Prediction with Incomplete Data under Agnostic Mask Distribution Shift
arXiv (Cornell University)(2023)
摘要
Data with missing values is ubiquitous in many applications. Recent years have witnessed increasing attention on prediction with only incomplete data consisting of observed features and a mask that indicates the missing pattern. Existing methods assume that the training and testing distributions are the same, which may be violated in real-world scenarios. In this paper, we consider prediction with incomplete data in the presence of distribution shift. We focus on the case where the underlying joint distribution of complete features and label is invariant, but the missing pattern, i.e., mask distribution may shift agnostically between training and testing. To achieve generalization, we leverage the observation that for each mask, there is an invariant optimal predictor. To avoid the exponential explosion when learning them separately, we approximate the optimal predictors jointly using a double parameterization technique. This has the undesirable side effect of allowing the learned predictors to rely on the intra-mask correlation and that between features and mask. We perform decorrelation to minimize this effect. Combining the techniques above, we propose a novel prediction method called StableMiss. Extensive experiments on both synthetic and real-world datasets show that StableMiss is robust and outperforms state-of-the-art methods under agnostic mask distribution shift.
更多查看译文
关键词
prediction,incomplete data
AI 理解论文
溯源树
样例
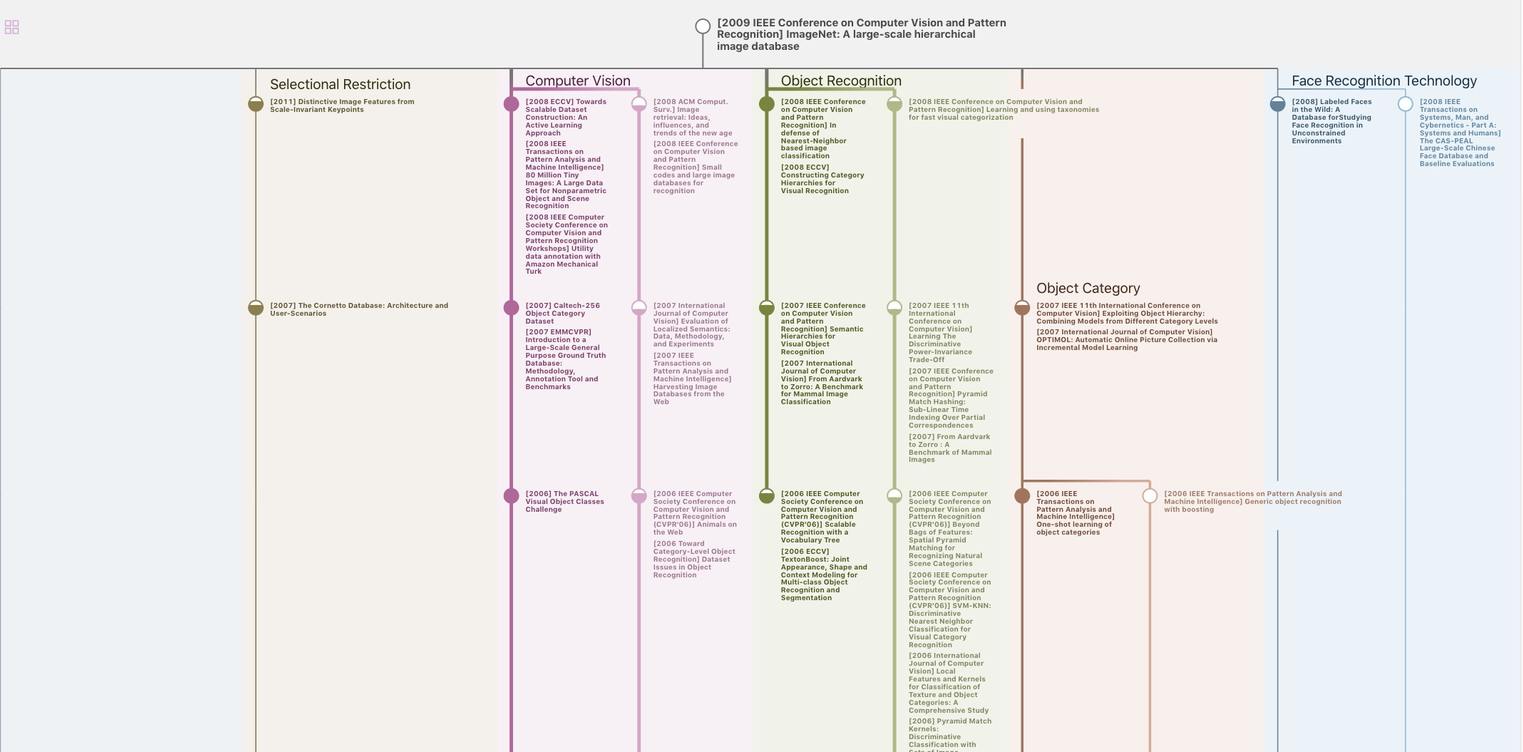
生成溯源树,研究论文发展脉络
Chat Paper
正在生成论文摘要