Optimal Transmission Scheduling over Multi-hop Networks: Structural Results and Reinforcement Learning
IEEE Transactions on Automatic Control(2023)
摘要
This paper studies the optimal transmission scheduling for remote state estimation over multi-hop networks. A smart sensor observes a dynamic system, and sends its local state estimate to a remote estimator (RE). To save energy, multi-hop networks are deployed to relay data packets from the smart sensor to the RE. The smart sensor needs to decide the hop number communicating with the RE by adjusting its transmission power. To minimize the estimation error and the energy consumption, the transmission scheduling is formulated as a modified Markov decision process (MDP) by incorporating historical actions into the state. A sufficient condition is constructed to guarantee that the MDP has an optimal deterministic and stationary policy. The optimal policy's structure is further obtained to reduce the computation complexity. A deep reinforcement learning (DRL) algorithm, i.e., dueling double Q-network (D3QN), is introduced to obtain a near-optimal policy. Finally, a simulation example is provided to illustrate the developed results.
更多查看译文
关键词
Sensor networks,Transmission scheduling,Estimation,Kalman filtering
AI 理解论文
溯源树
样例
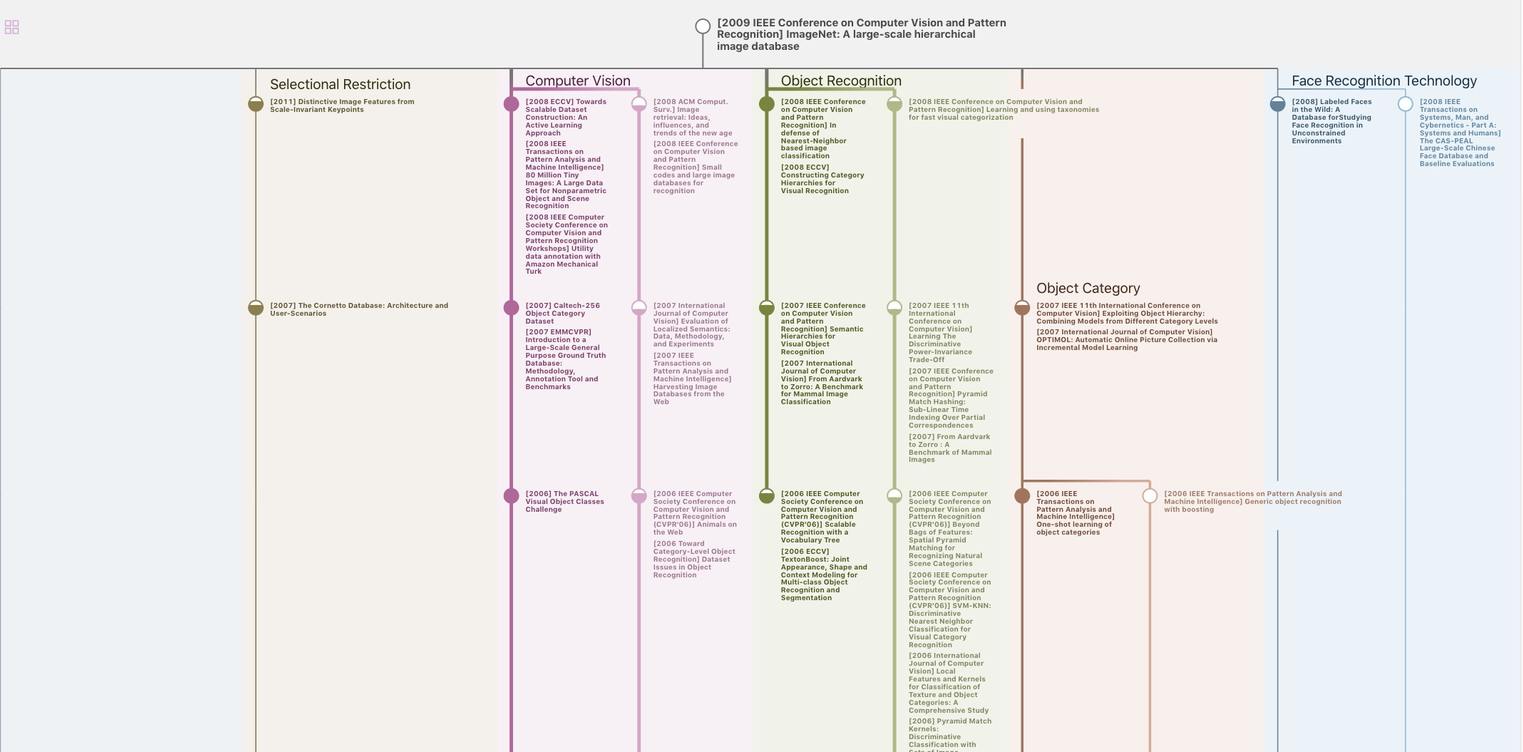
生成溯源树,研究论文发展脉络
Chat Paper
正在生成论文摘要