Variational Neuron Shifting for Few-Shot Image Classification Across Domains
IEEE TRANSACTIONS ON MULTIMEDIA(2024)
摘要
Few-shot image classification aims to recognize unseen classes with few labeled samples. Existing meta-learning models learn the ability of learning good representation or model parameters, in order to adapt to new tasks with a few training samples. However, when there exists a domain gap between training and test tasks, the learned ability often does not generalize well across domains, resulting in degraded performance on new tasks. In this article, we propose variational neuron shifting to generate adapted feature representations for few-shot learning. To do so, we introduce a working memory module to store the shifted neurons from the support set, which will be accessed to generate adapted feature representations of query samples. Under the meta-learning paradigm, the model is learned to acquire the ability of adaptation with single sample at meta-training time so as to further adapt itself to each single test sample at meta-test time. We formulate the adaptation process as a variational Bayesian inference problem, which incorporates the test sample as the condition into the generation of the model neuron shifting. We conduct extensive experiments on both within and across domain few-shot classification tasks. The new state-of-the-art performance substantiates the effectiveness of our variational neuron shifting. The thorough ablation studies further demonstrate the benefit of each component in our model.
更多查看译文
关键词
Meta learning,few-shot image classification,domain generalization,variational inference
AI 理解论文
溯源树
样例
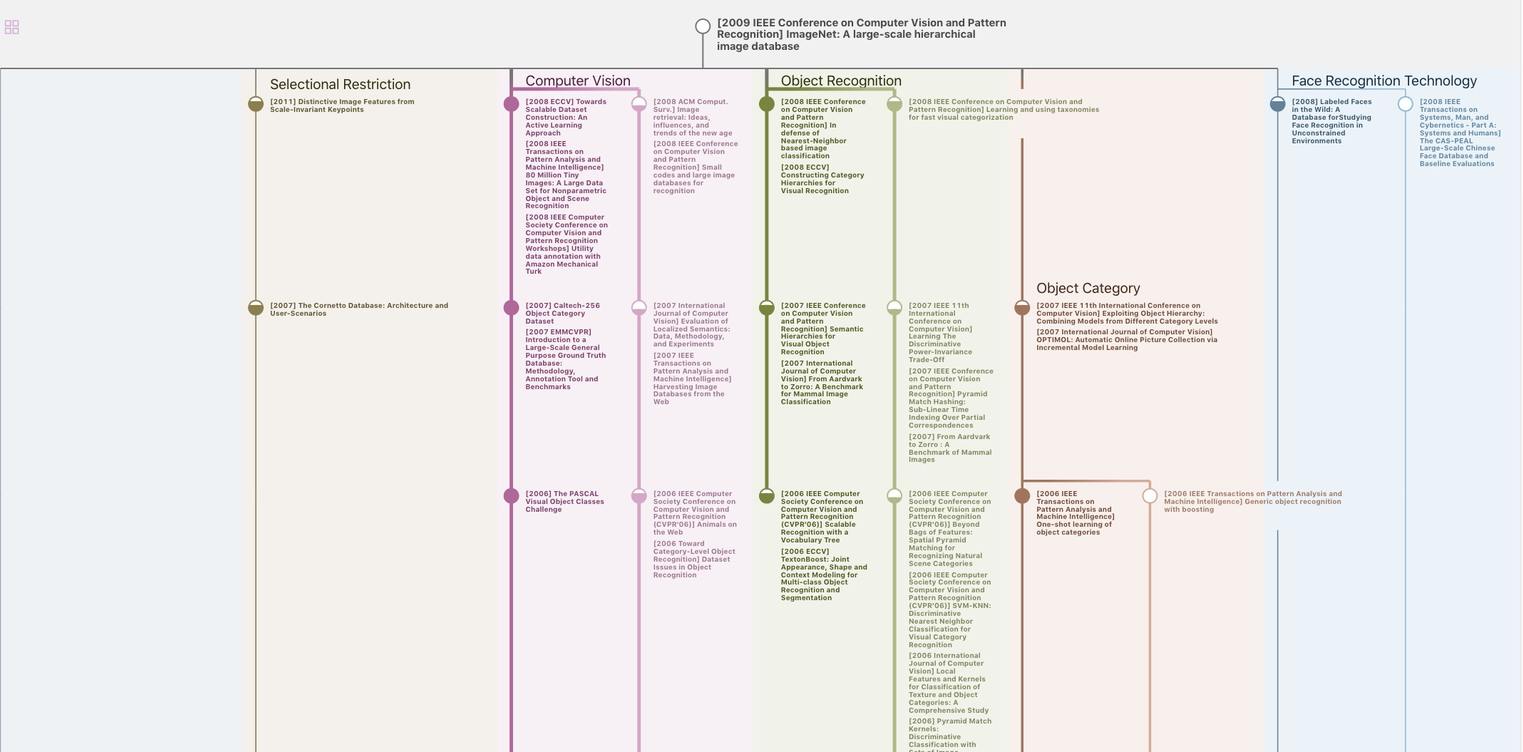
生成溯源树,研究论文发展脉络
Chat Paper
正在生成论文摘要