Feature Weakening, Contextualization, and Discrimination for Weakly Supervised Temporal Action Localization
IEEE TRANSACTIONS ON MULTIMEDIA(2024)
摘要
Weakly-supervised Temporal Action Localization (W-TAL) aims to train a model to localize all action instances potentially from different classes in an untrimmed video, using a training dataset that has video-level action class labels but has no detailed annotations on the start and end timestamps of action instances. We propose to solve the W-TAL problem from the feature learning aspect, with a new architecture, termed F3-Net, which includes (1) a Feature Weakening (FW) module that can identify and randomly weaken either the most discriminative action or the most discriminative background features over the training iterations to force the network to precisely localize the action instances in both discriminative and ambiguous action-related frames, without spreading to the background intervals; (2) a Feature Contextualization (FC) module that can infer the global contexts among video segments and attentionally fuse them with the local contexts from individual video segments to generate more representative features; and (3) a Feature Discrimination (FD) module that can highlight the most discriminative video segments/classes corresponding to each class/segment, respectively, for localizing multiple action instances from different classes within a video. Experimental results on THUMOS14 and ActivityNet1.3 demonstrate the state-of-the-art performance of our F3-Net, and the FW and FC are also effective plug-in modules to improve other methods. This project will be available at https://moniruzzamanmd.github.io/F3-Net/https://moniruzzamanmd.github.io/F3-Net/
更多查看译文
关键词
Temporal action localization,Feature weakening,Feature contextualization,Feature discrimination
AI 理解论文
溯源树
样例
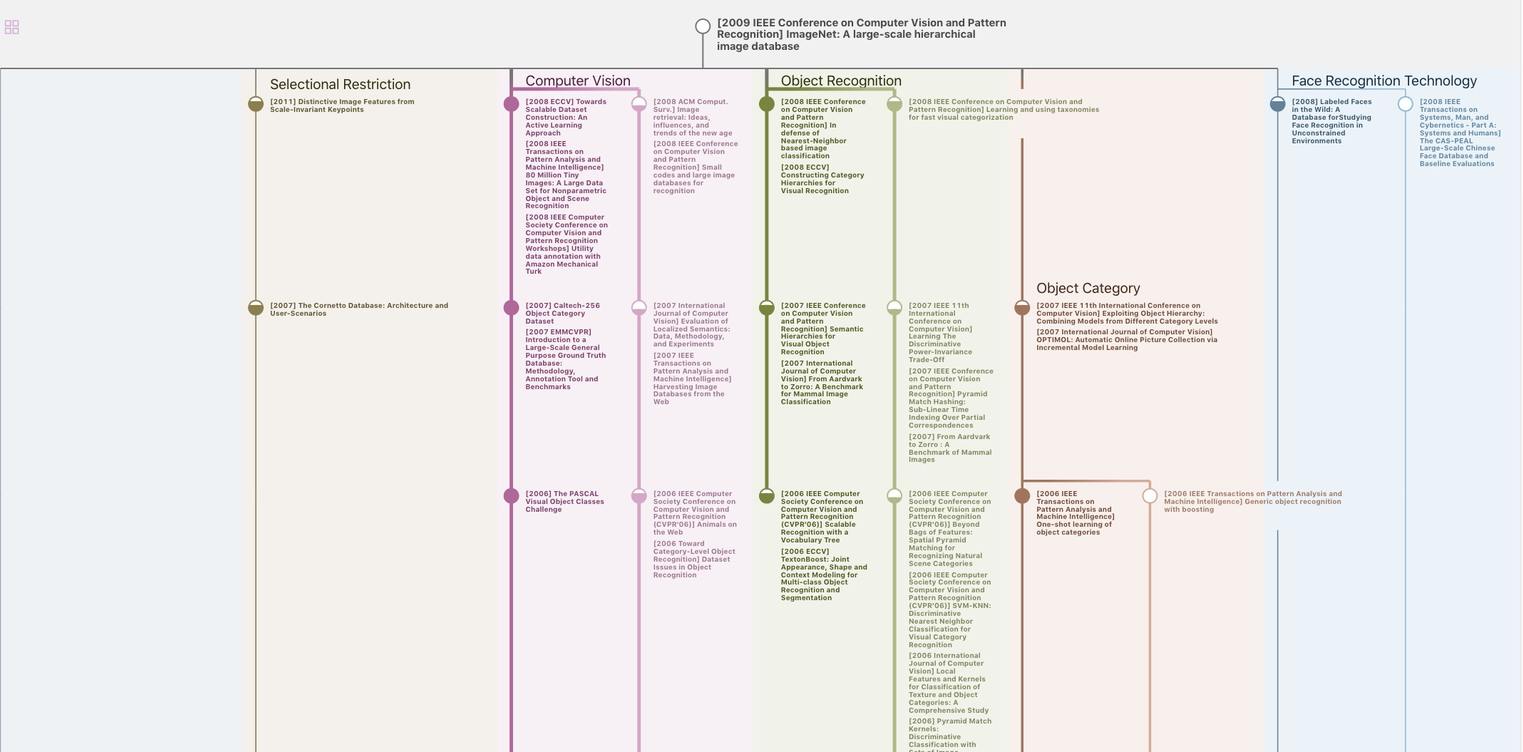
生成溯源树,研究论文发展脉络
Chat Paper
正在生成论文摘要