A Bayesian method for concurrently designing molecules and synthetic reaction networks
Science and Technology of Advanced Materials: Methods(2023)
摘要
In the last few years, de novo molecular design using machine learning has made great technical progress but there are still challenges to be overcome in their practical applications. This is mostly owing to the cost and technical difficulty of synthesizing such computationally designed molecules. To overcome such barriers, various methods for synthetic route design using deep neural networks have been studied intensively in recent years. However, little progress has been made in designing molecules and their synthetic routes simultaneously. Here, we formulate the problem of simultaneously designing molecules with the desired set of properties and their synthetic routes within the framework of Bayesian inference. The design variables consist of a set of reactants in a reaction network and its network topology. The design space is extremely large because it consists of all combinations of purchasable reactants, often in the order of millions or more. In addition, the designed reaction networks can adopt any topology beyond simple multistep linear reaction routes. To solve this hard combinatorial problem, we present a powerful sequential Monte Carlo algorithm that recursively designs a synthetic reaction network by sequentially building up single-step reactions. In a case study of designing drug-like molecules based on commercially available compounds, compared with heuristic combinatorial search methods, the proposed method showed overwhelming performance in terms of computational efficiency, coverage, and novelty with respect to existing compounds. We also provide the Python library “Seq-Stack-Reaction” with its illustrative example of designing highly viscous lubricant molecules.
更多查看译文
关键词
synthetic reaction networks,bayesian method,molecules
AI 理解论文
溯源树
样例
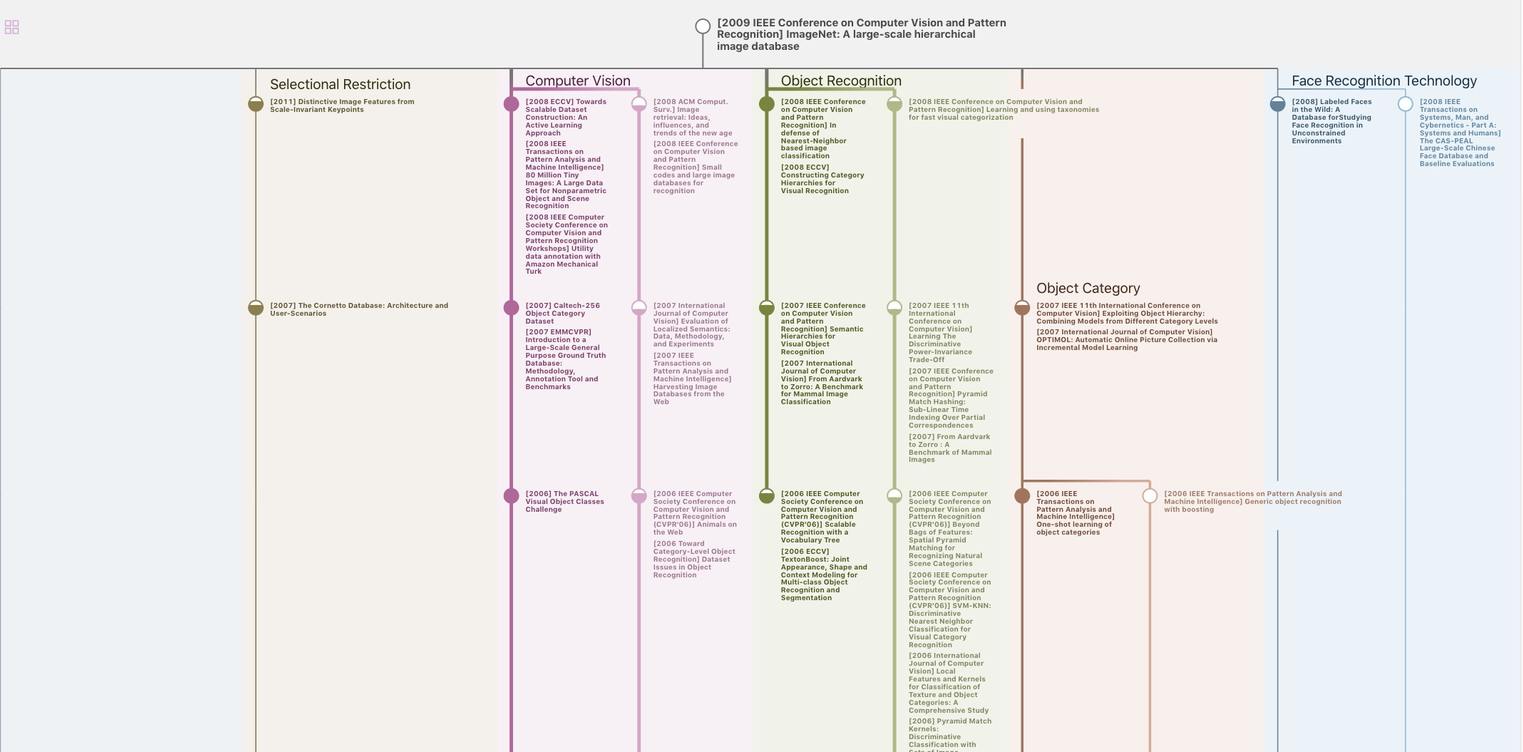
生成溯源树,研究论文发展脉络
Chat Paper
正在生成论文摘要