How to Tame Mobility in Federated Learning Over Mobile Networks?
IEEE TRANSACTIONS ON WIRELESS COMMUNICATIONS(2023)
摘要
Federated learning (FL) over mobile networks has attracted intensive attention recently. User mobility is a fundamental feature of mobile networks, which leads to dynamic network topology and wireless connectivity losses. As such, user mobility is usually considered a "trouble maker" and a great challenge to FL over mobile networks. Interestingly, we found that small user mobility can positively contribute to improving FL performance. This is because the total dataset size and the data diversity that the FL can utilize are increased by user mobility. Based on this observation, we aim to tame and exploit mobility instead of treating it as a hostile "trouble maker". To this end, we first investigate how the FL performance changes with user mobility theoretically by jointly taking into account the positive and negative aspects of mobility. Specifically, a closed-form expression to quantify the impact of mobility on the FL loss is derived, which explains when negative or positive aspects of mobility dominate the FL performance. Next, a joint FL and communication optimization problem is formulated based on theoretical analyses to minimize the FL loss function by optimizing wireless resource allocation. Finally, we propose a two-step optimization algorithm to solve the formulated problem. The simulation results verify the theoretical analyses. It is also shown that the proposed method can significantly enhance learning performance considering users with high mobility. When the average velocity is larger than 150 km/h, the proposed method achieves more than 80% accuracy in the MNIST dataset, while the existing methods may fail during training.
更多查看译文
关键词
Federated learning,user mobility,resource allocation,convergence analysis
AI 理解论文
溯源树
样例
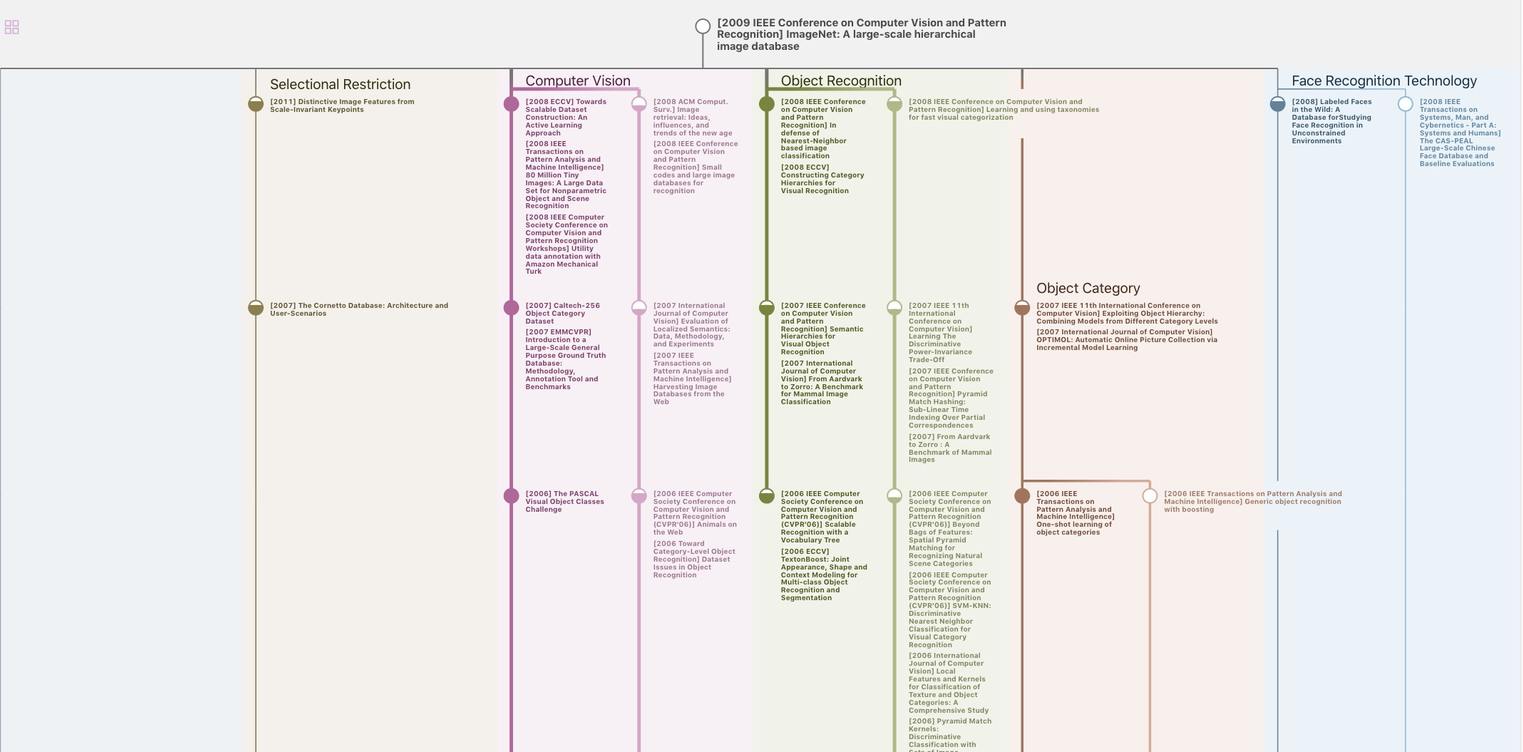
生成溯源树,研究论文发展脉络
Chat Paper
正在生成论文摘要