Automated detection of nine infantile fundus diseases and conditions in retinal images using a deep learning system
EPMA Journal(2024)
摘要
Purpose We developed an Infant Retinal Intelligent Diagnosis System (IRIDS), an automated system to aid early diagnosis and monitoring of infantile fundus diseases and health conditions to satisfy urgent needs of ophthalmologists. Methods We developed IRIDS by combining convolutional neural networks and transformer structures, using a dataset of 7697 retinal images (1089 infants) from four hospitals. It identifies nine fundus diseases and conditions, namely, retinopathy of prematurity (ROP) (mild ROP, moderate ROP, and severe ROP), retinoblastoma (RB), retinitis pigmentosa (RP), Coats disease, coloboma of the choroid, congenital retinal fold (CRF), and normal. IRIDS also includes depth attention modules, ResNet-18 (Res-18), and Multi-Axis Vision Transformer (MaxViT). Performance was compared to that of ophthalmologists using 450 retinal images. The IRIDS employed a five-fold cross-validation approach to generate the classification results. Results Several baseline models achieved the following metrics: accuracy, precision, recall, F1-score (F1), kappa, and area under the receiver operating characteristic curve (AUC) with best values of 94.62% (95% CI, 94.34%-94.90%), 94.07% (95% CI, 93.32%-94.82%), 90.56% (95% CI, 88.64%-92.48%), 92.34% (95% CI, 91.87%-92.81%), 91.15% (95% CI, 90.37%-91.93%), and 99.08% (95% CI, 99.07%-99.09%), respectively. In comparison, IRIDS showed promising results compared to ophthalmologists, demonstrating an average accuracy, precision, recall, F1, kappa, and AUC of 96.45% (95% CI, 96.37%-96.53%), 95.86% (95% CI, 94.56%-97.16%), 94.37% (95% CI, 93.95%-94.79%), 95.03% (95% CI, 94.45%-95.61%), 94.43% (95% CI, 93.96%-94.90%), and 99.51% (95% CI, 99.51%-99.51%), respectively, in multi-label classification on the test dataset, utilizing the Res-18 and MaxViT models. These results suggest that, particularly in terms of AUC, IRIDS achieved performance that warrants further investigation for the detection of retinal abnormalities. Conclusions IRIDS identifies nine infantile fundus diseases and conditions accurately. It may aid non-ophthalmologist personnel in underserved areas in infantile fundus disease screening. Thus, preventing severe complications. The IRIDS serves as an example of artificial intelligence integration into ophthalmology to achieve better outcomes in predictive, preventive, and personalized medicine (PPPM / 3PM) in the treatment of infantile fundus diseases.
更多查看译文
关键词
Deep learning,infant,Fundus disease,Retinal image,Predictive preventive personalized medicine (PPPM / 3PM)
AI 理解论文
溯源树
样例
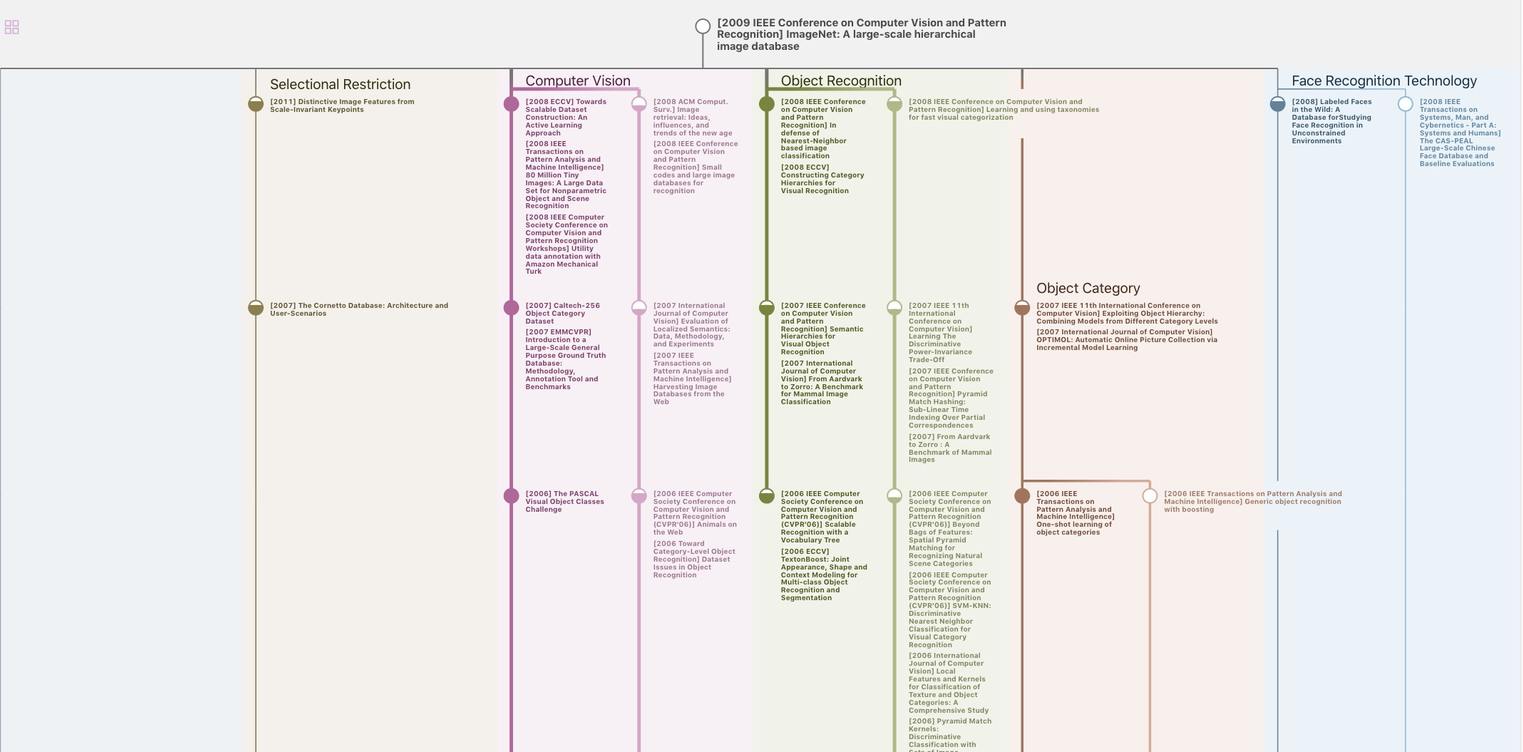
生成溯源树,研究论文发展脉络
Chat Paper
正在生成论文摘要