Improving cardiovascular risk prediction through machine learning modelling of irregularly repeated electronic health records
EUROPEAN HEART JOURNAL - DIGITAL HEALTH(2024)
摘要
Aims Existing electronic health records (EHRs) often consist of abundant but irregular longitudinal measurements of risk factors. In this study, we aim to leverage such data to improve the risk prediction of atherosclerotic cardiovascular disease (ASCVD) by applying machine learning (ML) algorithms, which can allow automatic screening of the population. Methods and results A total of 215 744 Chinese adults aged between 40 and 79 without a history of cardiovascular disease were included (6081 cases) from an EHR-based longitudinal cohort study. To allow interpretability of the model, the predictors of demographic characteristics, medication treatment, and repeatedly measured records of lipids, glycaemia, obesity, blood pressure, and renal function were used. The primary outcome was ASCVD, defined as non-fatal acute myocardial infarction, coronary heart disease death, or fatal and non-fatal stroke. The eXtreme Gradient boosting (XGBoost) algorithm and Least Absolute Shrinkage and Selection Operator (LASSO) regression models were derived to predict the 5-year ASCVD risk. In the validation set, compared with the refitted Chinese guideline-recommended Cox model (i.e. the China-PAR), the XGBoost model had a significantly higher C-statistic of 0.792, (the differences in the C-statistics: 0.011, 0.006-0.017, P < 0.001), with similar results reported for LASSO regression (the differences in the C-statistics: 0.008, 0.005-0.011, P < 0.001). The XGBoost model demonstrated the best calibration performance (men: D-x = 0.598, P = 0.75; women: D-x = 1.867, P = 0.08). Moreover, the risk distribution of the ML algorithms differed from that of the conventional model. The net reclassification improvement rates of XGBoost and LASSO over the Cox model were 3.9% (1.4-6.4%) and 2.8% (0.7-4.9%), respectively. Conclusion Machine learning algorithms with irregular, repeated real-world data could improve cardiovascular risk prediction. They demonstrated significantly better performance for reclassification to identify the high-risk population correctly.
更多查看译文
关键词
Prediction,Preventive Cardiology,Risk
AI 理解论文
溯源树
样例
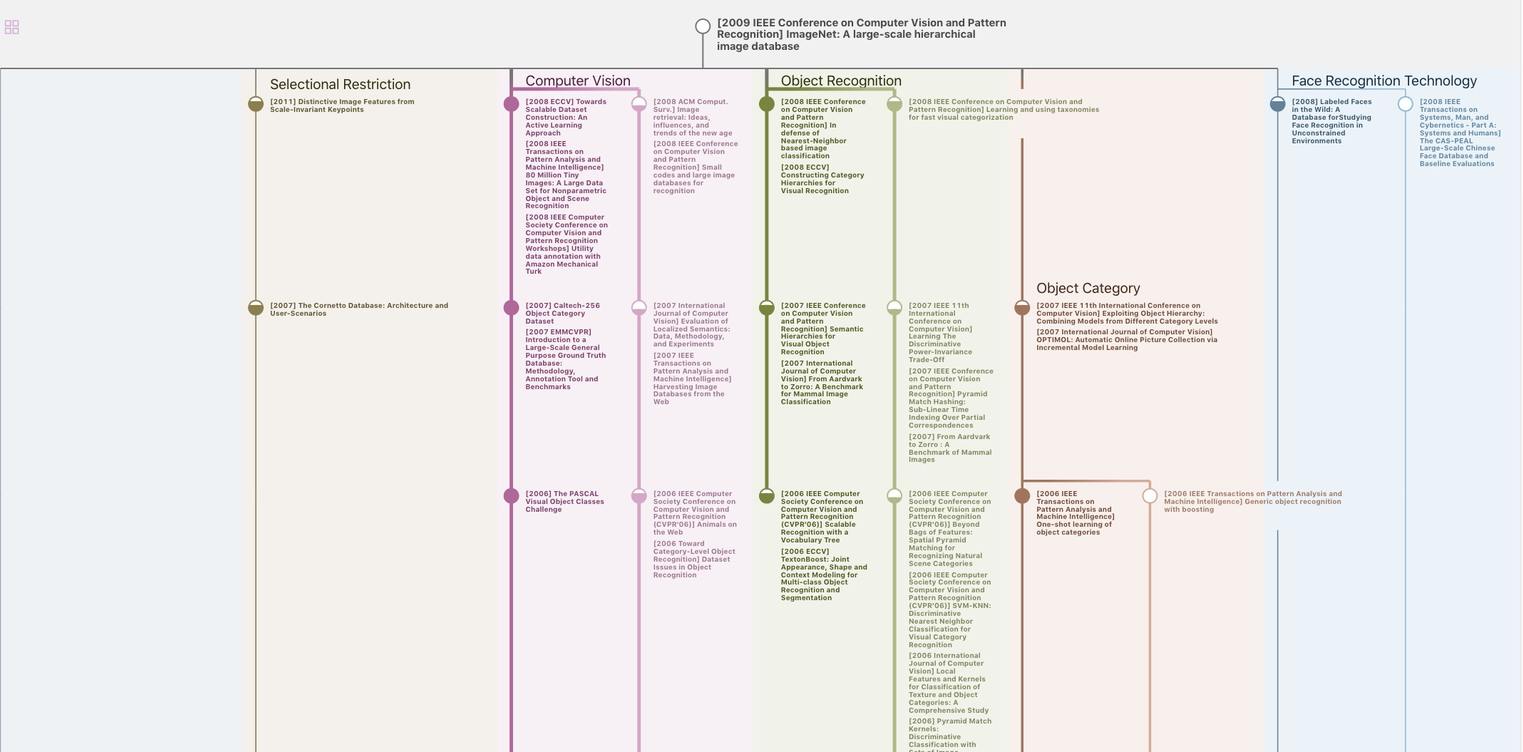
生成溯源树,研究论文发展脉络
Chat Paper
正在生成论文摘要