Hemispheric prediction of solar cycle 25 based on a deep learning technique
ADVANCES IN SPACE RESEARCH(2024)
摘要
In the current work, single-and double-layered Long Short-Term Memory (LSTM) model have been developed and compared to find the most suitable one for hemispheric prediction of solar cycle 25. The smoothed Hemispheric Sunspot Number (HSN) reconstructed by Veronig et al. (2021) has been used to achieve the objective. The deep learning models are evaluated over different pairs of train and test set for both the hemispheres using the 5-fold cross-validation scheme, to determine the best effective model for hemispheric prediction of cycle 25. Our analysis reveals that the single-layered LSTM model achieves lower RMSE when predicting the test dataset. To prove the reliability of our suggested LSTM models, we have predicted the hemispheric profiles of solar cycles 21-24. From our analysis, we found that the single-layered LSTM model performed better in comparison to the double-layered LSTM model in predicting past solar cycles 21-24. Hence, hemispheric prediction of solar cycle 25 is carried out utilizing the single-layered LSTM model due to better performance capability during experimental analysis. This work founds that the northern hemisphere will peak with an amplitude of 56 +/- 5 during July 2023 + 3 months while the southern hemisphere will have a maximum amplitude of 54 +/- 4 during June 2023 + 2 months. So, it can be said that the southern HSN will peak before the northern HSN and that the peak of northern HSN will be slightly higher than the southern one. (c) 2023 COSPAR. Published by Elsevier B.V. All rights reserved.
更多查看译文
关键词
Sun,Sunspot number,Solar cycle 25,Prediction,LSTM,Deep learning
AI 理解论文
溯源树
样例
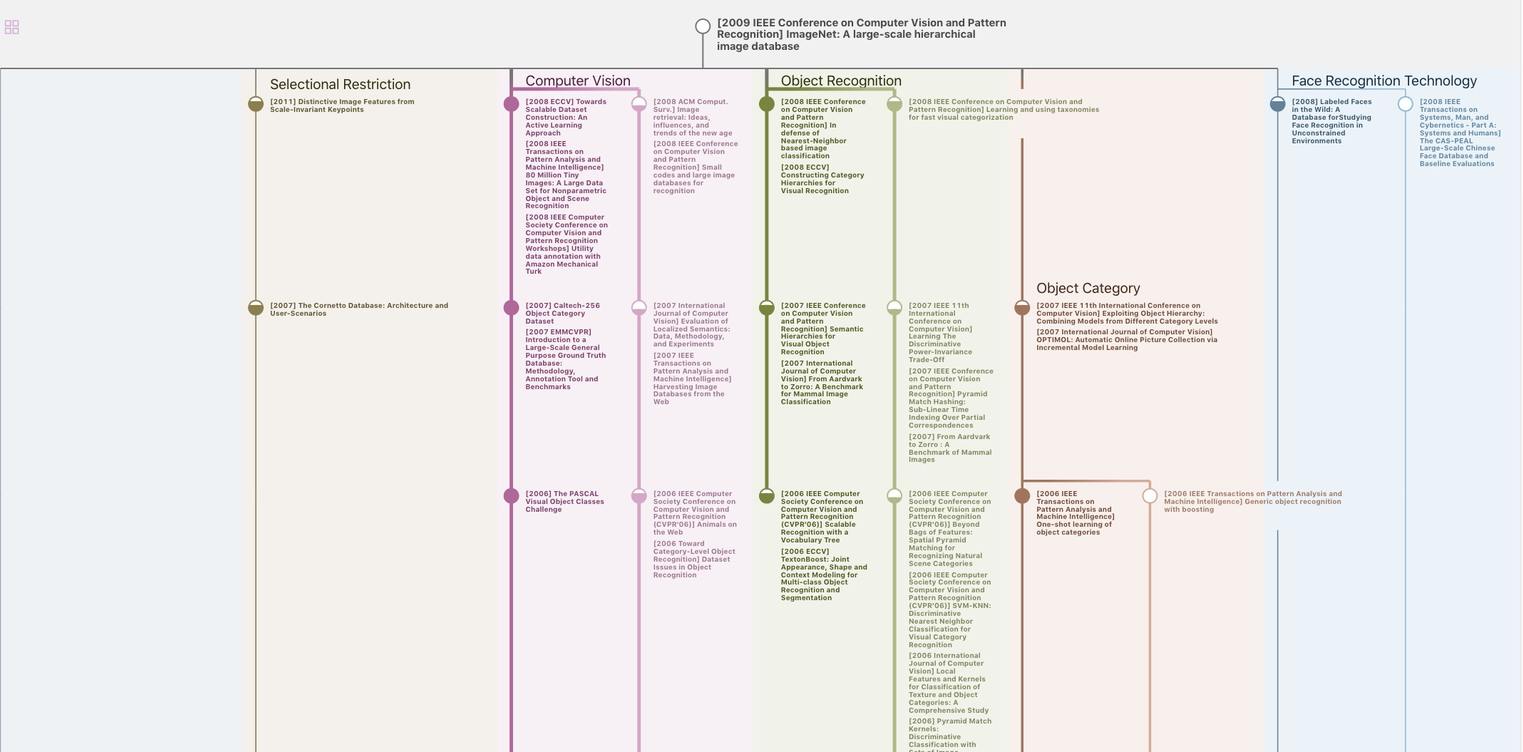
生成溯源树,研究论文发展脉络
Chat Paper
正在生成论文摘要