Joint Adversarial Domain Adaptation with Structural Graph Alignment.
IEEE transactions on network science and engineering(2024)
摘要
Generative adversarial networks as a powerful technique is also used in domain adaptation (DA) problem. Existing adversarial DA methods mainly conduct domain-wise alignment to alleviate marginal distribution shift between the two domains, while it may damage latent discriminative structure hidden in data feature space and cause negative transfer accordingly. To handle this problem, we propose a joint adversarial domain adaptation method with structural graph alignment to minimize joint distribution bias by further realizing class-wise matching (conditional distribution shift) based on a simple sampling strategy except for the domain-wise alignment, and validate that simultaneously considering these two types of shift can approximately reduce the joint distribution bias. To explore transferable structural information and realize more sufficient transfer for DA problem, we propose to align structural graphs between the two domains which is also based on a simple sampling strategy. Notably, the structural graph describes the relationship between each two samples and it is computed on two domains. As such, we can learn new feature representation of the two domains which are more discriminative and transferable to benefit a cross-domain classification task desirably. Finally, we design a number of experiments to evaluate our approach on four public cross-domain benchmark datasets including standard and large-scale ones, and empirical results show that the proposed model can outperform compared state-of-the-art methods.
更多查看译文
关键词
Feature extraction,Adaptation models,Adversarial machine learning,Training,Task analysis,Standards,Shape,Joint adversarial domain adaptation,marginal distribution,conditional distribution,joint distribution,structural graph alignment
AI 理解论文
溯源树
样例
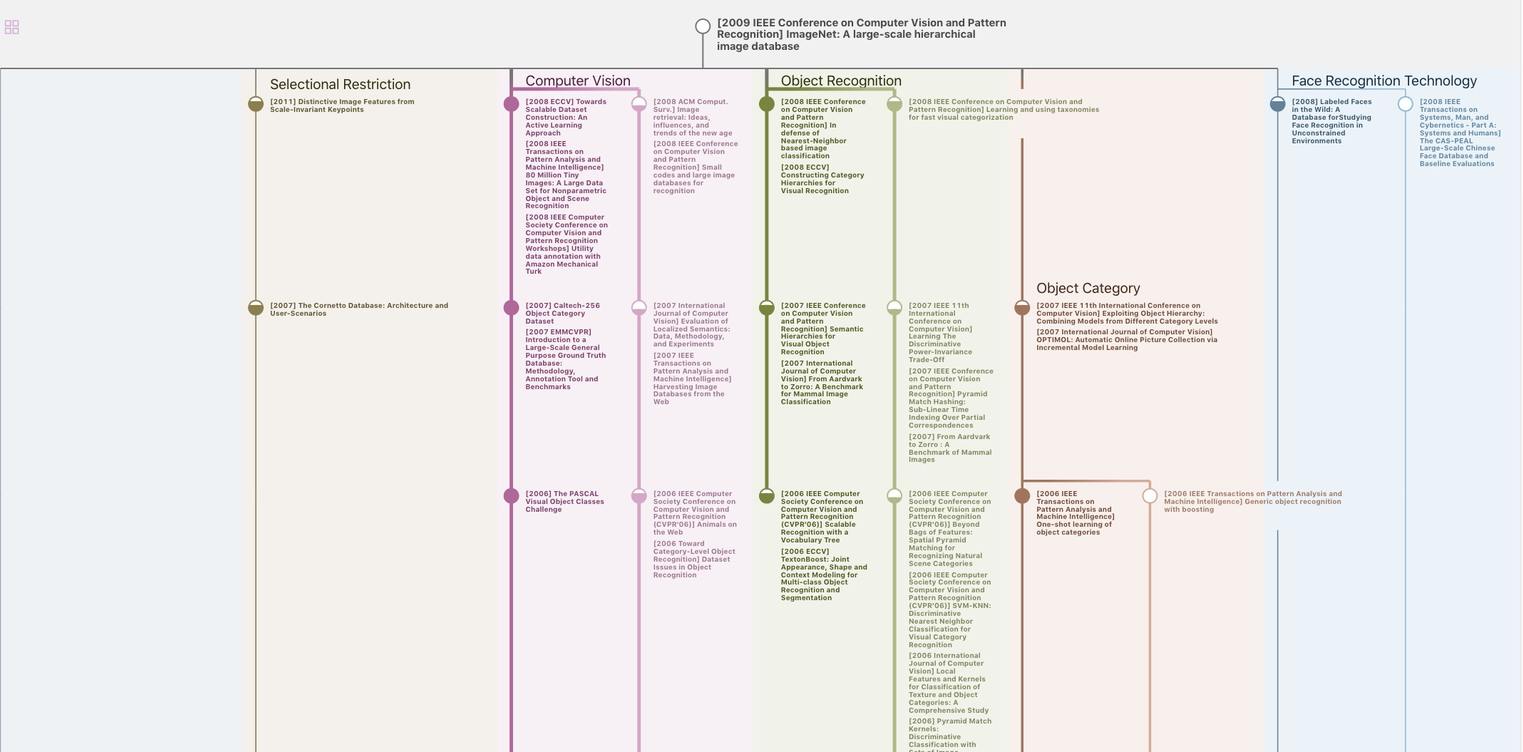
生成溯源树,研究论文发展脉络
Chat Paper
正在生成论文摘要