Leaf chlorophyll content retrieval for AVIRIS-NG imagery using different feature selection and wavelet analysis
ADVANCES IN SPACE RESEARCH(2024)
摘要
The Leaf Chlorophyll Content (LCC) is a crucial indicator of plant vitality. It plays a crucial role in photosynthetic processes and regulates metabolic activities in plants. Thus, it is an important task for the scientific community to estimate its precise quantity. In this study, we used AVIRIS-NG imagery, a wavelet analysis, and a number of feature selection approaches to estimate the chlorophyll content of several agricultural species. Eight different Discrete Wavelet Transform (DWT) methods, including Daubechies (db), Biorthogonal (bior), Reverse biorthogonal (rbio), etc., were employed to generate denoised vegetation spectra, in which bior produced the best approximation. Recursive Feature Elimination (RFE), Regularized Random Forest (RRF), Least Absolute Shrinkage and Selection Operator (LASSO), and Partial Least Square (PLS) were used to select features from the approximated signals, and the top three bands were chosen to be used in the creation of new indices for LCC retrieval. In order to estimate LCC, linear regression models were developed using these indicators. The best results were obtained by the PLS-based LCC retrieval model, with a correlation of r = 0.948, a Root Mean Square Error (RMSE) of 5.464, and a bias of 3.305. The LASSO model yielded the worst results. Hence, for any hyperspectral images such as of AVIRIS-NG, the chlorophyll content may be reliably estimated using a PLS model combined with wavelet analysis. (c) 2023 COSPAR. Published by Elsevier B.V. All rights reserved.
更多查看译文
关键词
Wavelets,Denoising spectra,AVIRIS-NG,Leaf chlorophyll content,Feature selection
AI 理解论文
溯源树
样例
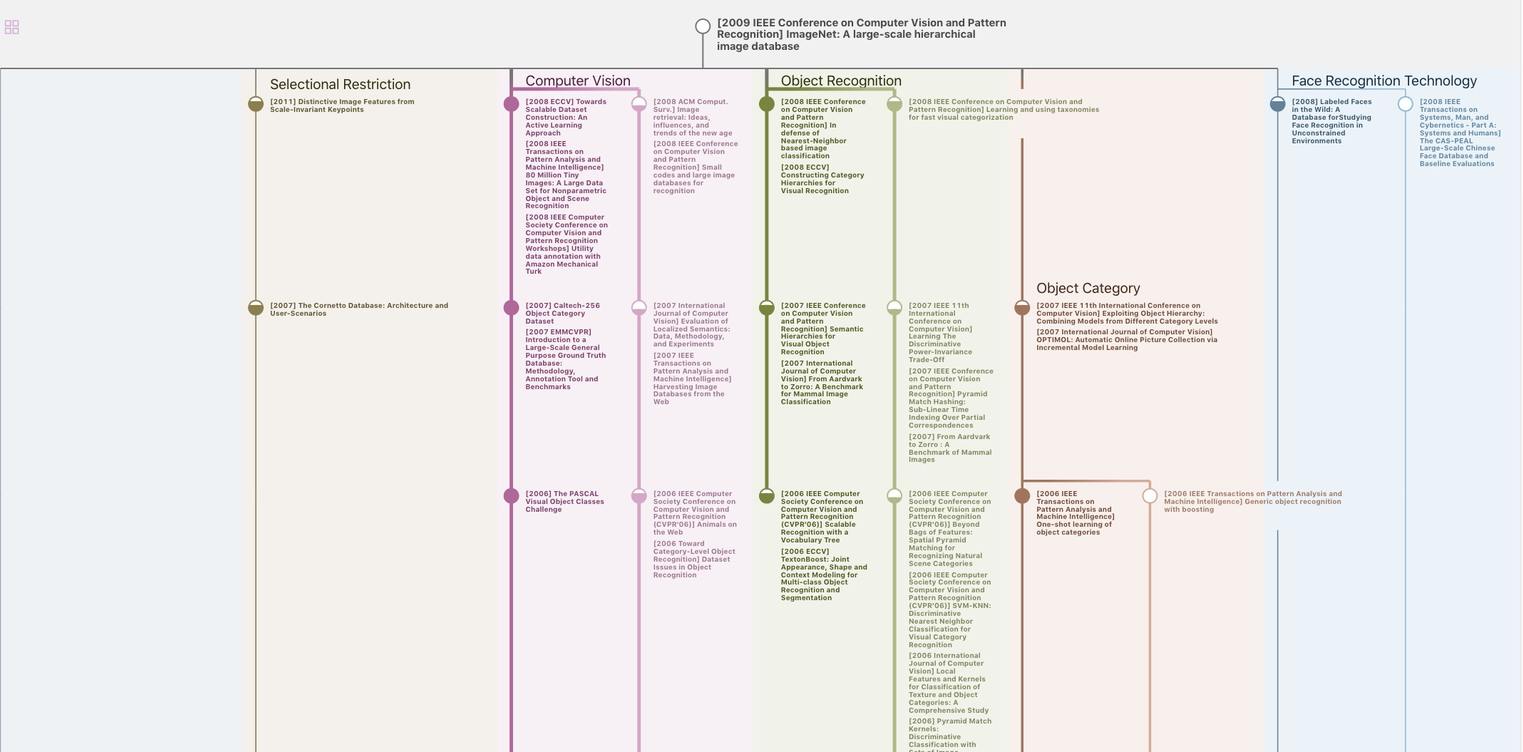
生成溯源树,研究论文发展脉络
Chat Paper
正在生成论文摘要