Multi-Scale Spatiotemporal Feature Fusion Network for Video Saliency Prediction
IEEE TRANSACTIONS ON MULTIMEDIA(2024)
摘要
Recently, video saliency prediction has attracted increasing attention, yet the improvement of its accuracy is still subject to the insufficient use of multi-scale spatiotemporal features. To address this issue, we propose a 3D convolutional Multi-scale Spatiotemporal Feature Fusion Network (MSFF-Net) to achieve the full utilization of spatiotemporal features. Specifically, we propose a Bi-directional Temporal-Spatial Feature Pyramid (BiTSFP), the first application of bi-directional fusion architectures in this field, which adds the flow of shallow location information on the basis of the previous flow of deep semantic information. Then, different from simple addition and concatenation, we design an Attention-Guided Fusion (AGF) mechanism that can adaptively learn the fusion weights of adjacent features to integrate them appropriately. Moreover, a Frame-wise Attention (FA) module is introduced to selectively emphasize the useful frames, augmenting the multi-scale temporal features to be fused. Our model is simple but effective, and it can run in real-time. Experimental results on the DHF1K, Hollywood-2, and UCF-sports datasets demonstrate that the proposed MSFF-Net outperforms existing state-of-the-art methods in accuracy.
更多查看译文
关键词
Video saliency prediction,multi-scale spatiotemporal features,feature fusion,attention mechanism
AI 理解论文
溯源树
样例
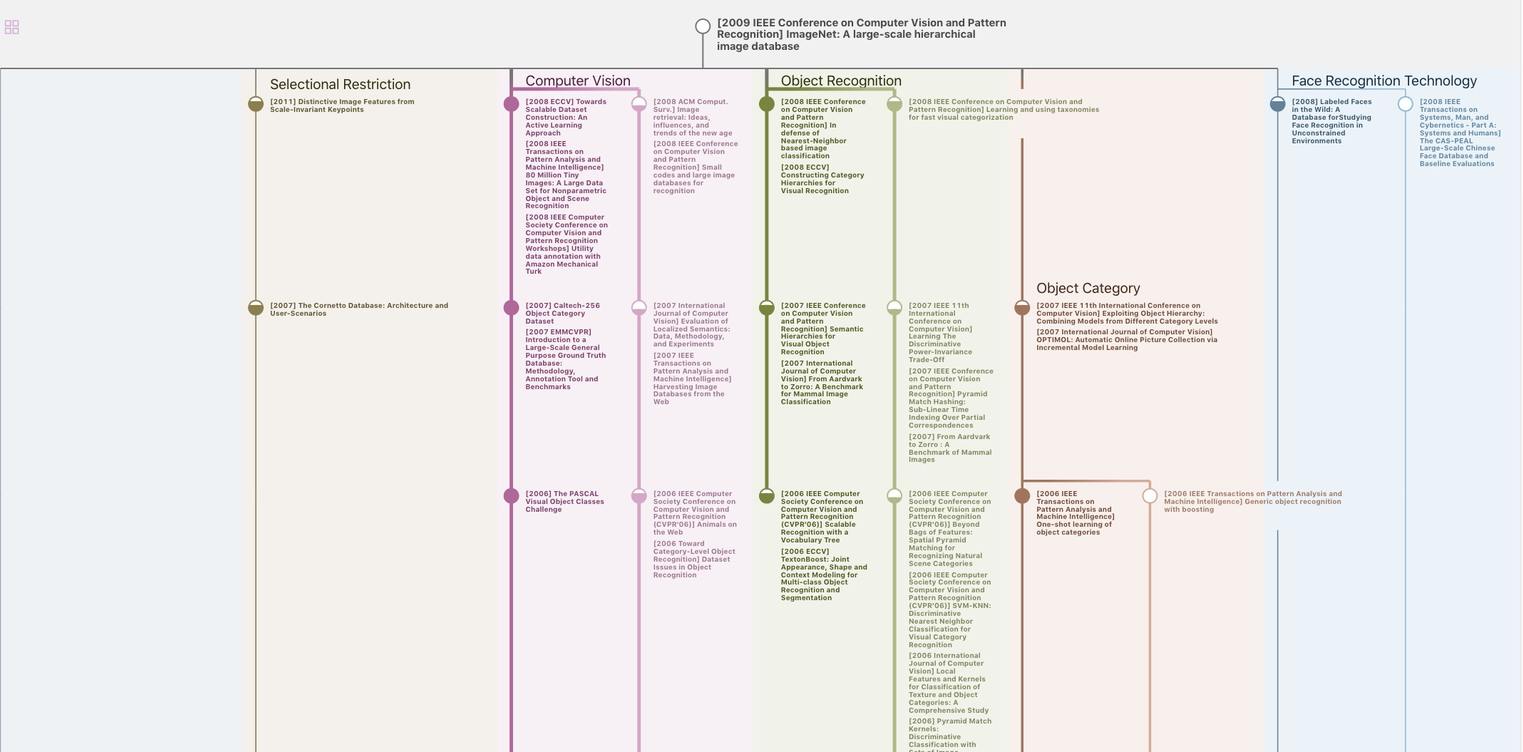
生成溯源树,研究论文发展脉络
Chat Paper
正在生成论文摘要