Masked Siamese Prompt Tuning for Few-Shot Natural Language Understanding.
IEEE Trans. Artif. Intell.(2024)
摘要
Recently, prompt-based learning has shown excellent performance on few-shot scenarios. Using frozen language models to tune trainable continuous prompt embeddings has become a popular and powerful methodology. For few-shot natural language understanding, even if we freeze the parameters of the pre-trained language model, the learned pseudo-prompts might still be overfitted. In this paper, we propose a novel masked siamese prompt tuning (MSP-tuning) to improve few-shot natural language understanding. Concretely, MSP-tuning masks randomly out part of the prompt tokens to get a pair of masked siamese prompts for each sample. Each training sample is then fed to the model twice with the masked siamese prompts. Finally, MSP-tuning minimizes the JS-divergence between the two output probability distributions of the pre-trained language model to regularize the model further. Experiment results on the few-shot GLUE benchmark and SuperGLUE benchmark show that MSP-tuning outperforms previous approaches. Numerically, our MSP-tuning achieves an average improvement of 1.79% (BERT-base) and 1.39% (BERT-large) of the GLUE benchmark and 1.90% (RoBERTa-base) and 1.71% (RoBERTa-large) of the SuperGLUE benchmark compared to state-of-the-art method P-tuning. Our method facilitates applying large pre-trained language models in natural language understanding.
更多查看译文
关键词
Few-shot,masked language model,natural language understanding,prompt learning
AI 理解论文
溯源树
样例
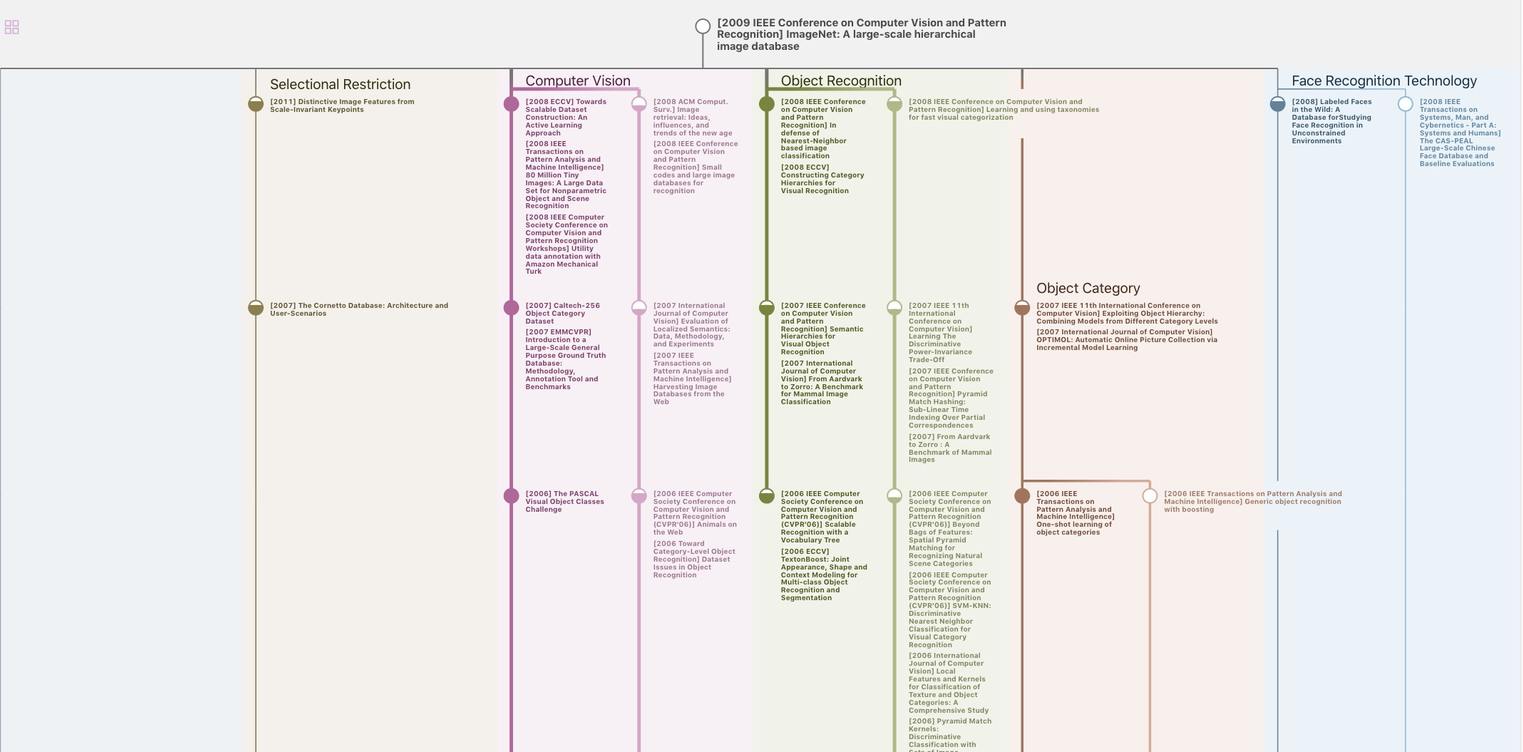
生成溯源树,研究论文发展脉络
Chat Paper
正在生成论文摘要