Fuzzy Centered Explainable Network for Reinforcement Learning
IEEE TRANSACTIONS ON FUZZY SYSTEMS(2024)
摘要
The explainability of reinforcement learning (RL) models has received vast amount of interest as its applications have widened. Most existing explainable RL models focus on improving the explainability of an agent's observations instead of the relationships between agent states and actions. This study presents a fuzzy centered explainable network (FCEN) for RL tasks to interpret the relationships between agent states and actions. The proposed FCEN leverages the interpretability of fuzzy neural networks to establish if-then rules and a generative model to visualize learned knowledge. Precisely, the FCEN includes if-then rules that formulate state-action mappings with human-understandable logic, such as the form "IF Input is A THEN Output is B." In addition, these rules connect with a generative model that concretizes the states into human-understandable patterns (figures). Our experimental results obtained on 4 Atari games show that the proposed FCEN can achieve a high level of performance in RL tasks and enormously boost the explainability of RL agents both globally and locally. In other words, the FCEN maintains a high-level explanation for the agent decision logic and the possibility of low-level analysis for each given observation sample. The explainability boost does not undermine reward learning performance, humans can even enhance the agent's performance with the provided explainability.
更多查看译文
关键词
Explainable AI (xAI),fuzzy neural network (FNN),generative model,reinforcement learning (RL)
AI 理解论文
溯源树
样例
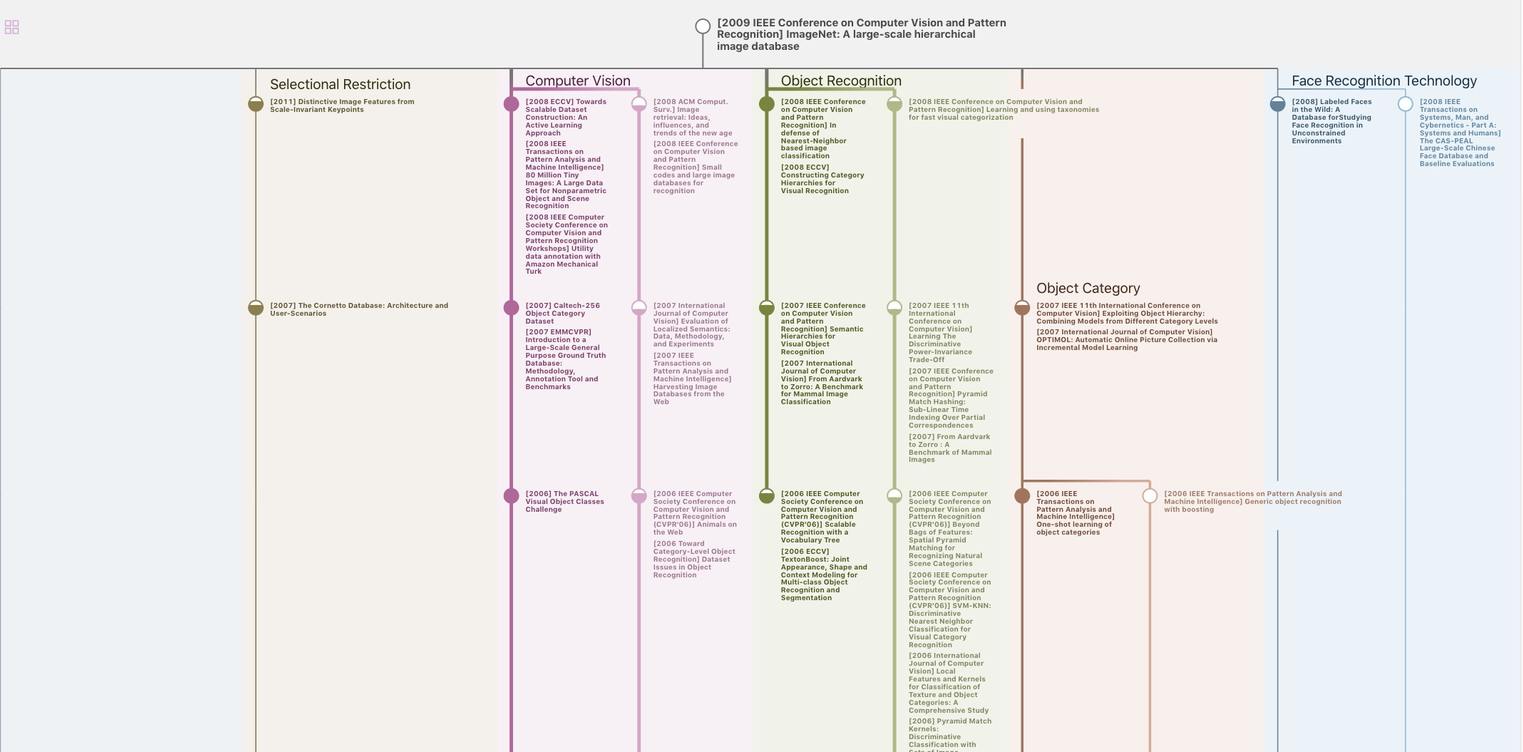
生成溯源树,研究论文发展脉络
Chat Paper
正在生成论文摘要