Distributed Bearing Fault Classification of Induction Motors Using 2-D Deep Learning Model
IEEE journal of emerging and selected topics in industrial electronics(2024)
摘要
Distributed bearing faults are highly common in industrial applications and display unpredictable vibration patterns impeding their detection. These faults stem from issues such as lubrication deficiencies, contamination, electrical erosion, roughness of the bearing surface, or the propagation of localized faults. This study aims to detect distributed bearing faults by utilizing a multisensory approach consisting of current, accelerometer, and fluxgate sensors. A novel 2-D deep learning framework is proposed, leveraging signals from six distinct sources, including three-axis vibration signals, stray magnetic flux signal, and two-phase current signals. Data are collected from 3- and 10-hp induction motors at 50 operational points, spanning ten speed levels and five torque levels. These six signals are transformed into matrices and combined to create a comprehensive matrix that provides an overall depiction of the bearing condition. The proposed deep learning architecture employs a 2-D convolutional model, which takes 2-D images as input and determines the bearing status. To evaluate the system's robustness, the data are divided into training and testing sets. The proposed model demonstrates remarkable effectiveness in detecting distributed bearing faults, achieving an impressive accuracy rate of 99.92%. Furthermore, a comprehensive comparison is provided, highlighting the impact of using various sets of inputs as sources for the deep learning model on the accuracy rate for each set. Through the analysis of the obtained results, a clear conclusion can be drawn: the model performs at its best when all six input sources are utilized.
更多查看译文
关键词
fault classification,induction motors,deep-learning deep-learning
AI 理解论文
溯源树
样例
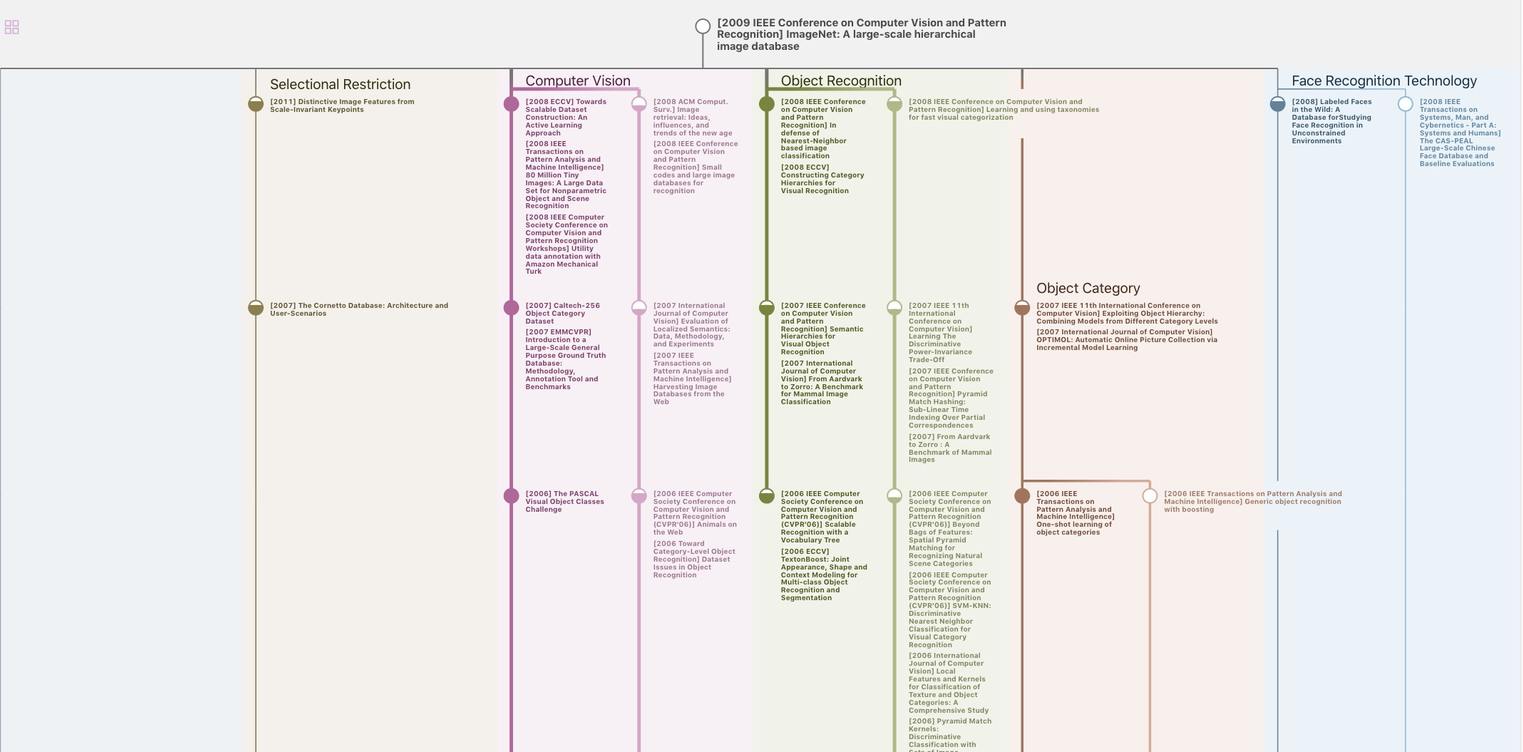
生成溯源树,研究论文发展脉络
Chat Paper
正在生成论文摘要