Distributed Resource Allocation With Federated Learning for Delay-Sensitive IoV Services.
IEEE Trans. Veh. Technol.(2024)
摘要
In this paper, we propose a distributed sidelink resource allocation approach named federated multi-agent deep Q network (FMDQN). The objective is the network spectrum-energy efficiency (SEE) maximization. To address the optimization problem in a distributed manner, we merge federated learning and deep reinforcement learning. We establish an asynchronous federated learning framework by facilitating local model training, thus avoiding the transmission of user-sensitive raw data. Our proposed approach utilizes a client-server architecture and federated aggregation. Additionally, we formulate a sub-band and power allocation strategy that aims to maximize SEE while adhering to strict delay limits for the given task. Simulation results illustrate the favorable convergence of the proposed algorithm in highly dynamic and uncertain Internet of vehicles (IoV) environments.
更多查看译文
关键词
Federated learning,Internet of vehicles,wireless resource allocation,deep reinforcement learning,double deep Q-network
AI 理解论文
溯源树
样例
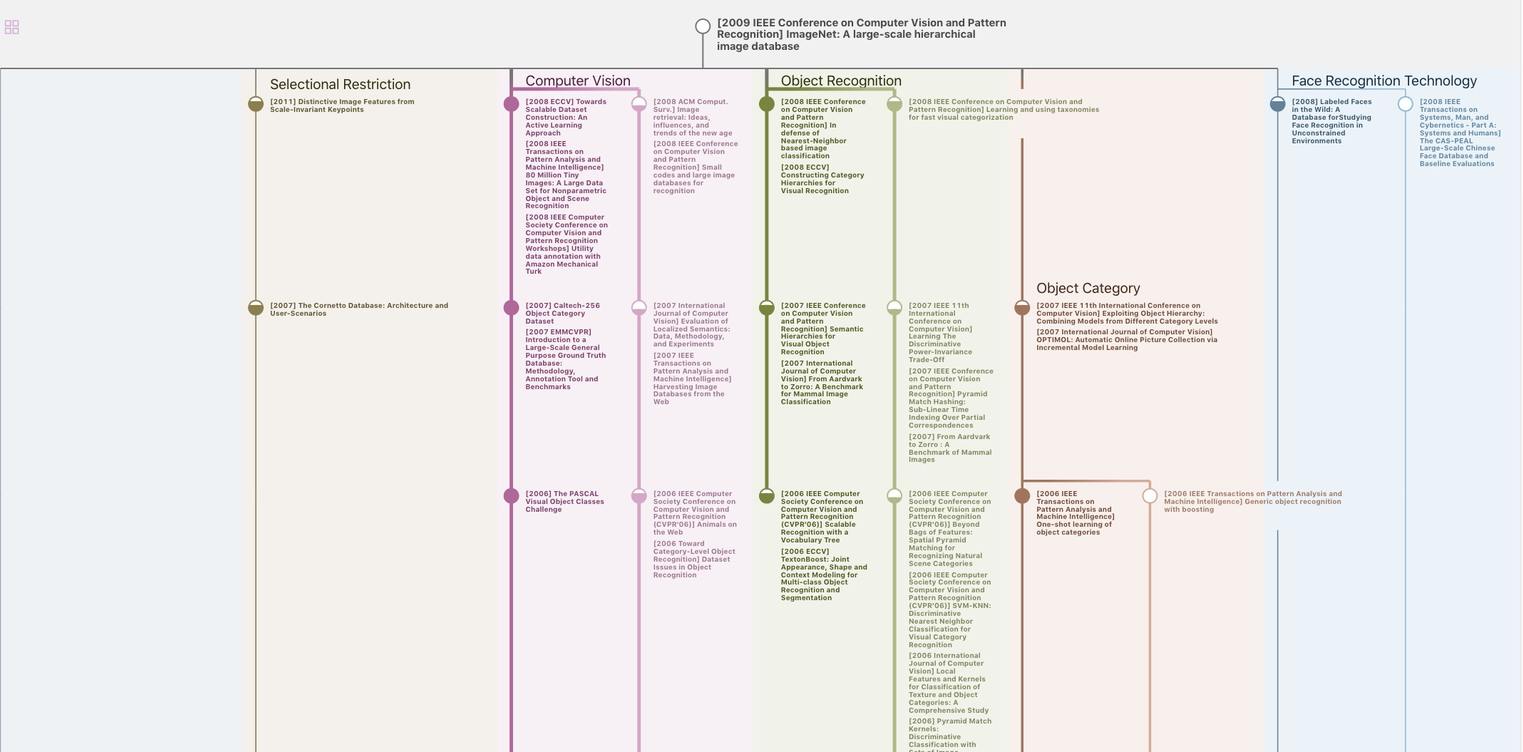
生成溯源树,研究论文发展脉络
Chat Paper
正在生成论文摘要