Is it time to re-think the appropriateness of autocorrelation for gait event detection? Preliminary results of an ongoing study
Gait & Posture(2023)
摘要
Recent developments in machine learning (ML)-based gait event detection have demonstrated superior results in terms of robustness and temporal accuracy compared to heuristic approaches [1–3]. “Autocorrelation” (AC) is an implemented heuristic algorithm in the Vicon Nexus application, which identifies events based on a recurring pattern of a certain marker. Clinicians often rely on the use of AC or other heuristic approaches to identify initial contact (IC) and foot off (FO) events. However, no literature exists on the accuracy of AC for event detection. We have recently developed IntellEvent [4], a ML-based event detection algorithm which has superior accuracy compared to current state-of-the-art methods [1,5]. We aim to evaluate its applicability in daily clinical use compared to the AC method. How large are the temporal differences of gait events detected by IntellEvent and the AC method? The retrospective dataset for this study comprises 3DGA data. Patients were classified having either malrotation deformities (MD, n=20) or infantile cerebral palsy (ICP, n=18). IntellEvent was used to detect all IC and FO events using the left and right velocity of the heel, ankle, and toe trajectories. For the AC method a threshold of 20 N was used to detect all IC and FO events on force plates. Subsequently, AC was used to detect all other events using the least square method of the x-axis trajectory (direction of motion). We validated IntellEvent by comparing its predictions to events solely identified with force plates. Afterwards, we calculated the differences between the remaining events of IntellEvent and the AC method. Mean absolute errors (95% confidence interval) of IntellEvent compared to the ground truth for IC (MD: 2.4ms (2.1-2.8), ICP: 3.7ms (3.2–4.1)) and FO (MD: 7.5ms (6.8–8.1), ICP: 10.5 (9.5–11.4)) events showed a high temporal accuracy for both pathologies (Fig. 1). The comparison between IntellEvent and the AC events shows greater deviations for IC (MD: 10.1ms (9.6–0.6), ICP: 11.5ms (10.9–12.1)) and FO (MD: 9.3ms (8.8–9.7), ICP: 15.4ms (14.6–16.2)). Fig. 1. Temporal errors between IntellEvent vs. force plate data (blue) and IntellEvent vs. Autocorrelation (orange). Dotted grey lines indicate an error of 6.67ms (= 1 frames) and dotted red lines an error of 26.66ms (= 4 frames).Download : Download high-res image (146KB)Download : Download full-size image IntellEvent achieves a very high temporal accuracy and robustness when compared to ground truth data. For the IC events, a high deviation between IntellEvent and AC was observed. Therefore, the results suggest that the AC can potentially introduce errors that may affect clinical decision making. Our preliminary results indicate that AC events need to be used with care when applied to pathological gait patterns and ML-based methods such as IntellEvent could improve the overall accuracy of gait event detection.
更多查看译文
关键词
gait event detection,autocorrelation,re-think
AI 理解论文
溯源树
样例
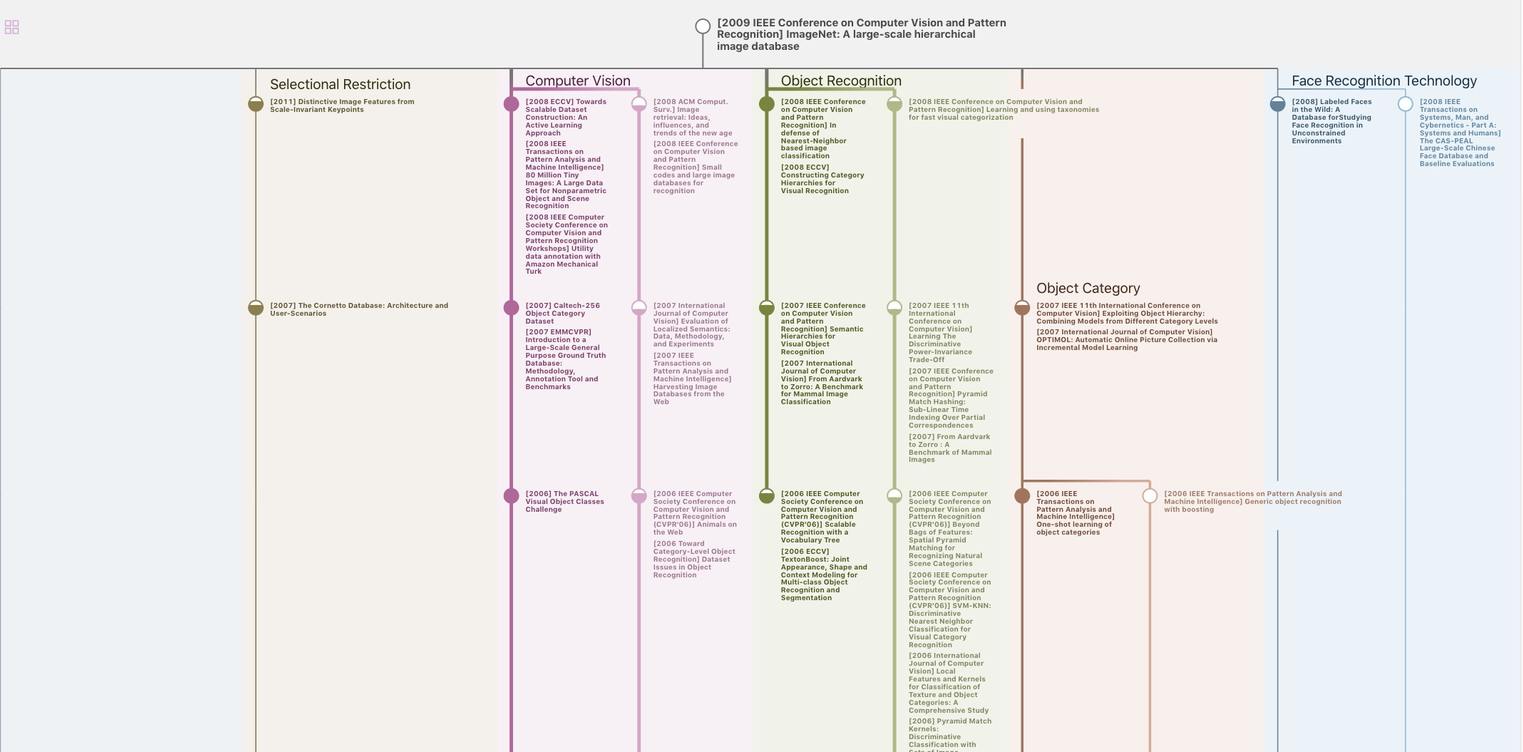
生成溯源树,研究论文发展脉络
Chat Paper
正在生成论文摘要