Demystifying the Evolution of Android Malware Variants
IEEE Transactions on Dependable and Secure Computing(2023)
摘要
It is important to understand the evolution of Android malware as this facilitates the development of defence techniques by proactively capturing malware features. So far, researchers mainly rely on dendrogram or family-tree analysis for malware's evolutionary development. However, our research finds that these techniques cannot support comprehensive malware evolution modelling, which provides a detailed explanation for why Android malware samples evolve in specific ways. This shortcoming is mainly caused by the coarse-grained clustering and analysis of malware samples. For example, because these works do not divide malware samples of a family into variant sets and explore the evolution principles among those sets, they usually fail to capture new variants that have been empowered by the feature ‘drifting’ in evolution. To address this problem, we propose a fine-grained and in-depth analysis of Android malware. Our experimental work systematically reveals the phylogenetic relationships among the variant sets for a deeper malware evolution analysis. We introduce five metrics: silhouette coefficient, creation date, variant labels, the presentativeness of the variant set formula, and the correctness of the linked edges to evaluate the correctness of our analysis. The results show that our variant clustering achieved a high silhouette value at a small sample distance (0.3), a small standard deviation (three months and 16 days) date based on when the malware samples are lastly modified, a high label consistency (91.4%), a high representativeness (93.1%) of the variant set formula. All the linked variant sets are connected based on our PhyloNet construction rules. We further analyse the coding details of Android malware for each variant set and summarise models of their evolutionary development. In this work, we successfully expose two major models of malware evolution:
active evolution
and
passive evolution
. We also disclose four technical explanations on the incentives of the two evolution models (two for each model respectively). These findings are valuable for proactive defence against newly emerged malware samples.
更多查看译文
关键词
Android,evolution,malware,phylogeny,variants
AI 理解论文
溯源树
样例
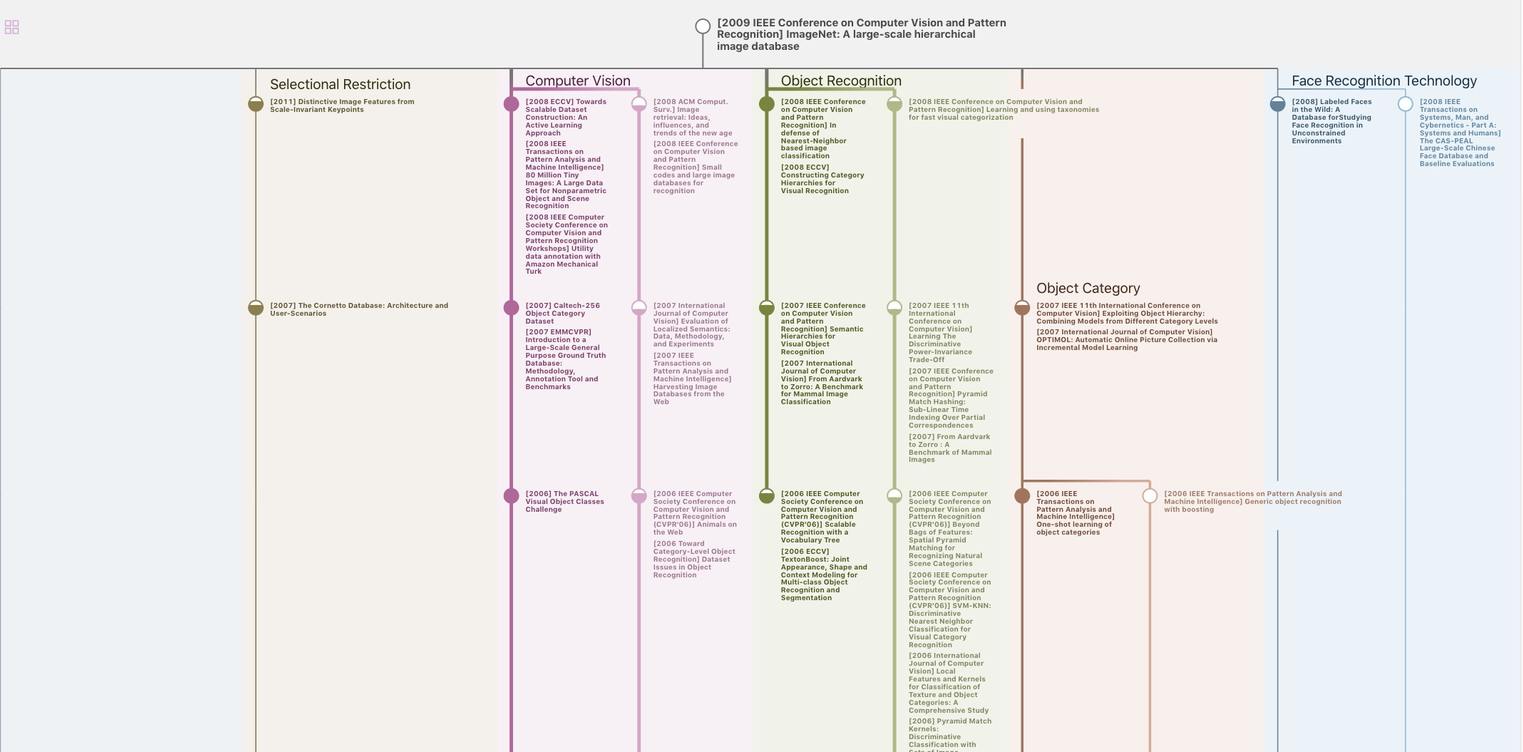
生成溯源树,研究论文发展脉络
Chat Paper
正在生成论文摘要