A Deep Learning Framework for the Classification of Pre-prodromal and Prodromal Alzheimer’s Disease Using Resting-State EEG Signals
Smart innovation, systems and technologies(2023)
摘要
Alzheimer’s Disease (AD) is a neurodegenerative disease that today represents the most common form of dementia in the elderly. The costs associated with the treatment of AD patients alone have tripled in less than twenty years, pointing out the problem of managing diagnosis and treatment and highlighting the need for effective therapeutic interventions. The identification of cases of AD in its preclinical phase, through an early diagnosis already in its asymptomatic stages, could allow more appropriate treatment options and better overall management of the disease. In this work, we designed and developed a Deep Learning (DL) algorithm for the classification of patients with Subjective Cognitive Decline (SCD) and Mild Cognitive Impairment (MCI) starting from the EEG signal, with the aim of early diagnosing the course of Alzheimer’s Disease in its initial manifestations. EEG recordings of 35 SCD subjects and 32 MCI patients have been acquired at resting state. After preprocessing, a graphical representation of the input data has been generated splitting the EEG signal into non-overlapping epochs of 5 s and applying a Continuous Wavelet Transform (CWT). The images have been used to train and test a DL model, based on the ResNet-18 architecture coupled with a Recurrent Neural Network, to classify the input temporal sequence. The performance of the model has been evaluated in terms of Accuracy, Sensitivity, and Specificity, with results of 75.0%, 66.7%, and 81.8%, respectively. The implemented method and relative performances demonstrate the effectiveness of the proposed approach for the identification of SCD and MCI, through biomarkers obtainable from minimally invasive diagnostic investigations.
更多查看译文
关键词
pre-prodromal alzheimers,deep learning,deep learning framework,classification,resting-state
AI 理解论文
溯源树
样例
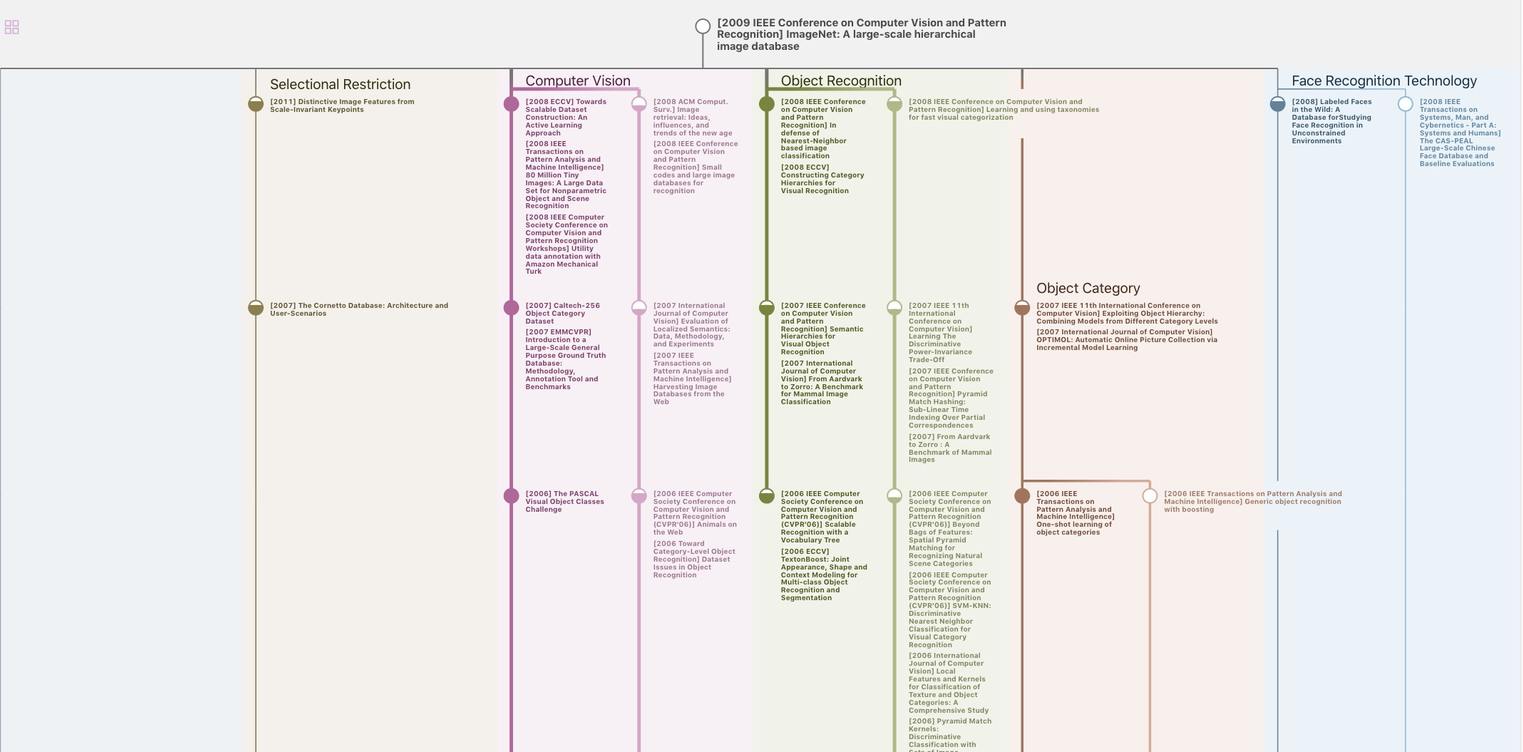
生成溯源树,研究论文发展脉络
Chat Paper
正在生成论文摘要