Unsupervised Abnormal Transaction Order Detection Method Based on Deep Learning Time Factor
Journal of physics(2023)
摘要
Abstract Abnormal transaction order detection plays an important role in identifying transaction data changes and abnormal transaction behaviors of users. The existing abnormal order detection methods have low generalization ability in different transaction time series, and the exception itself has a variety of patterns. In addition, the positive sample points with a large proportion carry much more information than the abnormal sample points, which will cause the classifier to be disturbed in the learning process and inaccurate classification. The research proposes an unsupervised abnormal transaction order detection method based on the depth learning time factor. By processing the original data column, the first feature sequence is obtained, and then the later feature sequence is trained through the depth learning model. The abnormal order is detected by comparing the differences between the two feature sequences. The results show that the research effectively solves the practical problem of too few abnormal transaction samples, can be applied to the transaction process of different modes, reduces the noise in the original transaction data, and has obvious improvement in each evaluation index compared with the traditional method.
更多查看译文
关键词
deep learning time factor,deep learning,order
AI 理解论文
溯源树
样例
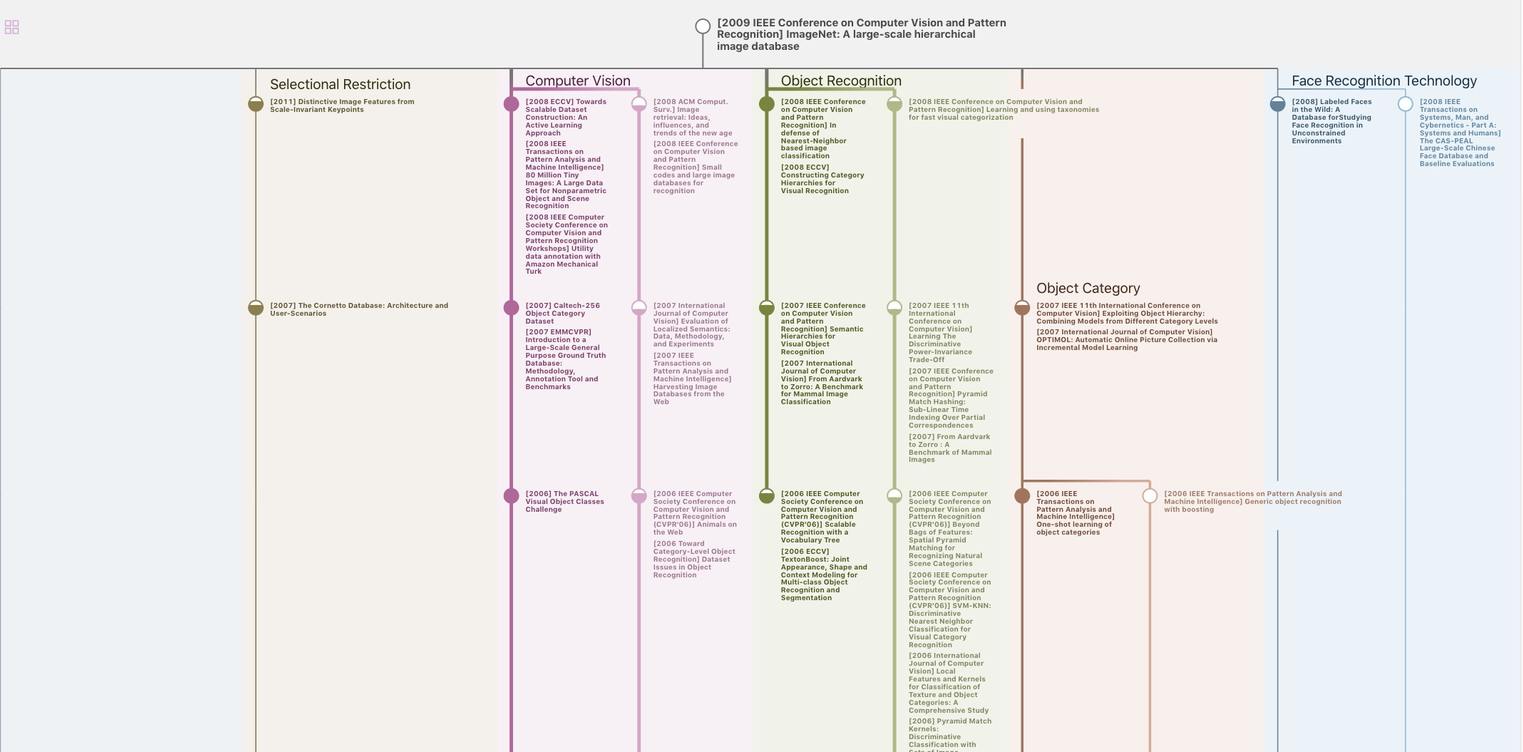
生成溯源树,研究论文发展脉络
Chat Paper
正在生成论文摘要