QARMA-FL: Quality-Aware Robust Model Aggregation for Mobile Crowdsourcing
IEEE INTERNET OF THINGS JOURNAL(2024)
摘要
Over the past few years, the improved detection and processing features of Internet of Things (IoT) devices have opened the doors to several mobile crowdsourcing (MC) applications. Federated learning (FL) is being seen as an attractive framework to address the data privacy concerns of mobile users in the context of crowdsourcing. In FL on a crowdsourcing platform, constructing an effective deep neural network (DNN) is challenging. This is primarily because the quality of the global model depends on the local model quality, which can vary greatly due to differences in the computational resources, data quantity, and data quality provided by each worker. To address these challenges, we propose QARMA-FL: quality-aware robust model aggregation for FL in crowdsourcing applications, where we select the local model for aggregation based on its quality and performance. We also propose a model-quality-aware incentive mechanism to reward workers, based on their contribution to model training. Our model selection and incentive mechanism is capable of detecting free rider (FR) attacks, identifying workers who benefit from others' contributions without contributing themselves. Most existing evaluations of FL in MC studies are not based on the real-world FL scenarios. Therefore, we evaluate QARMA-FL alongside a baseline FL model in a quantity-skew, non-identically distributed (IID) data setup where different workers contribute varying amounts of data for model training. Our diverse experiments validated QARMA-FL's performance, demonstrating its ability to efficiently aggregate models in MC scenarios, reaching baseline results with a reduced worker participation by 40% to 60%.
更多查看译文
关键词
Federated learning (FL),mobile crowdsource,model selection,robust model aggregation
AI 理解论文
溯源树
样例
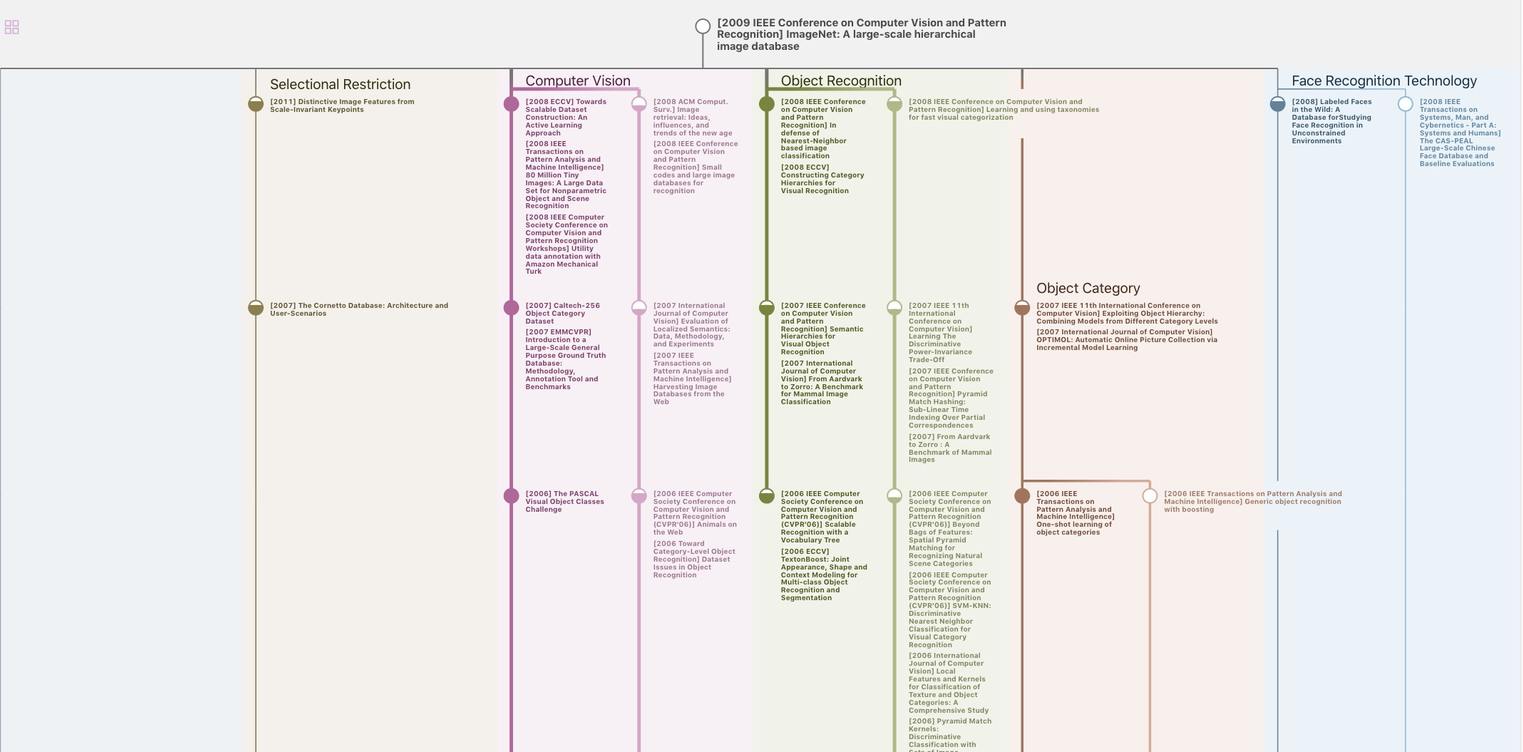
生成溯源树,研究论文发展脉络
Chat Paper
正在生成论文摘要