Exploiting Noise as a Resource for Computation and Learning in Spiking Neural Networks
arXiv (Cornell University)(2023)
摘要
$\textbf{Formal version available at}$ https://cell.com/patterns/fulltext/S2666-3899(23)00200-3 Networks of spiking neurons underpin the extraordinary information-processing capabilities of the brain and have become pillar models in neuromorphic artificial intelligence. Despite extensive research on spiking neural networks (SNNs), most studies are established on deterministic models, overlooking the inherent non-deterministic, noisy nature of neural computations. This study introduces the noisy spiking neural network (NSNN) and the noise-driven learning rule (NDL) by incorporating noisy neuronal dynamics to exploit the computational advantages of noisy neural processing. NSNN provides a theoretical framework that yields scalable, flexible, and reliable computation. We demonstrate that NSNN leads to spiking neural models with competitive performance, improved robustness against challenging perturbations than deterministic SNNs, and better reproducing probabilistic computations in neural coding. This study offers a powerful and easy-to-use tool for machine learning, neuromorphic intelligence practitioners, and computational neuroscience researchers.
更多查看译文
关键词
spiking
AI 理解论文
溯源树
样例
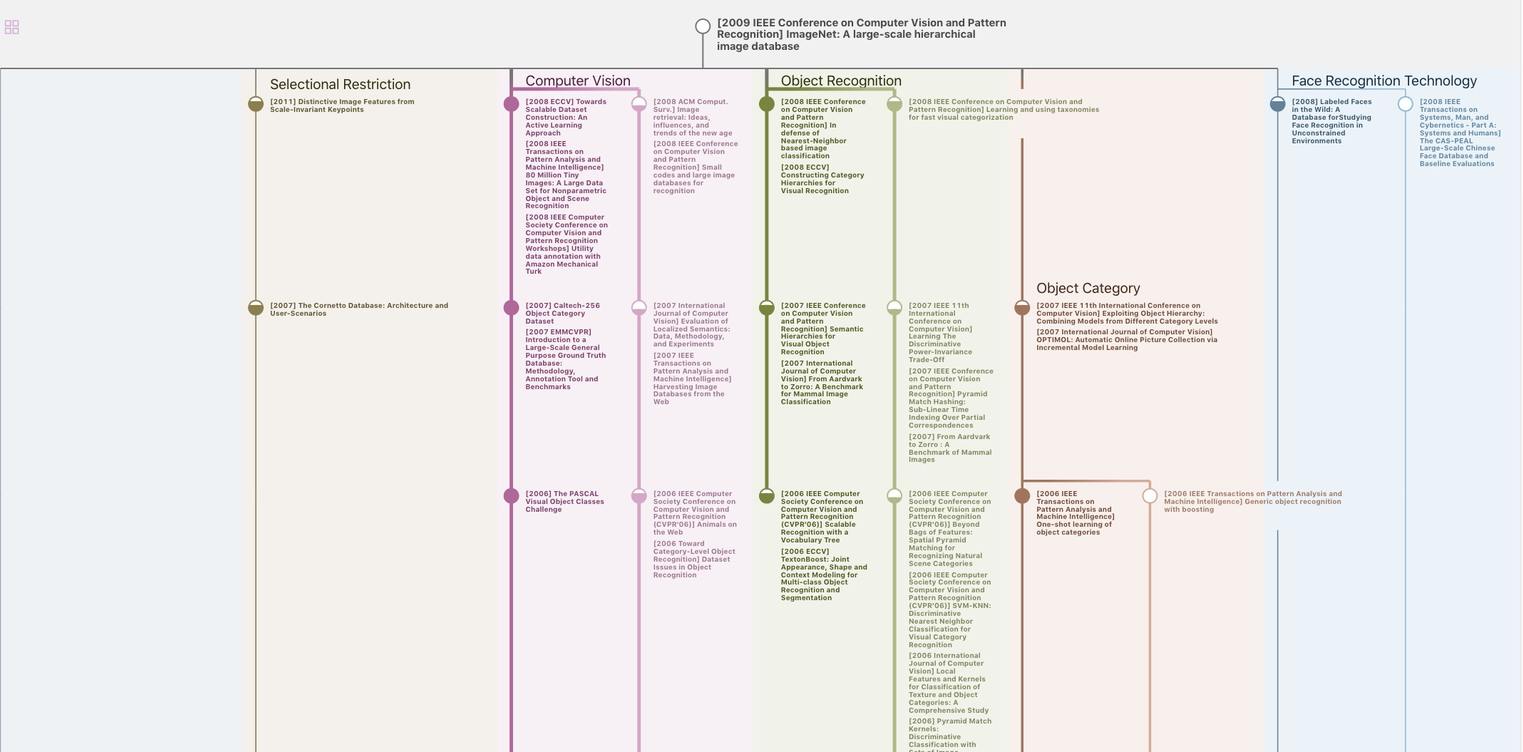
生成溯源树,研究论文发展脉络
Chat Paper
正在生成论文摘要