Model compression method combining multi-factor channel pruning and knowledge distillation
Third International Conference on Computer Vision and Data Mining (ICCVDM 2022)(2023)
摘要
Deploying deep learning models in embedded terminals is essential for applications with real-time reasoning requirements. In order to make the model run efficiently in the embedded end with limited resources, we propose a model compression method combining multi-factor channel pruning and knowledge distillation. In the process of network sparsity, this method uses the double factors of the BN layer to improve the pruning standard and guides the local pruning of the model according to the new standard to ensure the compression rate. In order to further improve the accuracy, we use the knowledge transfer method in the idea of knowledge distillation to fine-tune the model and use the more continuous parameter distribution of the student model to ensure accuracy. We use several deep learning models to test. The experimental results show that the proposed model compression method has the advantages of fewer parameters and higher accuracy, which reduces the resources occupied by the model application to the embedded end. More importantly, this method not only realizes the efficient operation of various models in the embedded end, but also ensures the high accuracy of the model.
更多查看译文
关键词
model compression method,knowledge distillation,multi-factor
AI 理解论文
溯源树
样例
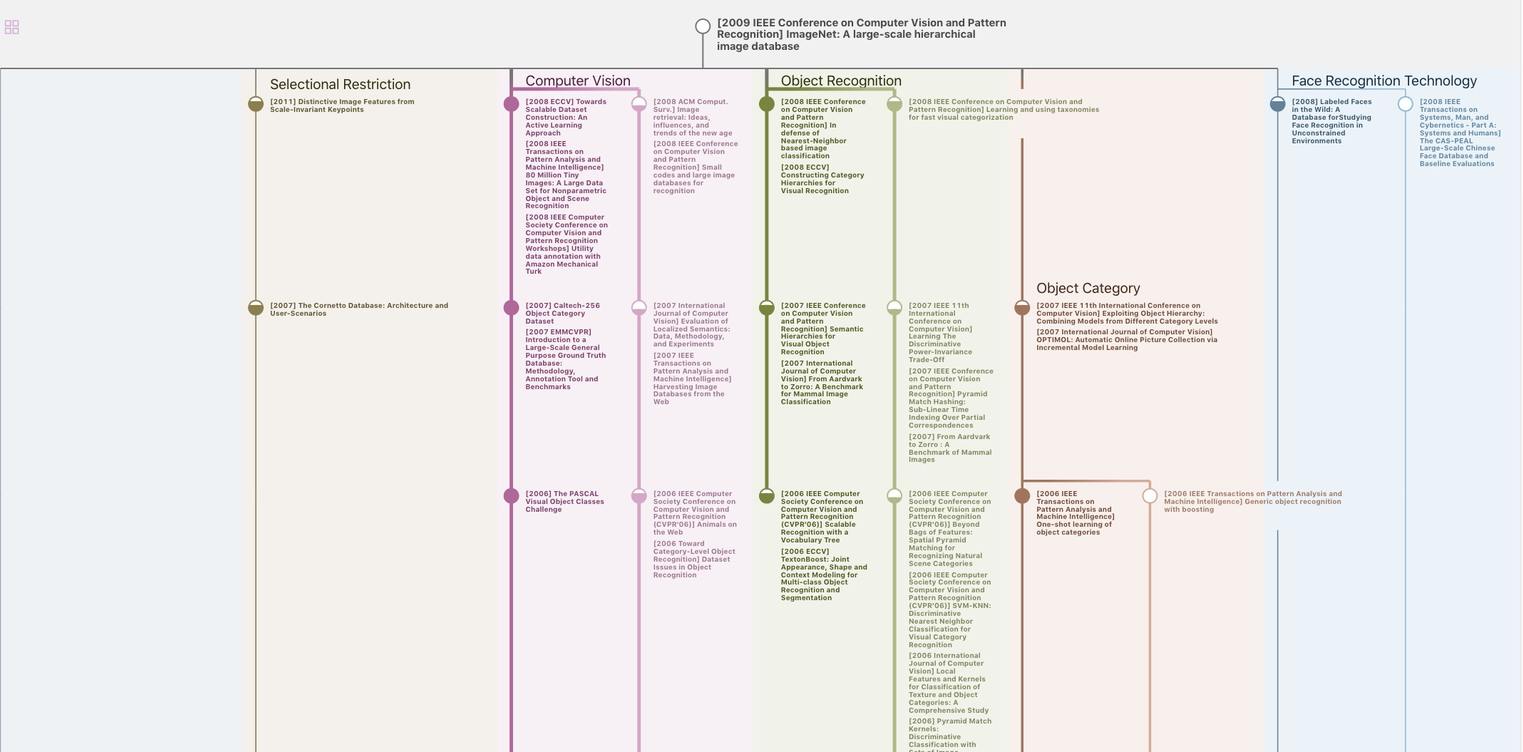
生成溯源树,研究论文发展脉络
Chat Paper
正在生成论文摘要