Identifying Risk Factors For Running-Related Injuries And Their Interrelation Using Machine Learning- A Prospective Cohort Study (Smart Injury Prevention)
MEDICINE & SCIENCE IN SPORTS & EXERCISE(2023)
摘要
PURPOSE: While individual risk factors for running-related injuries (RRI) have been previously identified, the interrelationship of risk factors has not been adequately explored. The aim of this study is to evaluate how machine learning (ML) may identify the combination of risk factors of RRI. METHODS: In this prospective cohort study over 12 months, competitive injury-free adult runners (running at least 20 km per week) were included. Baseline assessment contained medical examination, questionnaires (demographics, sport and injury history, menstruation, medication), musculoskeletal blood laboratory tests as well as biomechanical evaluation via motion capture laboratory (Qualisys AB, Sweden and Theia Markerless, Canada). For the following 12 months, injury surveillance was conducted via the Oslo Sports Trauma Research Center questionnaire (OSTRC-H2 on athletemonitoring.com; FITSTATS Technologies, Inc., Canada). Running exposure was assessed via GPS data (strava.com; Strava, Inc., USA). Any reported injury was followed up and precisely diagnosed by a medical assessment with possible imaging in a specialized running clinic. The main outcome was biomechanical, training-related, and medical baseline risk factors attributing to RRI represented by the injury incidence (injuries per 1000 h running). A ML model will be used to learn the interrelation and ranking of possible risk factors with a sensitivity analysis. RESULTS: Overall, 120 runners (33.3% female, 40.4 ± 10.1 years, 176.4 ± 7.9 cm, 70.6 ± 9.5 kg, BMI 22.6 ± 10.1 kg/m2) were recruited and 114 (95%) reported to the injury monitoring during 11,827 hours of running. Fifty injured runners (prevalence 43.9%, 95%CI 31.7 to 56.0%), reported 94 new injuries corresponding to an incidence rate of 8.0 injuries per 1000 h of running (95%CI 5.7 to 10.2). Of these injuries, most were Achilles tendinopathies (n = 13) and iliotibial band syndromes (n = 10). As of writing the abstract, the ML analysis is still to be conducted. CONCLUSIONS: This prospective cohort study used an extensive baseline assessment with state-of-the-art injury surveillance. A machine learning approach will be used to determine the interrelation of risk factors for RRI which may be a possible base for novel injury risk reduction strategies.
更多查看译文
AI 理解论文
溯源树
样例
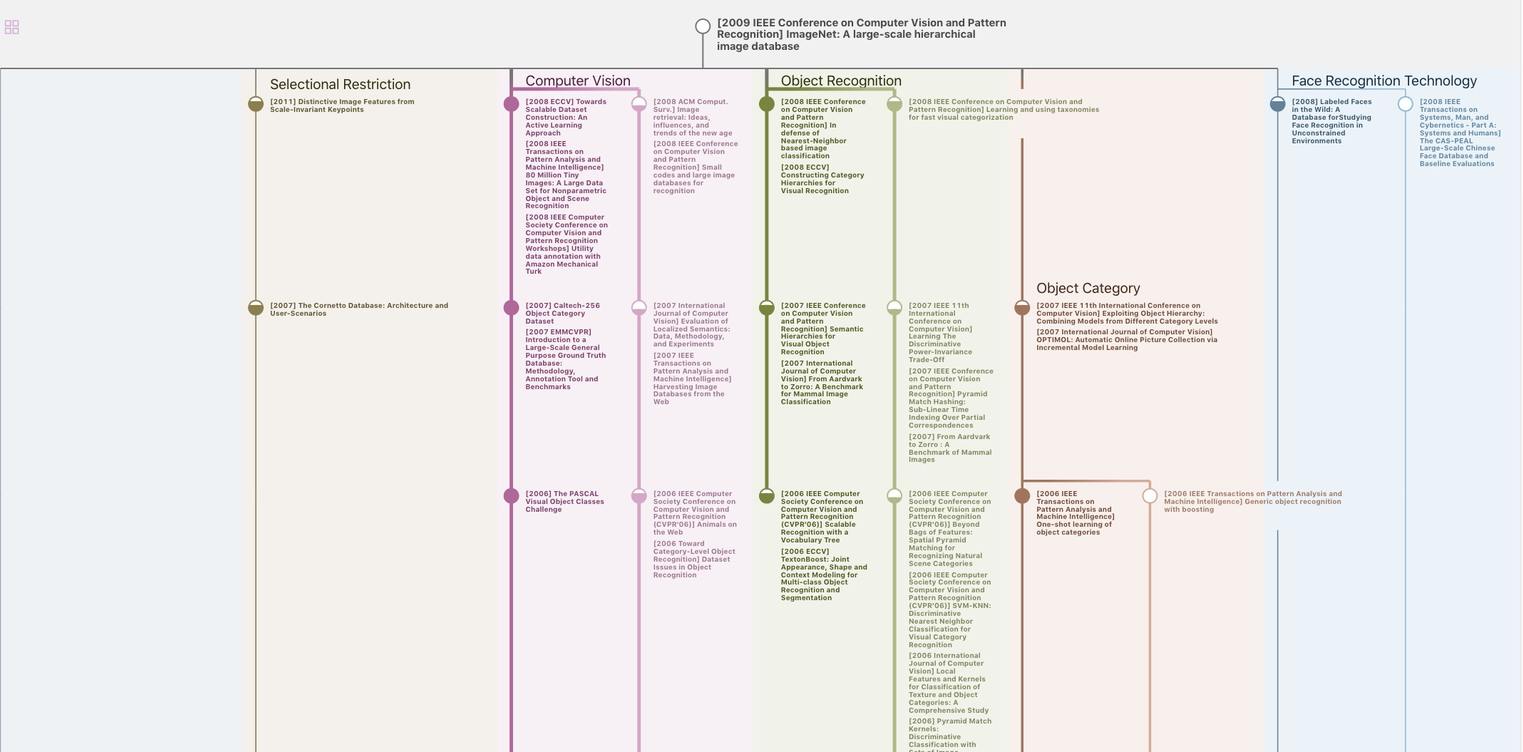
生成溯源树,研究论文发展脉络
Chat Paper
正在生成论文摘要