A Lightweight-Window-Portion-Based Multiple Imputation for Extreme Missing Gaps in IoT Systems.
IEEE Internet Things J.(2024)
摘要
Intelligent techniques, including artificial intelligence and deep learning, normally perform on complete data without missing data. Multiple imputation is indispensable for addressing missing data resulting in unbiased estimates and dealing with uncertainty by providing more valid results. Most state-of-the-art techniques focus on high missing rates (around 50%-60%) and short missing gaps, while imputation for extreme missing gaps and missing rates is an important challenge for multivariate time-series data generated through the Internet of Things (IoT). Hence, we propose a Lightweight Window Portion-based Multiple Imputation (LWPMI) based on multivariate variables, correlation, data fusion, regression, and multiple imputations. We conduct extensive experiments by generating extreme missing gaps and high missing rates ranging from 10% to 90% on data generated by sensors. We also investigate different sets of feature to examine how LWPMI works when features have high, weak, or a mixture of high and weak correlation. All the obtained results prove LWPMI outperforms baseline techniques in preserving pattern, structure, and trend in both 90% extreme missing gap and missing rates.
更多查看译文
关键词
LWPMI,Imputation in IoT,imputation in extreme missing gaps,missing data
AI 理解论文
溯源树
样例
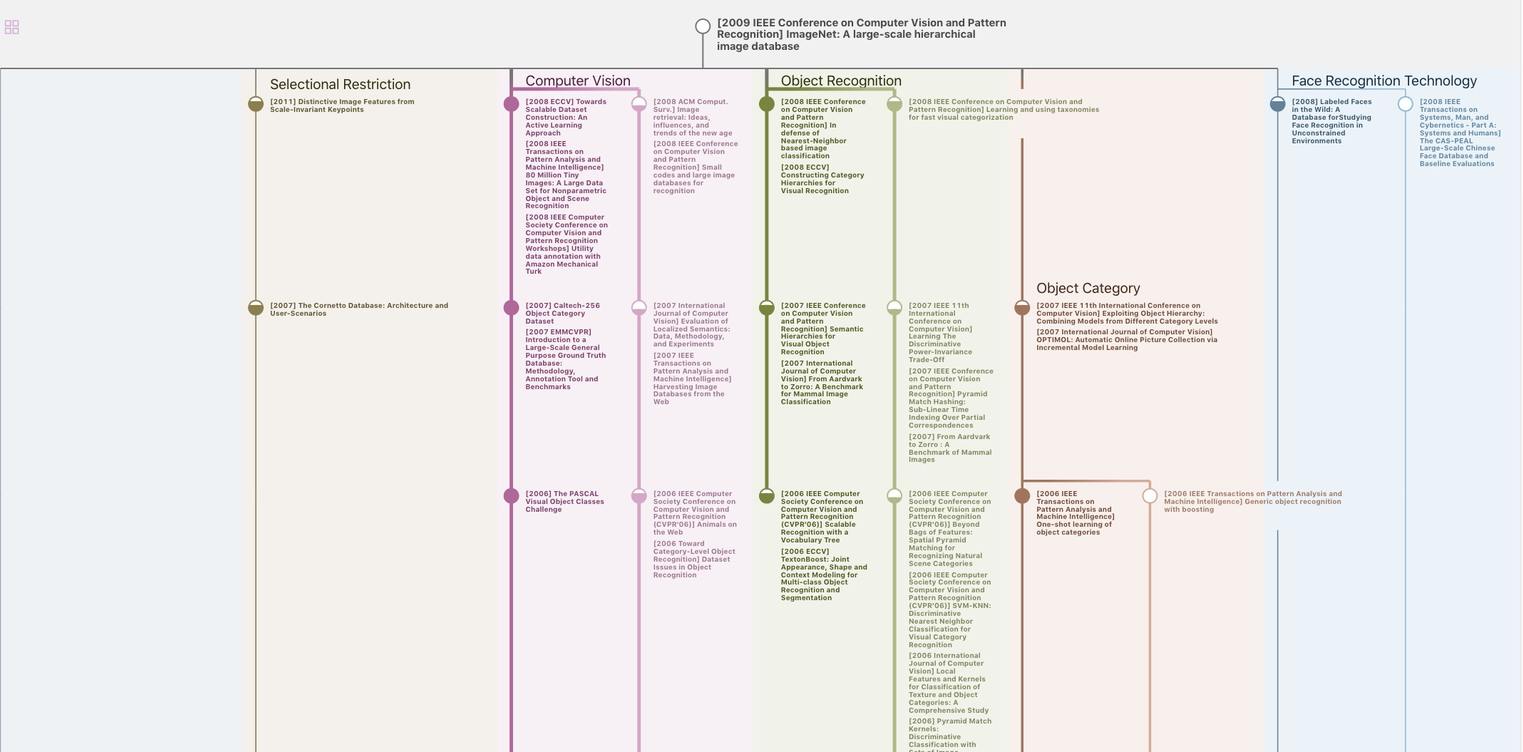
生成溯源树,研究论文发展脉络
Chat Paper
正在生成论文摘要