Multi-Descriptor Design of Ruthenium Catalysts for Durable Acidic Water Oxidation
Research Square (Research Square)(2023)
摘要
Abstract Further improvements in the performance and cost-effectiveness of water electrolyzers are urgently needed to accelerate decarbonization of hydrogen production. Iridium-free oxygen evolution reaction (OER) electrocatalysts are needed that are active and durable under acidic conditions. Here we report Ru 0.6 Cr 0.2 Ti 0.2 O 2 , identified from a machine-learning aided density functional theory (DFT) model using Pourbaix decomposition energy and metal-oxygen covalency as descriptors for electrochemical stability. To screen the entire space of bimetallic oxides for stability under harsh acidic conditions, we employ a graph convolution neural network to predict the Pourbaix decomposition energy accurately from unrelaxed structures. This was accomplished with an accuracy of 32 meV/atom. Notably, utilizing an optimized hyperbolic tangent activation function and dropout algorithm reduced the prediction error by 90%. Experimentally, the catalyst has an overpotential of 267 mV at 100 mA/cm 2 , accompanied by 200 hours of operation with an overpotential increase of less than 5 mV. DFT calculations show that adding Ti into the structure increases the metal-oxygen covalency of the system, improving the stability of the mixed-metal-oxide. At the same time, adding Cr lowers the energy barrier of the HOO* formation rate-determining step, thus improving activity compared to RuO 2 . We investigate structural and chemical changes during the reaction using in situ X-ray absorption spectroscopy and ptychography-scanning transmission X-ray microscopy. These evidence the evolution of a metastable structure compromised of a strong Ti-oxo network and a hydrous Cr-O passivation layer during the reaction – a structure that slows the dissolution of Ru by 20x while simultaneously suppressing lattice oxygen participation by > 60% compared to the case of RuO 2 .
更多查看译文
关键词
ruthenium catalysts,oxidation,multi-descriptor
AI 理解论文
溯源树
样例
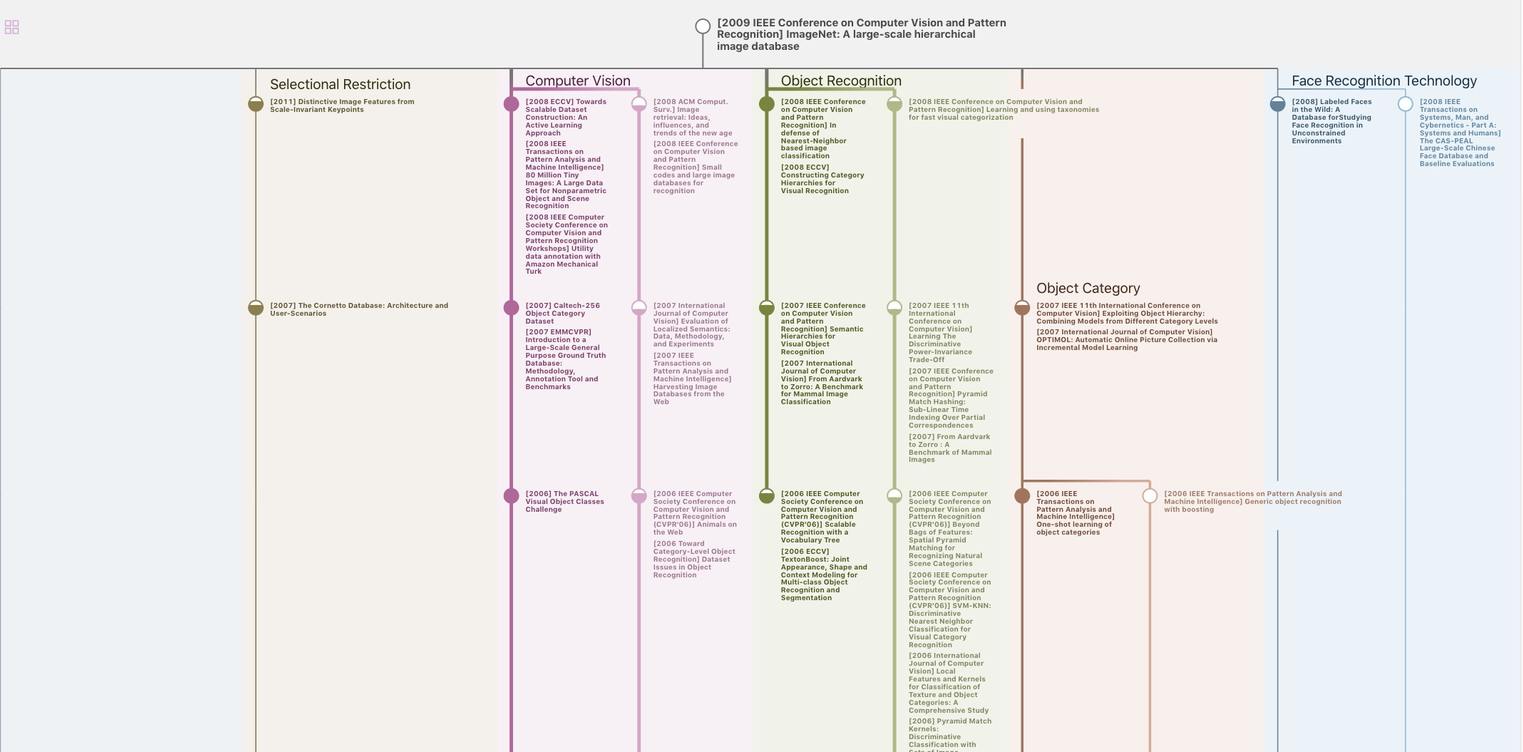
生成溯源树,研究论文发展脉络
Chat Paper
正在生成论文摘要