Bounding Box Vectorization for Oriented Object Detection With Tanimoto Coefficient Regression.
IEEE Trans. Multim.(2024)
摘要
Current oriented object detection methods mainly utilize a vanilla coordinate-angle representation for bounding box regression, which usually suffers from inconsistency between the bounding box regression losses and prediction errors induced with respect to different rotation angles, aspect ratios, and scales. Therefore, although the existing oriented object detectors have achieved very good performances under coarse evaluation metrics such as AP50, their performance significantly degrades when using stricter evaluation metric such as AP75. To address the abovementioned issues, we propose a new regression method with bounding box vectorization that implicitly represents the shape and orientation of an object with a set of orthogonal vectors. By doing this, the proposed method delicately avoids the inconsistency issues encountered in oriented bounding box regression. During training, we introduce the Tanimoto coefficient to evaluate the similarity of the bounding box vector in a shape- and orientation-aware manner, and we refer to the proposed box-to-vector loss as the B2V loss. In addition to 2D object detection, the proposed method can be easily generalized to 3D scenarios involving orientation estimation, such as autonomous driving. We evaluate the proposed method through extensive experiments conducted on four popular oriented object detection datasets, including both 2D and 3D datasets, where the proposed method significantly outperforms the recently developed state-of-the-art methods when using a more accurate evaluation metric.
更多查看译文
关键词
Oriented object detection,bounding box regression,implicit vector representation
AI 理解论文
溯源树
样例
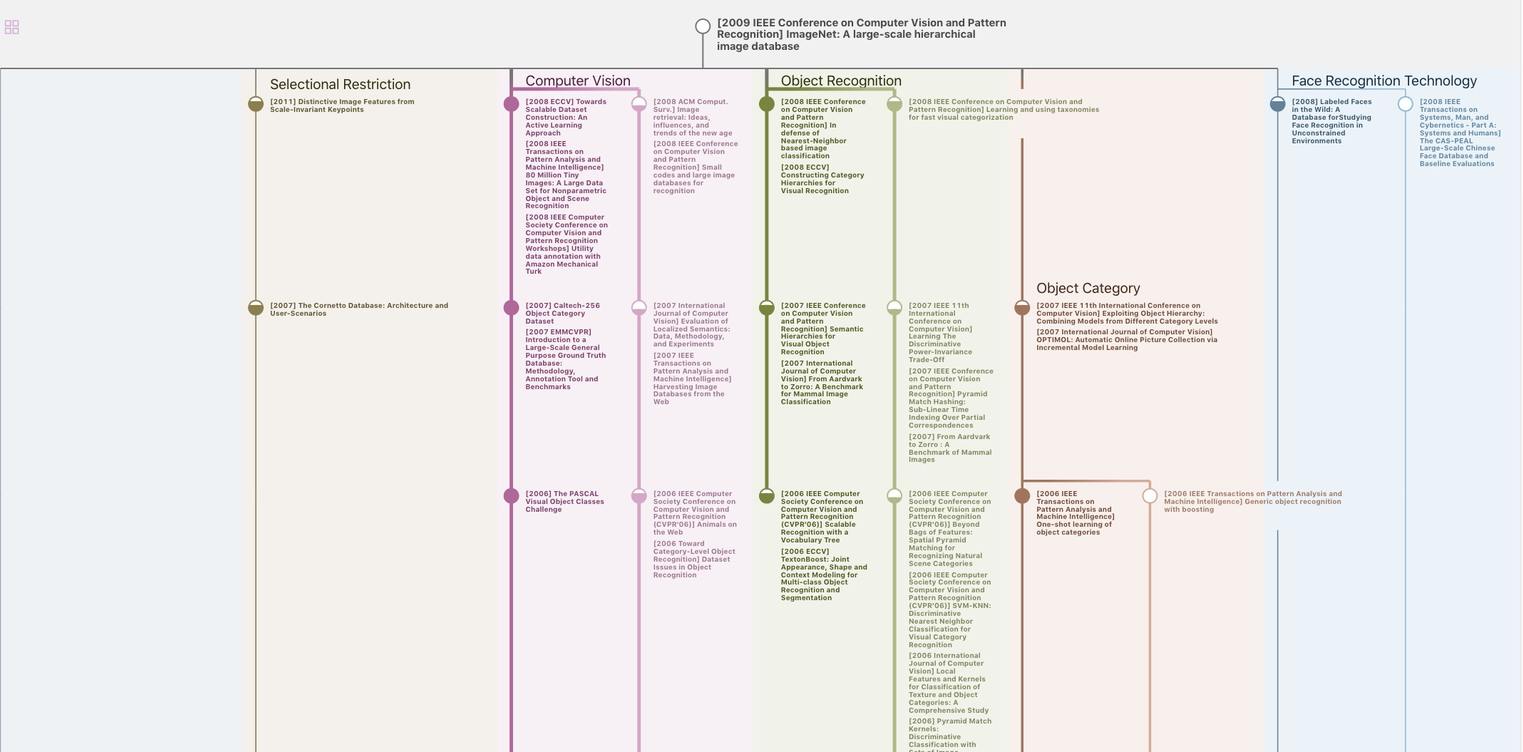
生成溯源树,研究论文发展脉络
Chat Paper
正在生成论文摘要