Does Human Collaboration Enhance the Accuracy of Identifying LLM-Generated Deepfake Texts?
arXiv (Cornell University)(2023)
摘要
Advances in Large Language Models (e.g., GPT-4, LLaMA) have improved the generation of coherent sentences resembling human writing on a large scale, resulting in the creation of so-called deepfake texts. However, this progress poses security and privacy concerns, necessitating effective solutions for distinguishing deepfake texts from human-written ones. Although prior works studied humans’ ability to detect deepfake texts, none has examined whether “collaboration” among humans improves the detection of deepfake texts. In this study, to address this gap of understanding on deepfake texts, we conducted experiments with two groups: (1) nonexpert individuals from the AMT platform and (2) writing experts from the Upwork platform. The results demonstrate that collaboration among humans can potentially improve the detection of deepfake texts for both groups, increasing detection accuracies by 6.36% for non-experts and 12.76% for experts, respectively, compared to individuals’ detection accuracies. We further analyze the explanations that humans used for detecting a piece of text as deepfake text, and find that the strongest indicator of deepfake texts is their lack of coherence and consistency. Our study provides useful insights for future tools and framework designs to facilitate the collaborative human detection of deepfake texts. The experiment datasets and AMT implementations are available at: https://github.com/huashen218/llm-deepfake-human-study.git
更多查看译文
关键词
human collaboration enhance,texts,llm-generated
AI 理解论文
溯源树
样例
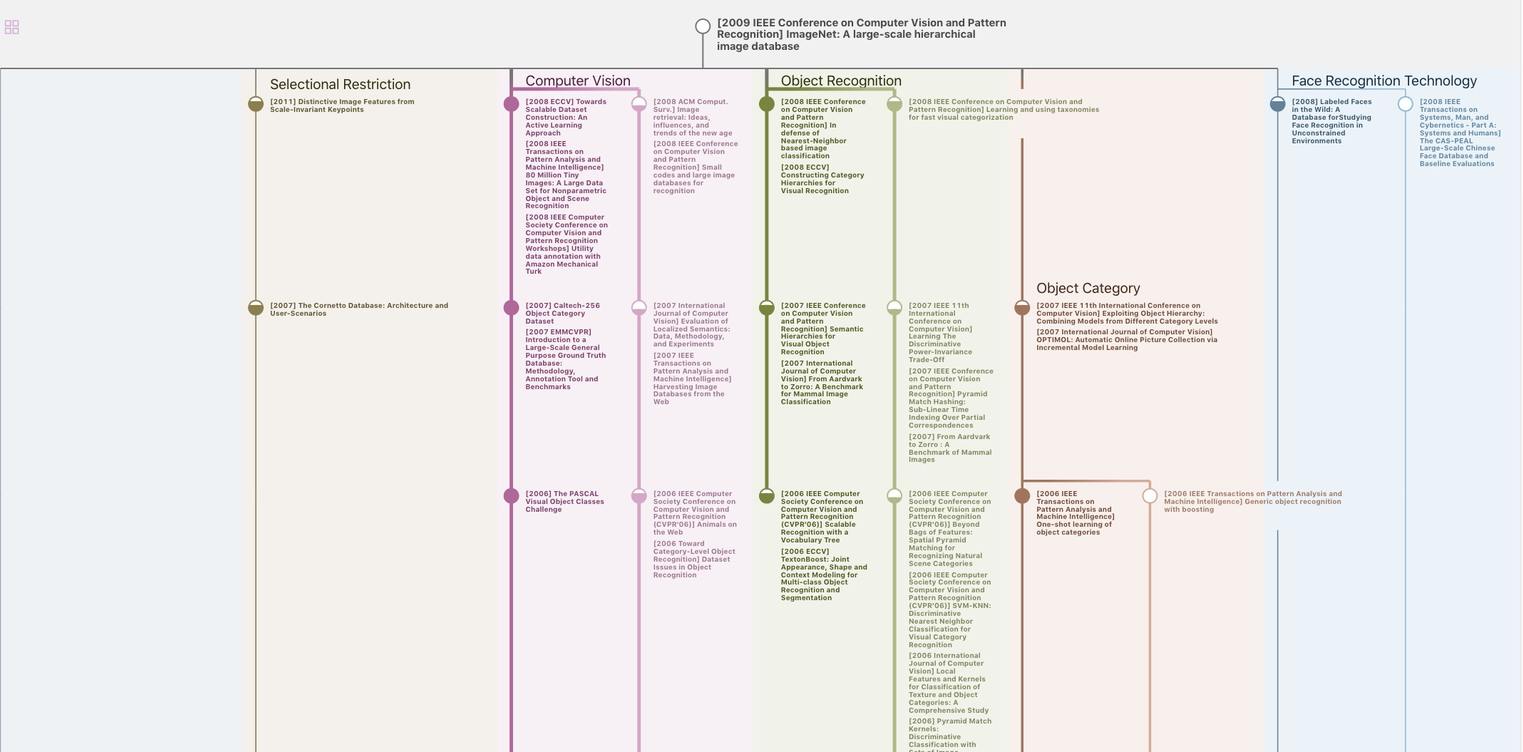
生成溯源树,研究论文发展脉络
Chat Paper
正在生成论文摘要