U-Shape Networks Are Unified Backbones for Human Action Understanding From Wi-Fi Signals.
IEEE Internet Things J.(2024)
摘要
Wi-Fi is well-known in communication and deployment for indoor localization. Recently, Wi-Fi has been exploited for human action understanding, e.g., elder fall detection, smoking detection, and hand-gesture recognition. Many researchers have made great efforts to associate their own expertise on Wi-Fi signals with deep networks like convolutional neural networks, recurrent neural networks, and Transformers for representation learning, and demonstrate that expertise can promote action understanding accuracy. However, expert knowledge always relies on the existing personal understanding and assumptions of Wi-Fi signals, which limits the scalability of associated models and may introduce subjective bias to the models. Besides, requiring expertise raises an inescapable barrier and cost to the model design. Recent years have witnessed the great value of backbone networks, such as ResNet, in advancing the research progress in computer vision. We believe a backbone network for Wi-Fi signals will also play a crucial role. In this paper, instead of proposing novel algorithms with expertise, we present that simple U-shape deep networks such as FCN, U-Net, and U-Net++ are efficient and unified backbones for Wi-Fi based human action understanding tasks, i.e., action recognition, action detection, and action segmentation. Results on three public datasets, WiAR, ARIL, and HTHI, show that all these U-shape deep networks have superior or competitive performance compared with original papers as well as state-of-the-art approaches, e.g., >97% recognition accuracy, <0.5-second detection errors, and >90% segmentation accuracy. We envision this work breaks the barrier of network design and facilitates human action understanding from Wi-Fi signals.
更多查看译文
关键词
Wi-Fi,backbone networks,U-Net,action understanding,deep learning
AI 理解论文
溯源树
样例
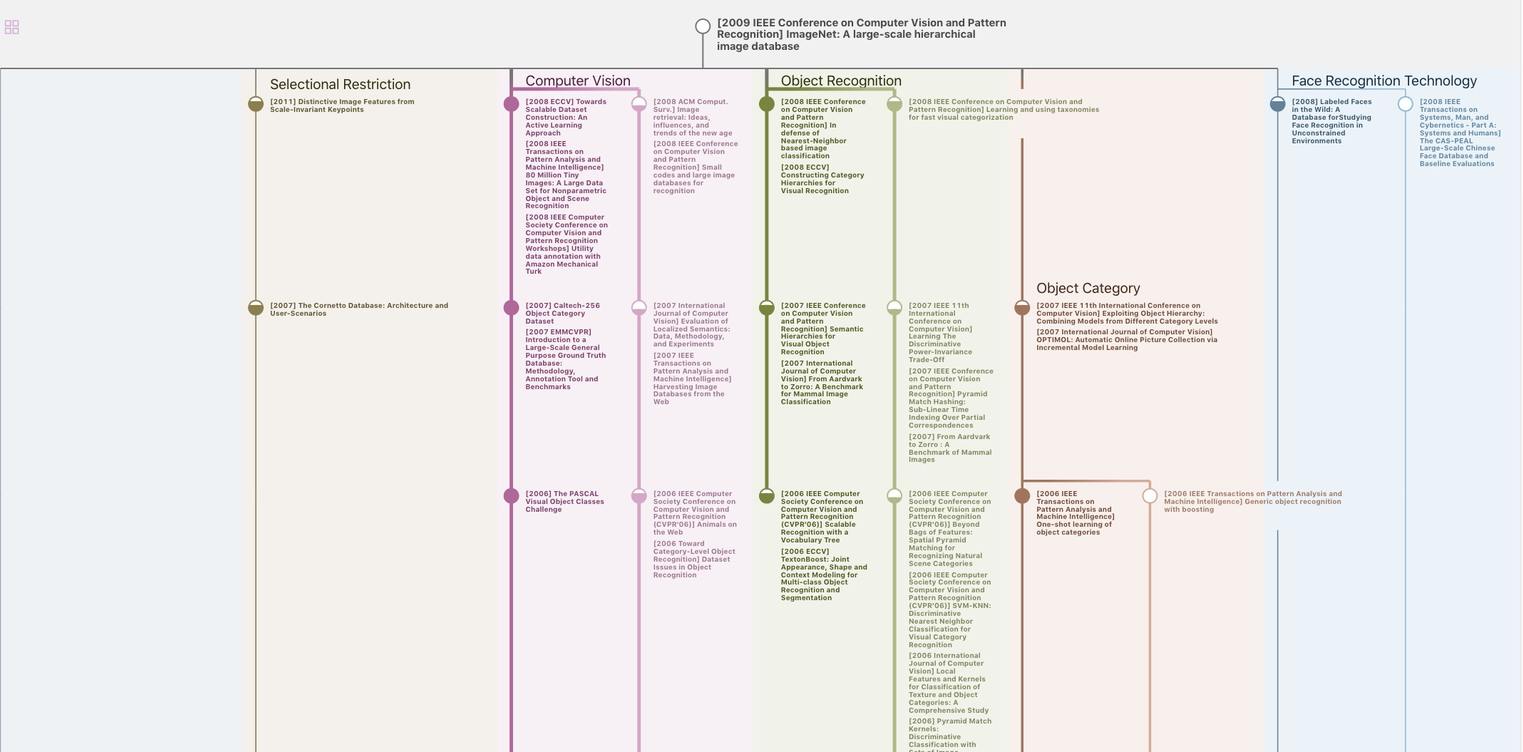
生成溯源树,研究论文发展脉络
Chat Paper
正在生成论文摘要