Accuracy Improvement of Knowledge Transfer from Private Training Data with PATE
Research Square (Research Square)(2023)
摘要
Abstract Some machine learning applications involve sensitive training data, such as the medical histories of patients in a clinical trial. A model may inadvertently and implicitly store some training data and careful analysis of the model may reveal the sensitive information. To address this problem, Papernot et al. proposed Private Aggregation of Teacher Ensembles (PATE) framework. PATE transfers the knowledge from the teacher model to the student model with teachers training on disjoint data and privacy guaranteed by noisy aggregation of teachers? predictions. However, PATE has a limit that it requires disjoint training data for a large number of teachers. If the number of teachers is small, then the student model has a poor accuracy. To address the issue of the PATE, we propose an efficient algorithm to improve the student accuracy when the number of teachers is small. In order to validate the performance of our algorithm, we conduct a series of experiments on MNIST and BreastCancer. The evaluation shows our algorithm improves on the original PATE with both high accuracy and strong privacy. For example, when the number of teachers n = 50 and the privacy budget = 3.76, the student of our algorithm achieves 92.1% accuracy while the original PATE only has 60.0%.
更多查看译文
关键词
private training data,knowledge transfer
AI 理解论文
溯源树
样例
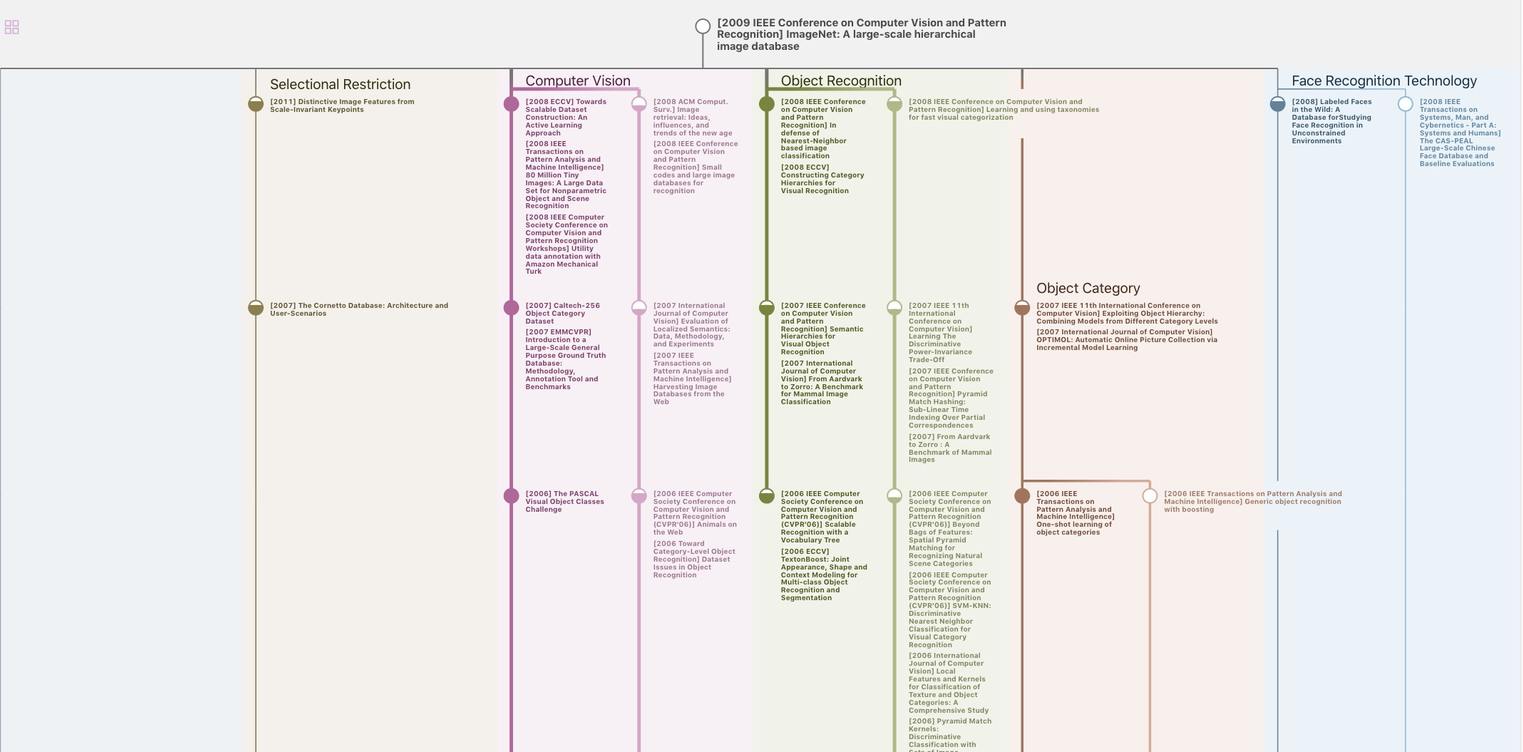
生成溯源树,研究论文发展脉络
Chat Paper
正在生成论文摘要