Sky-Image-Derived Deep Decomposition for Ultra-Short-Term Photovoltaic Power Forecasting
IEEE TRANSACTIONS ON SUSTAINABLE ENERGY(2024)
摘要
Because of the stochastic and nonstationary weather changes, photovoltaic (PV) power exhibits high uncertainty and fluctuation, which severely challenges the operation of power systems. PV variations can be detected by investigating inherent properties and reflections of sky images. However, limited study has focused on the effect of sky image changes on PV inherent properties. Therefore, in this study, a novel sky-image-derived decomposition method was proposed for ultra-short-term PV power forecasting. First, historical PV series were decomposed into seasonal and trend parts with guidance of sky image sequence. The Auto-Correlation mechanism was adopted to enhance the temporal dependencies of seasonal parts. Then, to reduce the model instability in different weather scenarios, the latent features were handled by an improved density-based spatial clustering of applications with noise using a semi-supervised strategy to identify forecasting scenarios. Finally, a rolling scenario-wise decoder was proposed to conduct multi-step forecasting task. The proposed method was compared with various state-of-the-art methods, including empirical mode decomposition and deep decomposition methods. The results validated the high performance of the proposed method in terms of accuracy within forecast horizons of 5 to 30 min.
更多查看译文
关键词
Deep decomposition,deep learning,ground-based sky images,photovoltaic power forecasting
AI 理解论文
溯源树
样例
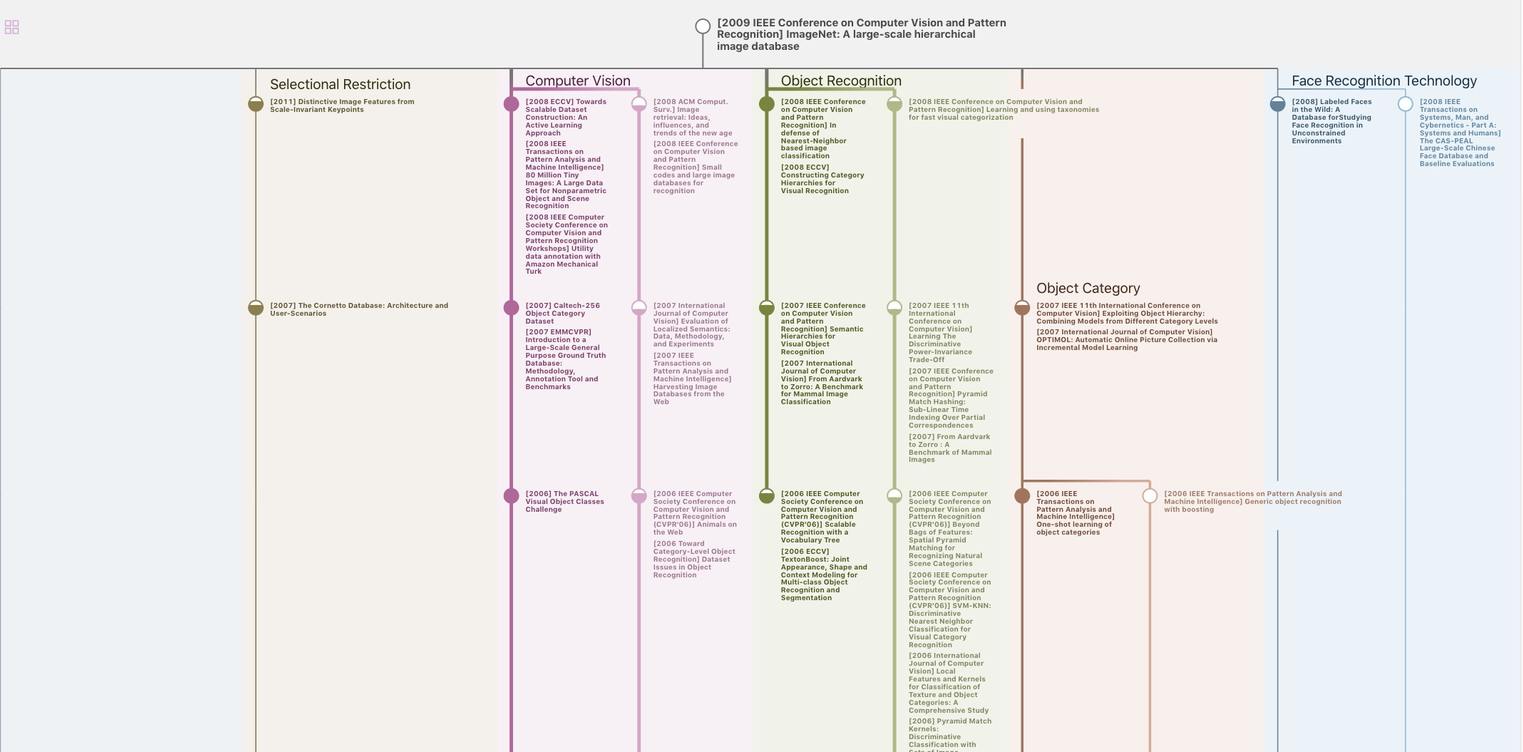
生成溯源树,研究论文发展脉络
Chat Paper
正在生成论文摘要