Motion Complement and Temporal Multifocusing for Skeleton-Based Action Recognition
IEEE TRANSACTIONS ON CIRCUITS AND SYSTEMS FOR VIDEO TECHNOLOGY(2024)
摘要
Modeling sequences with spatial-temporal graph convolutional networks has become a mainstream paradigm in skeleton-based action recognition. However, many existing methods adopt redundant or cluttered structures to mine the key action features, thus making it difficult to achieve a balanced or leading performance in accuracy and efficiency. In this paper, we propose a novel framework, referred to as Motion Complement and Temporal Multifocusing Network (MCTM-Net), to capture the relationships within skeleton sequences by means of an efficient decomposition of the spatiotemporal graph model. Specifically, for spatial modeling, we introduce a motion-related relational descriptor that extends the channel dimension so as to enhance the modeling of motion salient regions as a complement to the conventional physical adjacency relationships. An improved parameterized physical relationship model is also proposed to better fit the data characteristics. As for temporal modeling, we propose an efficient multi-focus temporal information acquisition strategy that aggregates the information from multiple temporal spans and adjacent regions. We conduct extensive experiments on multiple representative datasets, including NTU-RGB+D (60&120), Northwestern-UCLA, and UWA3D Multiview Activity II, to validate our innovations. The experimental results show the effectiveness of our method.
更多查看译文
关键词
Graph convolutional network,skeletion-based action recognition
AI 理解论文
溯源树
样例
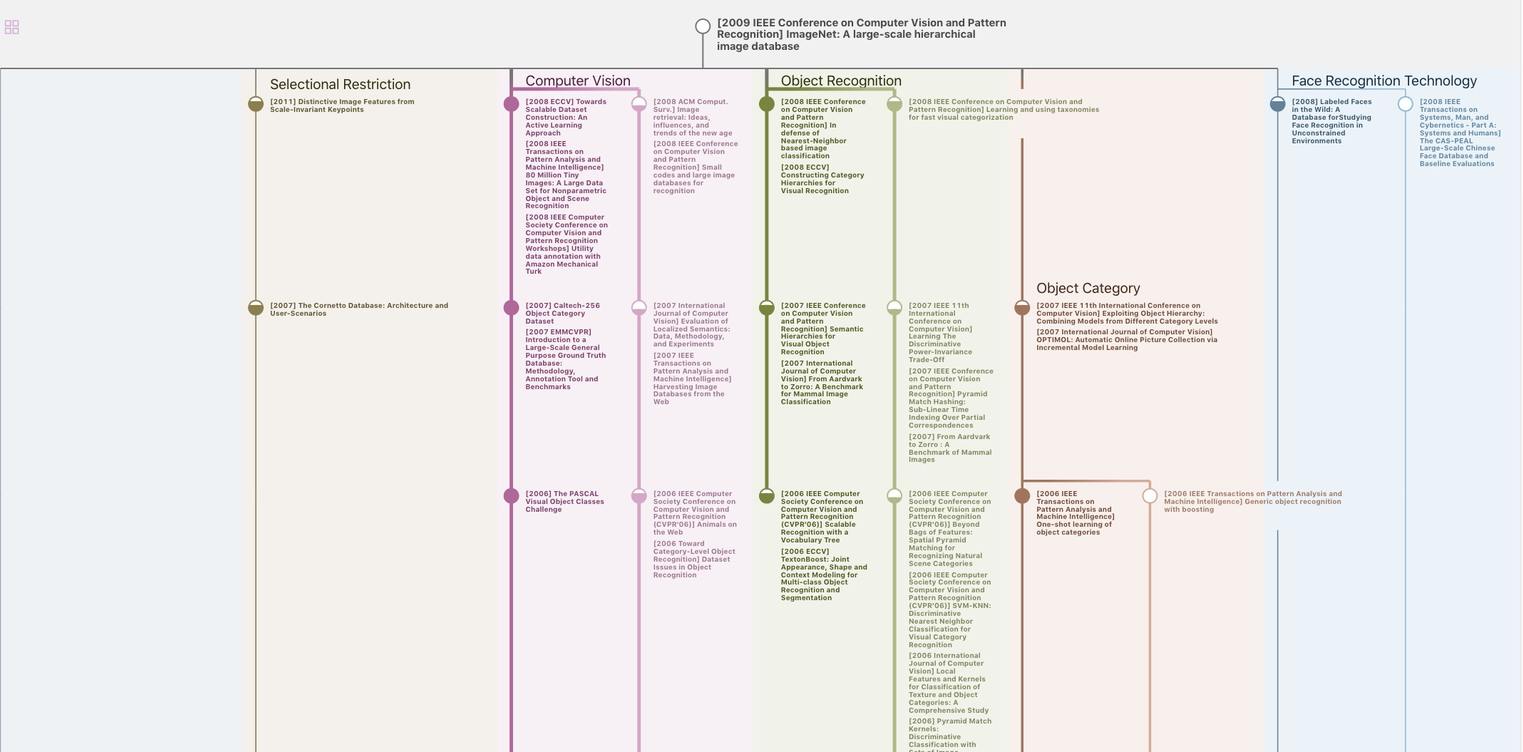
生成溯源树,研究论文发展脉络
Chat Paper
正在生成论文摘要