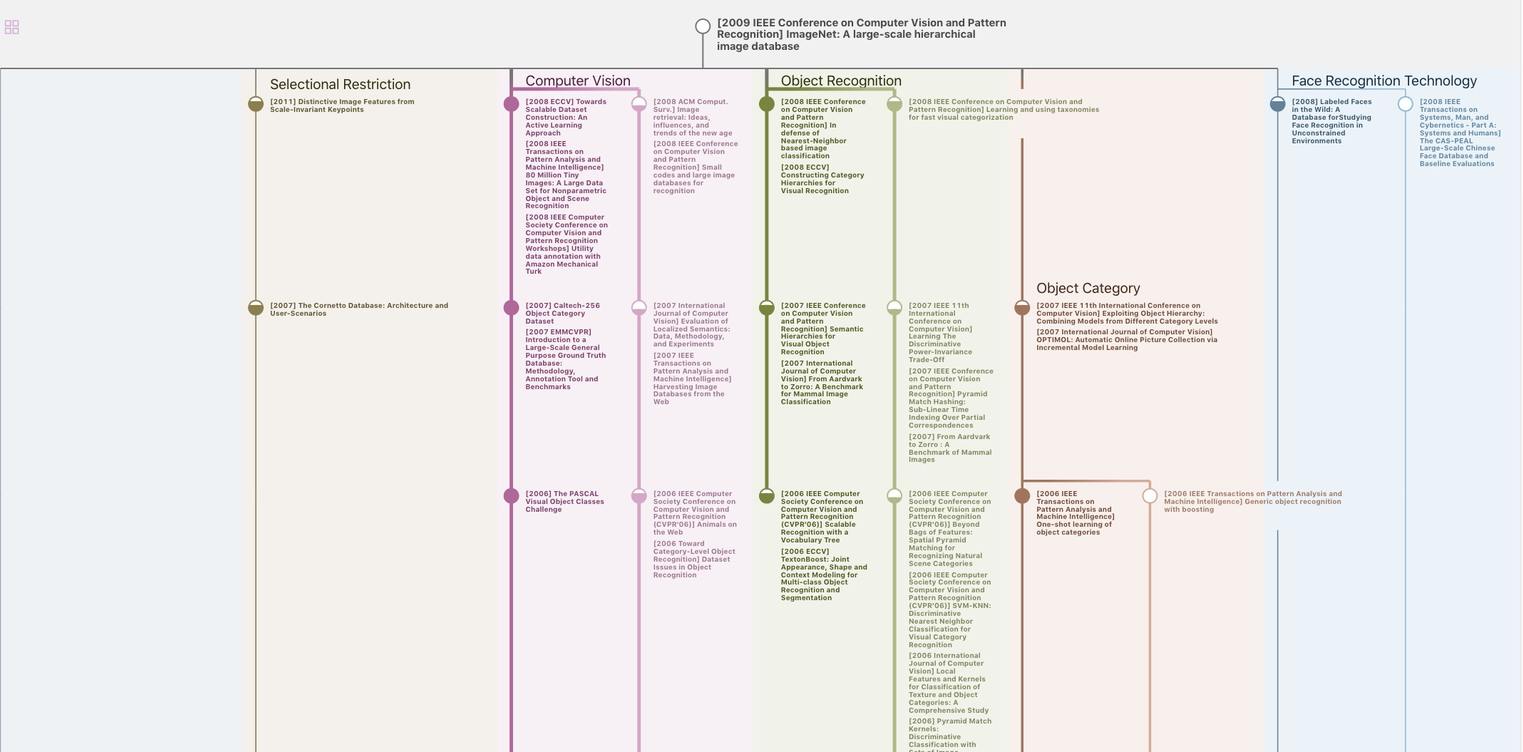
生成溯源树,研究论文发展脉络
Chat Paper
正在生成论文摘要